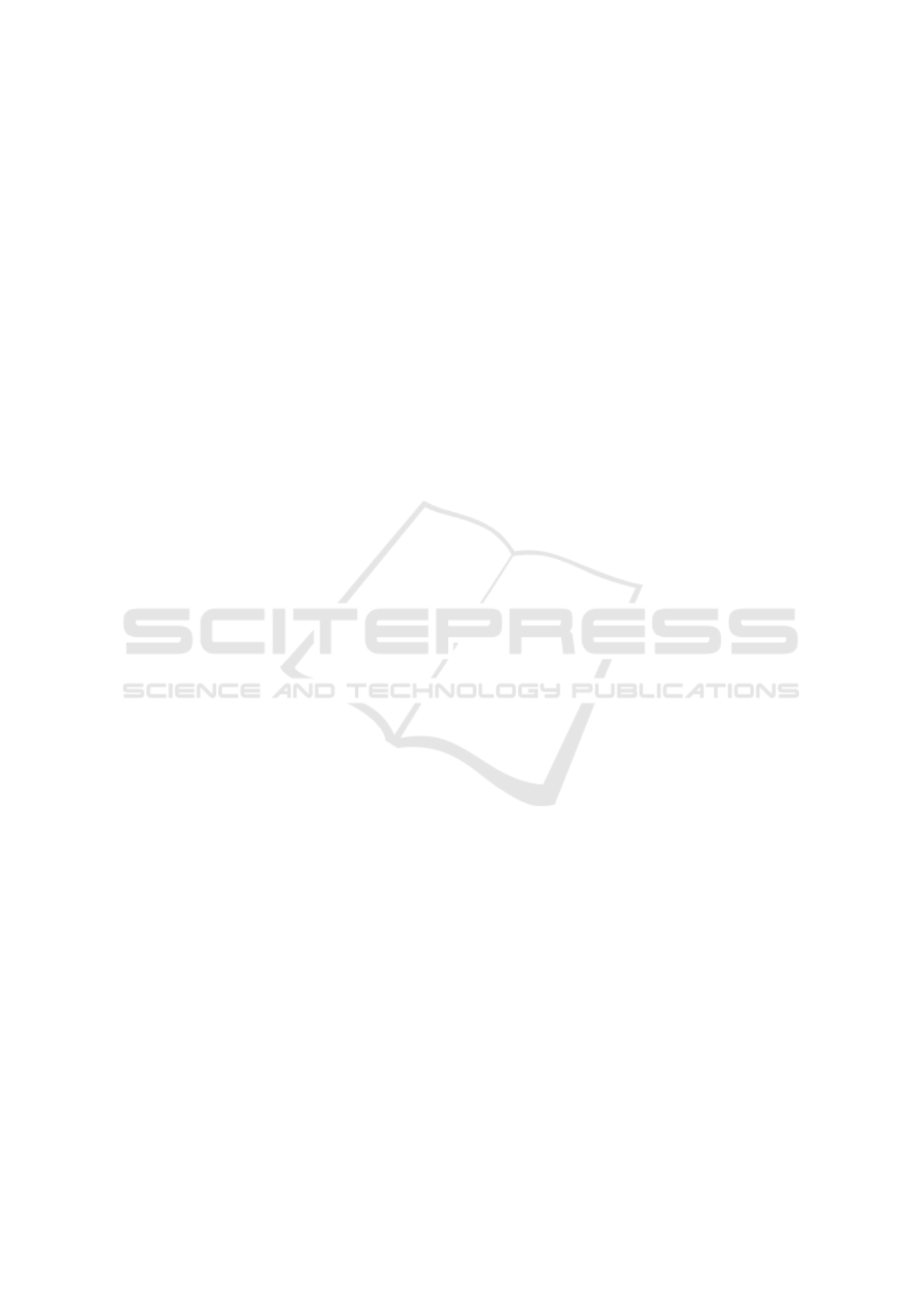
GDP. For the record, the empirical finding is robust
with the alternative method such as DOLS.
REFERENCES
Adaramola, O. A., & Dada, O. (2020). Impact of inflation
on economic growth: Evidence from Nigeria.
Investment Management and Financial Innovations,
17(2), 1–13.
https://doi.org/10.21511/imfi.17(2).2020.01
Africa, S. (2020). Causality E ff ects among Gross Capital
Formation , Unemployment and Economic Growth in
South Africa.
Agboola, M. O., Bekun, F. V., Osundina, O. A., &
Kirikkaleli, D. (2022). Revisiting the economic growth
and agriculture nexus in Nigeria: Evidence from
asymmetric cointegration and frequency domain
causality approaches. Journal of Public Affairs, 22(1).
https://doi.org/10.1002/pa.2271
Alharthi, M., & Hanif, I. (2020). Impact of blue economy
factors on economic growth in the SAARC countries.
Maritime Business Review, 5(3), 253–269.
https://doi.org/10.1108/MABR-01-2020-0006
Ardhani, I. (2021). Indonesia and The Criminalization of
Illegal, Unregulated, and Unreported Fishing in The
Global Level. Jurnal Hubungan Internasional, 9(2),
165–177. https://doi.org/10.18196/jhi.v9i2.10374
Asian Development Bank. (2014). State of the Coral
Triangle: Indonesia.
