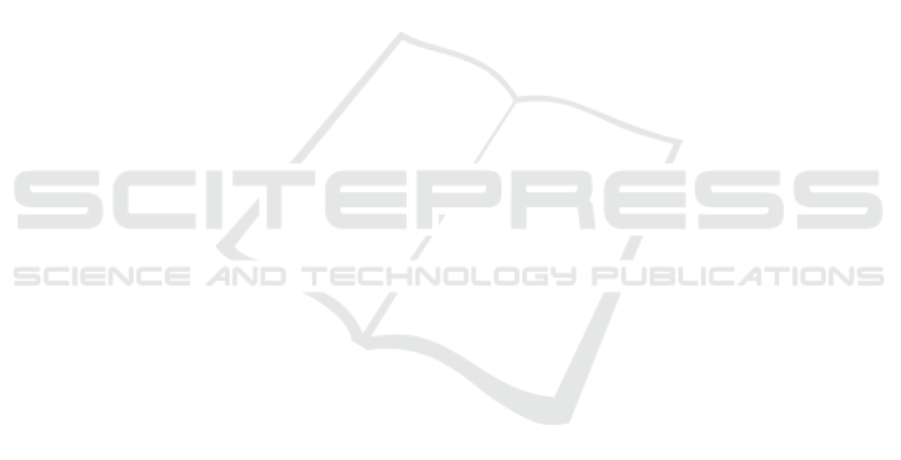
covers a wide range of statistical techniques for
recognising visual patterns, such as support vector
machines, logistic regression, and linear regression
(Ripley, 1986). The approach for using linear
regression to do image processing tasks, such as
denoising, deblurring, and super-resolution, is
presented in this study. Using a linear regression
classifier (Wieland and Pittore, 2014). This study
proposes a method for classifying facial expressions
based on a collection of characteristics collected from
a face picture using linear regression. In order to
increase performance, this work suggests a linear
regression-based method for classifying images that
makes use of a low-rank representation of the picture
attributes (Hester and Casasent, 1980).
Low capacity to recognise intricate patterns:
Linear regression is a straightforward approach that
frequently fails to identify intricate nonlinear
correlations between picture characteristics and class
labels (Chen and Peter Ho, 2008).
Research paper stated that performance may thus
be constrained in tasks that call for the understanding
of intricate picture patterns. Lack of resilience to
picture data fluctuations. Linear regression models
are frequently vulnerable to image data alterations,
such as shifts in size, rotation, or viewpoint. Because
of this, they may perform poorly in situations when
the picture data varies widely. The proposed work
aim is to increase the computational time efficiently
so that it can quickly classify the images and make
predictions.
2 MATERIALS AND METHODS
The work was carried in AI lab of the CSE
department in SSE of the Saveetha Institute of
Medical and Technical Sciences. The novel Linear
Regression Method was used in Group 1 and KNN
was used in Group 2. The sample size was calculated
based on earlier studies using clinicalc.com (Roy,
2018) withGpower as 80%, the confidence interval at
95%, and the threshold for the computation was set at
0.05 with a significance level of p=0.001. The dataset
for this particular research topic was retrieved from
the Kaggle repository. 25% of the database is set
aside for testing, while 75% is reserved for training.
Ten data samples total are gathered, divided into two
groups.
2.1 Linear Regression
Linear regression is one of the most fundamental and
popular Machine Learning methods. It is a method for
carrying out predictive analysis that is based on
statistics. Linear regression generates forecasts for
continuous/real/numeric variables like sales, salary,
age, and product price, among others. a dependent
variable and one or more independent variables (y)
are shown to be linearly related by the linear
regression method, also known as linear regression. It
is possible to use linear regression to determine how
the value of the dependent variable changes as a
function of the value of the independent variable
because it exhibits a linear relationship. The two types
of machine learning linear regression methods are as
follows: Simple linear regression is a sort of linear
regression technique that uses just one independent
variable to predict the value of a numerical dependent
variable. When more than one independent variable
is used to predict the value of a numerical dependent
variable, multiple linear regression is the technique
used.
Algorithm 1.
Input: Image pattern recognition_ Input Features
Output: Classification of Image pattern recognition
Step 1: Gather labeled images with corresponding
input features A and output values B.
Step 2: Partition data in training plus testing.
Step 3: Normalize the input features A and output
values B.
Step 4: Implement Linear Regression algorithm to
build a model.
Step 5: Performance is evaluated on training set.
Step 6: Adjust hyperparameters or consider using
other algorithms if performance is unsatisfactory.
Step 7: Use the model to predict output values B for
new images based on input features A.
2.2 Enhanced K-Nearest Neighbor
It is a simple algorithm in which each pixel is
allocated to the class in the training set with the
highest intensity. A NN algorithm may determine
something incorrectly if the acquired single neighbor
is an outlier of a different class. In order to get around
this and improve the method's resilience, the KNN
Algorithm employs K patterns. The KNN Algorithm
is characterized as a non parametric Machine learning
Algorithm since it does not rely on any underlying
assumptions about the statistical make-up of the data.
Algorithm 2.
Input: Image pattern recognition_ Input Features
Output: Classification of Image pattern recognition
Step-1: Gather labeled images with corresponding
input features A and B.
AI4IoT 2023 - First International Conference on Artificial Intelligence for Internet of things (AI4IOT): Accelerating Innovation in Industry
and Consumer Electronics
374