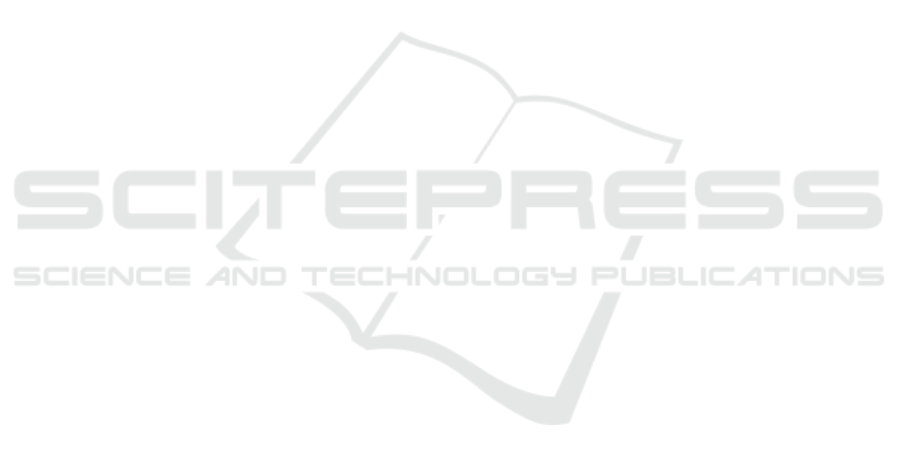
context, time, and sequencing are crucial. Educational
data mining can predict student performance, identify
at-risk students, determine important concerns in the
learning patterns of various student groups, increase
pass-out rates, accurately assess the institution's
performance, maximize campus resources, and
optimize subject curriculum renewal (Hicham et al,
2020).
A thorough quality management system helps
universities make necessary changes to combat
process entropy and provides critical feedback for
continuous progress. This ensures high-quality
university services. Keeping note of and investigating
the myriad ideas and emotions that arise throughout
teaching and learning is crucial. These feed-forward
mechanisms control deviations and introduce
appropriate interventions at the right times to ensure
smooth and effective teaching and learning growth.
Make-up classes, bridge courses, and extra
homework may be needed to maintain and improve
university education (Kovalev et al, 2020).
Learning and teaching aim to give students the
best experience possible in learning and
understanding the topic. Data and information for
each activity, such as teaching and learning, is crucial
to comprehensive quality management in higher
education. Empirical evidence should support TQM
rather than subjective assessments. Intelligent data
analysis, classification, and prediction offered by
machine learning may improve this issue (Khodeir et
al, 2019).
This article uses machine learning to analyze
student comments on higher education quality
management. Preprocessed student feedback data
removes noise. SVM, ANN, and random Forest
algorithms analyze student feedback. SVM algorithm
performs better for assessing student feedback data to
improve higher education quality.
2 LITERATURE SURVEY
The best decision tree algorithm, SVM, C4.5, Naive
Bayesian, and RIPPER prediction algorithms were
compared (Eswara et al, 2017). When FP rate,
Precision, F-M, Recall, and MCC are compared,
Naive Bayes wins. It is unclear if or how these
algorithms can improve college instruction.
Researcher employed decision tree categorization
on student evaluation results to improve teaching and
learning quality. They sought to identify students at
risk of poor performance. The technique fails to
identify student strengths and weaknesses, creating a
knowledge gap (Mesaric et al, 2016). The feedback
will be excellent or bad based on how well the person
can identify the lesson or understanding that needs
improvement. A study on the reliability of student
feedback ratings or quantitative characteristics used
linguistic qualities of the accompanying free text in
feature space (Kannan et al, 2011). A stronger
awareness for textual evidence leads to higher marks.
The quantitative ratings and qualitative remarks
regarding the feature are compared. Naive Bayes was
used to classify Gujarati texts into a few main groups
(Rakholia et al, 2017). The classifier performs better
on randomly partitioned 10 times test data than 2
times. This shows that the classifier may have had
insufficient training data for the latter instance. Since
feature selection enhances prediction accuracy, not
employing a classifier is better. Additionally, it works
on small data sets. K-Nearest Neighbor (K-NN) and
Naive Bayes were tested for movie and hotel
evaluation accuracy, precision, and recall. The Naive
Bayes approach outperformed the K-NN method in
movie rating prediction, but both systems performed
similarly in hotel rating prediction.
3 METHODOLOGY
This section provides machine learning techniques
for analysing students feedback towards quality
management in higher education. Student feedback
data set is preprocessed to remove noise. Then student
feedback data is analysed using SVM, ANN and
random Forest algorithm. An excellent example of
supervised learning is the SVM classifier, which
processes enormous volumes of data in order to
recognize patterns that were not previously visible.
The categorization and analysis of multivariate data
are two examples of common uses. The support
vector machine (SVM) classifier assigns a single
label to newly collected data. This classifier is built
on the basis of the probabilistic binary classifier.
Nevertheless, in spite of the fact that it belongs to the
non-linear category, it is sometimes referred to as the
kernel. Support vector machines, often known as
SVMs, are frequently hailed as the most efficient
margin classifiers. This is generally due to the fact
that SVMs are able to efficiently split n-numbers of
records that belong to the same category. It is better
to have a non-linear relationship between the margin
value and each of the categories. The SVM idea is
used rather often when it comes to the process of data
classification Arifin et al, 2021).
ANNs are not a novel concept by any means.
Because of the interdependence of its input and
output data, this system may replicate a sophisticated
AI4IoT 2023 - First International Conference on Artificial Intelligence for Internet of things (AI4IOT): Accelerating Innovation in Industry
and Consumer Electronics
310