Ceccarelli, D. M., Lestari, A. P., Rudyanto, & White, A. T.
(2022). Emerging marine protected areas of eastern
Indonesia: Coral reef trends and priorities for
management. Marine Policy, 141, 105091.
https://doi.org/https://doi.org/10.1016/j.marpol.2022.1
05091
Eyüboğlu, S., & Akmermer, B. (2023). The Relationship
between Economic Growth and Fisheries Production in
Türkiye. Aquaculture Studies, 24(2).
https://doi.org/10.4194/AQUAST1017
Guan, J., Kirikkaleli, D., Bibi, A., & Zhang, W. (2020).
Natural resources rents nexus with financial
development in the presence of globalization: Is the
“resource curse” exist or myth? Resources Policy,
66(January 2020), 101641.
https://doi.org/10.1016/j.resourpol.2020.101641
Hadi, S. N., & Chung, R. H. (2022). Estimation of Demand
for Beef Imports in Indonesia: An Autoregressive
Distributed Lag (ARDL) Approach. Agriculture, 12(8),
1212. https://doi.org/10.3390/agriculture12081212
Hassan, S. T., Xia, E., Latif, K., Huang, J., & Ali, N. (2020).
Another Look at the Nexus Among Energy
Consumption, Natural Resources, and Gross Capital
Formation: Evidence from Pakistan. Natural Resources
Research, 29(4), 2801–2812.
https://doi.org/10.1007/s11053-019-09607-0
Ilyas, F., Gillani, D. Q., Yasin, M., Iqbal, M. A., Javed, I.,
Ahmad, S., & Nabi, I. (2021). Impact of Livestock and
Fisheries on Economic Growth: An Empirical Analysis
from Pakistan. Sarhad Journal of Agriculture, 38(1),
160–169.
https://doi.org/10.17582/journal.sja/2022/38.1.160.169
Jaunky, V. C. (2011). Fish exports and economic growth:
The case of SIDS. Coastal Management, 39(4), 377–
395. https://doi.org/10.1080/08920753.2011.589210
Kent, G. (1997). Fisheries, food security, and the poor.
Food Policy, 22(5), 393–404.
https://doi.org/https://doi.org/10.1016/S0306-
9192(97)00030-4
Kurohman, F., Wijayanto, D., & Jayanto, B. B. (2020). The
strategy of capture fisheries development in Pamekasan
regency, Madura Island. AACL Bioflux, 13(5), 2816–
2827.
Liu, Y., Amin, A., Rasool, S. F., & Zaman, Q. U. (2020).
The role of agriculture and foreign remittances in
mitigating rural poverty: Empirical evidence from
Pakistan. Risk Management and Healthcare Policy, 13,
13–26. https://doi.org/10.2147/RMHP.S235580
McClanahan, T., Allison, E. H., & Cinner, J. E. (2015).
Managing fisheries for human and food security. Fish
and Fisheries, 16(1), 78–103.
https://doi.org/https://doi.org/10.1111/faf.12045
Moore, P. D. (2013). Impact of inflation on economic
growth: A Case Study of Tanzania. Asian Journal of
Empirical Research, 3(4), 363–380.
https://doi.org/10.1038/253011b0
Nathaniel, S. P., & Bekun, F. V. (2020). Environmental
management amidst energy use, urbanization, trade
openness, and deforestation: The Nigerian experience.
Journal of Public Affairs, 20(2).
https://doi.org/10.1002/pa.2037
Oktavilia, S., Firmansyah, Sugiyanto, F. X., & Rachman,
M. A. (2019). Competitiveness of Indonesian fishery
commodities. IOP Conference Series: Earth and
Environmental Science, 246(1).
https://doi.org/10.1088/1755-1315/246/1/012006
Oyakhilomen, O., & Zibah, R. G. (2013). Fishery
production and economic growth in Nigeria: Pathway
for sustainable economic development. Journal of
Sustainable Development in Africa, 15(2), 99–109.
Peristiwady, T. (2021). Ichthyological Research and
Biodiversity of Marine Fishes in Indonesia. IOP
Conference Series: Earth and Environmental Science,
789(1). https://doi.org/10.1088/1755-
1315/789/1/012009
Pesaran, M. H., Shin, Y., & Smith, R. J. (2001). Bounds
testing approaches to the analysis of level relationships.
Journal of Applied Econometrics, 16(3), 289–326.
https://doi.org/10.1002/jae.616
Rehman, A., Deyuan, Z., Hena, S., & Chandio, A. A.
(2019). Do fisheries and aquaculture production have
dominant roles within the economic growth of
Pakistan? A long-run and short-run investigation.
British Food Journal, 121(8), 1926–1935.
https://doi.org/10.1108/BFJ-01-2019-0005
Ridzuan, N. H. A. M., Marwan, N. F., Khalid, N., Ali, M.
H., & Tseng, M. L. (2020). Effects of agriculture,
renewable energy, and economic growth on carbon
dioxide emissions: Evidence of the environmental
Does the Blue Economy Resource of Capture Fisheries Generate Economic Growth? Evidence from Indonesia
23