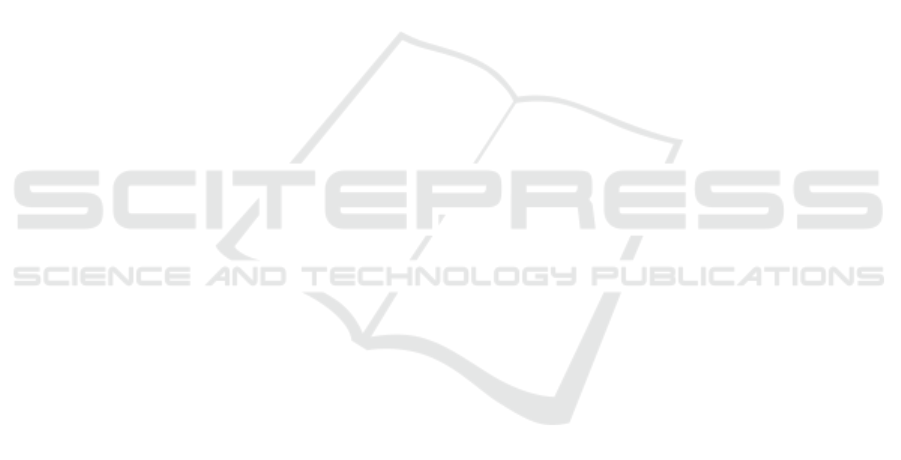
supervised, unsupervised, and reinforcement
learning. Reinforcement learning teaches computers
new tasks by showing them their real-world results.
Unsupervised machine learning uses cluster analysis
to analyze unlabeled data. Training supervised
machine learning requires labeled data. Every
labelled training data set has input values and a
predicted output value (Raghuvanshi et al, 2022). A
supervised learning method examines data to create
an inferred function for mapping unknown values.
Reinforcement learning is best for decision-making,
while unsupervised and supervised learning are best
for data analysis (Zamani et al, 2022).
The study seeks to determine crop yield factors.
In actuality, many factors determine harvest size.
Ecological and economic factors affect it.
Multivariate analysis is often used to investigate
many potential confounding factors (MVA). Linear
regression, factor analysis, canonical correlation, and
principal component analysis are used in agricultural
research to find hidden correlations.
Section 2 presents literature survey of various
techniques for crop yield prediction. Section 3
portrays methodology for accurate crop yield
prediction. Section 4 presents results and discussion.
Section 5 contains conclusion and future work.
2 LITERATURE SURVEY
Since massive amounts of data are now available in
many fields, natural resource management must use a
variety of computational methods and digital
technology. Data mining, big data analytics, cloud
computing, and ERP are used to study limits to better
long-term resource management. A literature
research can help you make sense of the mass of data
you have, analyze and interpret it using the best
models, and utilise it. New perspectives on the
obstacles and the project's potential impact are
revealed.
This article reviews and evaluates major
international, national, regional, and local research
and case studies on using digital technologies to
analyze crop yield parameters to better understand its
nature, magnitude, and complexity. This will help
understand the work.
Environmental factors like unexpected weather
disasters and economic considerations like market
demand and supply can affect crop productivity. In a
world with limited resources, crop productivity must
increase to fulfill population growth and food safety
needs. Thus, studying the variables that affect crop
yields and the models that best forecast them is
crucial. Grain storage and farm productivity
improvements would boost India's agricultural
production (Choudhary et al, 2020), benefiting its
domestic and international markets.
In 2011, wheat crops rose 6.4% to 85.9 million
metric tonnes. In 2011, global rice production rose
7% to 95.3% of the previous year's total (Veenadhari
et al, 2014). India was the seventh largest agricultural
exporter and sixth largest net exporter in 2013 with
$39 billion in agricultural exports. Agriculture,
forestry, and fisheries contributed 13.7% to GDP
(Patel et al, 2014).
Authors mapped the optimum agricultural
climates using transient, spatial, and spatiotemporal
data mining. Longitudinal data, big data quantity,
non-linear dependency, unexpected behavior, and
minimum and maximum threshold values were
potential hurdles. Reading about similar historical
situations may assist guide your future studies and
predictions (Ganguly et al, 2022).
Researchers provided a detailed ecological
systems and power plants caused environmental
shifts that inhibited infrastructure development.
Contemporary Earth System Models (ESM) were
performed at unacceptable spatial targets to examine
the effects in the limited area. Analysis was the goal.
Statistical downscaling can be utilized for regional
forecasts. Atmospheric senses are used for low- and
high-resolution mapping. Downscaled predictions
varied in accuracy and reliability based on observable
options. The climate system's spatial-temporal
characteristics necessitated image processing and
statistical downscaling. SRCNN technology for
downscaling climatic variables was described in this
paper. 20 ESM models were downscaled under
different emission scenarios (Sharma et al, 2022).
Researchers demonstrated a lightweight
computing platform that simulates and visualizes
massive data sets. An ICT platform gives researchers
a "simple-to-coordinate" general tool for remote data
administration. Scalable cloud computing and
industry-standard web technologies that work across
a variety of clients and platforms underpin the
architecture. Specifically, before and after
representation, 3D data set compression and
interpretation, combined virtual conditions, and
CAD/E application analysis needed improvement. In
chemical process design, the CAD/E stage produced
from academic applications and contextual research.
Computer-aided design/engineering is CAD/E
(Kumar et al, 2022).
Data-driven firms like "Natural Resource
Management" (NRM) profit tremendously from
government programs that provide precise ecological
AI4IoT 2023 - First International Conference on Artificial Intelligence for Internet of things (AI4IOT): Accelerating Innovation in Industry
and Consumer Electronics
542