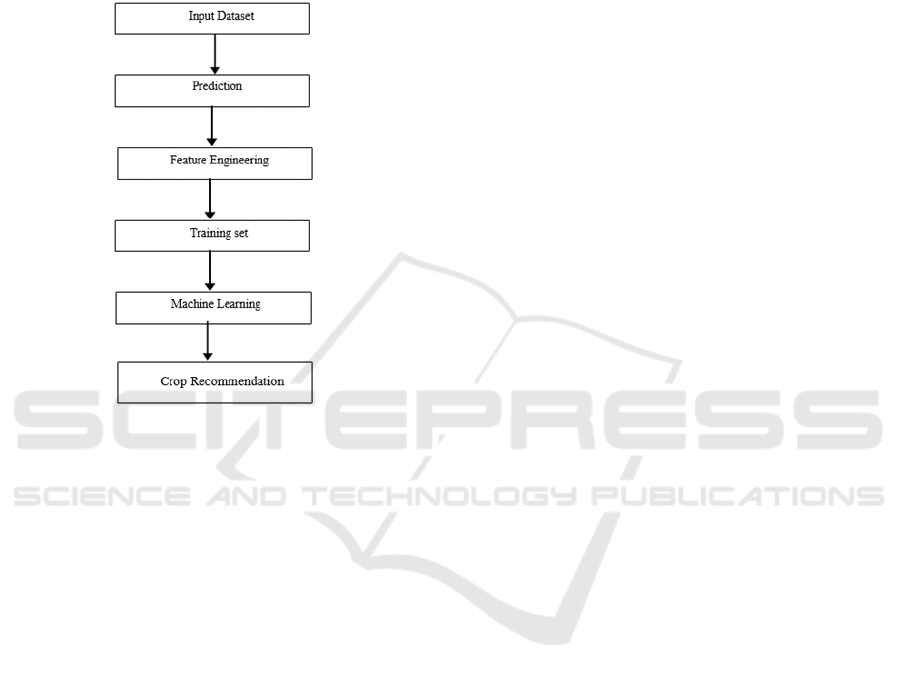
algorithms to agricultural data, such as Support
Vector Machine and Random Forest, and making
fertilizer recommendations that are suitable for each
unique crop. The research focuses on developing a
prediction model that could be applied to crop yield
forecasting in the future. It gives a succinct
description of how agricultural yield can be predicted
using machine learning methods.
Figure 1: Flow chart of Proposed Model.
Crop Recommendation on Analyzing Soil Using
Machine Learning
Influence on crop productivity. By implementing
emerging Agriculture into the effect, our
government's crops are stretched, which further
greatly boosts the economy of our nation. Crop
productivity has been strongly influenced by
fluctuations in the weather. Modern paradigm has the
potential to replace conventional farming with
precision farming, that will further improve
agricultural yields. Two examples of contemporary
technology in use are preliminary study and indeed
the internet of things (IOT). The fundamental issue
that remains to be investigated is growing precisely
crops at precise times (Anguraj.Ka et al. 2019). This
can be done using machine learning techniques,
which have been found to be an effective strategy for
predicting the perfect crop. Agriculture IOT sensors
are used to gather the soil data, such as soil moisture,
temperature, humidity, and pH, and provide them to a
graphical user interface (GUI). The GUI gathers the
inputs and recommends the appropriate crops. The
system created with IOT and ML significantly aids
farmers in making wise decisions.
Crop Recommendation Predictor Using Machine
Learning for Big Data
The needs of a constantly expanding human
population. In rural areas of India, agriculture is the
primary industry. We all know that the majority of
Indians work mostly in agriculture. Most Indians,
either explicitly or implicitly, depend on agriculture
for their livelihood. Most farmers in India rely on
their instincts to choose which crop to grow during a
certain season. Farmers are used to planting the same
crop, applying additional fertiliser, and adhering to
popular opinion. They are unaware of the fact that
crop productivity is heavily reliant on the current
weather, soil, and other factors and instead find
comfort in just adhering to previous agricultural
traditions and norms. The most frequent issue Indian
farmers have is that they don't choose the right crop
depending on the needs of their soil and other
elements like fertilisers and irrigation schedules
(Manish Kumar et al 2022. Nikam et al 2022).
Productivity suffers as a result. A single farmer,
however, cannot be expected to take into account all
of the various factors that affect crop development
when determining which crop to plant. Machine
learning is an effective solution to this issue.
Throughout the past few years, significant
improvements have been made in the ways that
machine learning can be used in numerous studies and
enterprises. As a result, we want to create a model or
system that will let farmers use machine learning in
agriculture.
3 PROBLEM DEFINITION
Few platforms exist that aid farmers in developing
their farming strategies. Decisions based on intuition
might not turn out to be advantageous in the long run.
Farmers frequently overestimate or underestimate the
soil fertility on their fields. They frequently have
trouble identifying plant illnesses that have an
immediate impact on the rate of output. It is feasible
to provide precise crop forecast results by using the
right criteria, such as rain patterns, temperature
patterns, soil structures, and other things like crop
diseases. Furthermore, it is also feasible to determine
in advance what disease a crop has. Several of the
current systems are exceedingly difficult to use or
have numerous problems that make them unintuitive.
AI4IoT 2023 - First International Conference on Artificial Intelligence for Internet of things (AI4IOT): Accelerating Innovation in Industry
and Consumer Electronics
282