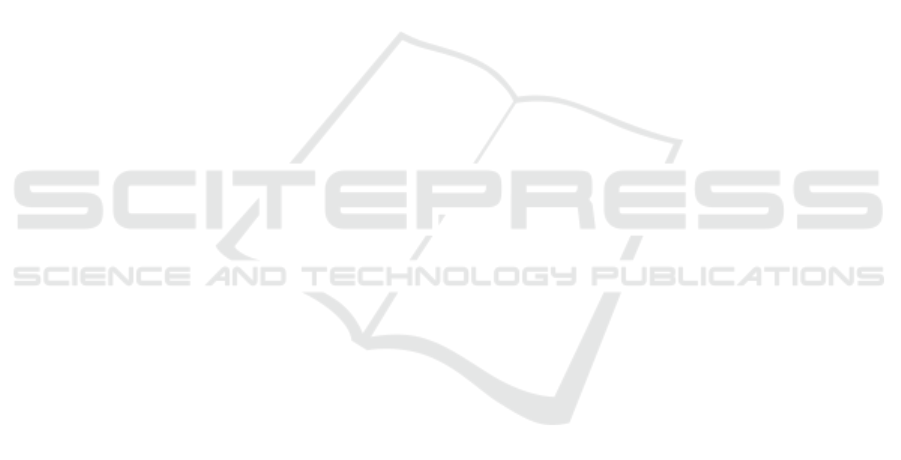
evaluating histological features, morphological
patterns, and biologically relevant regions. This
surpasses manual assessments, saving time and
reducing human errors associated with tasks like
counting fatigue (Barisoni, L., et al., 2020), Neltner,
J. H. et al., 2012).
3.3 Artificial Intelligence and Machine
Learning
The increasing demand for data reproducibility and
the complexity of pathology analyses have driven the
integration of AI (Artificial Intelligence). AI,
employing algorithms, extracts information beyond
human visual perception (Aeffner, F. et al., 2019).
It rapidly analyzes extensive data, expediting the
discovery of novel histopathology features. In breast
cancer, unsupervised learning models differentiate
between tumor grades and assess prognostically
relevant morphological features. Deep learning,
applied to IHC- and H&E-stained specimens, impacts
various tumor types, including prostate cancer
grading, biomarker identification in melanoma,
breast cancer region detection, prediction of response
to therapy, and recurrence prediction in lung cancer.
It enhances interpretability through graph neural
networks, providing comprehensive tissue
representations (Aeffner, F. et al., 2019)-
Vamathevan, J. et al., 2019).
To assess AI's utility, comparisons with
pathologists are crucial. In classifying melanoma, a
CNN outperformed histopathologists. In detecting
breast cancer metastases, deep learning matched top
pathologists and excelled in finding micrometastases.
Machine learning aids tumor purity assessment,
surpassing visual estimates in accuracy. Combining
WSI with machine learning offers insights in
translational research, but requires vast, high-quality
data. Challenges arise from variability in histological
data across labs, impacting algorithm development.
Robust AI algorithms could mitigate inter-reader
variability, but further research is needed to
understand their impact fully (Tizhoosh, H. R. &
Pantanowitz, L., 2018).
3.3.1 Stratification of Patients
Digital pathology aids in predicting treatment
response and identifying responsive patients. Spatial
analysis, utilizing machine learning models, extracts
morphological details to distinguish responders to
therapies like nivolumab in NSCLC. Digital image
analysis quantifies CD8 and PD-L1 positive cell
densities, revealing potential predictive composite
biomarkers. AI and machine learning assist in tumor
classification and staging, with deep learning models
predicting patient survival more accurately than
traditional pathology practices (Wang X. et al., 2018),
(Barrera C. et al., 2018).
3.3.2 Applications in Diagnosis
Biomarker research in immune-oncology explores
predictive values in solid tumors, particularly the role
of PD-L1 expression in response to immune
checkpoint inhibitors (ICIs). Challenges in accurate
patient stratification using visual interpretation lead
to a shift towards digital scoring, aiding pathologists
with standardized metrics. Studies reveal AI and
digital techniques outperform or equal manual
assessments across tumor types. AI's role extends to
morphological assessments in nonalcoholic liver
diseases. FDA-approved platforms support
quantitative image analysis, showcasing potential in
molecular epidemiology studies and expanding
beyond HER2 detection to classify ER- and PR-
positive breast cancer subtypes (Langer, C. J. et al.,
2016).
3.3.3 Applications in Clinical Practice
Several resources provide guidelines for
pathologists and physicians in digital pathology,
covering image handling, WSI device standards,
validation, AI concepts, and reimbursement
considerations. The College of American
Pathologists offers comprehensive guidelines for
WSI system validation, emphasizing emulation,
sample size, concordance, and documentation. AI
performance relies on dataset size and quality,
requiring diverse, well-curated images for training
and validation, with pathologists playing a crucial
role in ensuring accuracy and relevance to clinical
applications (Pantanowitz, L. et al., 2014),
(Janowczyk, A. & Madabhushi, A., 2016).
4 COPYRIGHT FORM
The authors ensure that the publisher has the
authorization to publish the Contribution.
5 CONCLUSIONS
Digital pathology advancements provide practical
benefits, enhancing accuracy and enabling remote
image review. In immuno-oncology, AI and machine
IeHF 2023 - International e-Health Forum
6