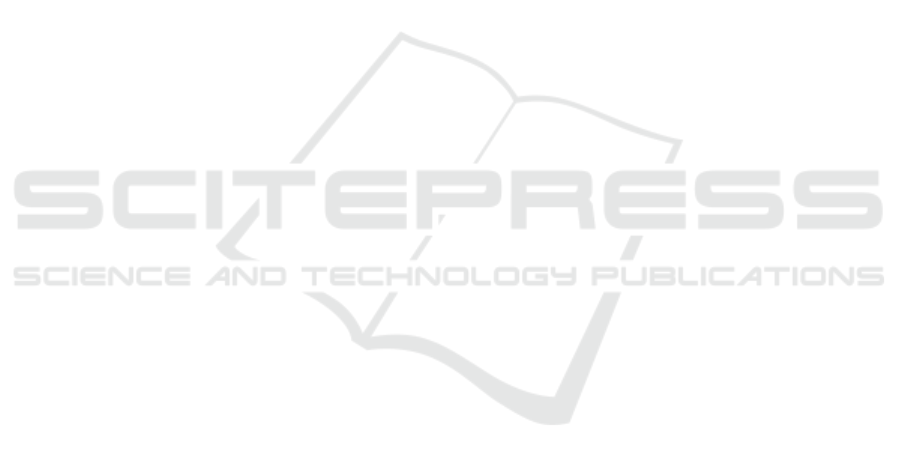
attributes and churn scores were illuminated through
the lens of linear regression analysis.
The findings reveal that subscription tenure,
internet service type, monthly charges, and contract
duration all contribute to the intricate tapestry of
customer churn. Longer tenure and extended contracts
were found to correlate with lower churn scores,
underscoring their role in fostering loyalty.
Conversely, certain internet service types and higher
monthly charges were associated with elevated churn
scores, highlighting the need for service quality and
pricing considerations.
However, this study is not without its limitations.
The linear regression model used in this study, while
effective, may oversimplify the complex relationships
between various factors contributing to customer
churn. Additionally, some factors like Payment
Method, Tech Support, or Online Security that might
affect the results are not considered in the research.
This may lead to an error in the study.
Future research could explore more sophisticated
predictive models or machine learning algorithms that
can capture non-linear relationships and interactions
between variables. Moreover, comparative studies
involving multiple service providers across different
geographical locations could provide more
comprehensive insights into customer churn patterns.
The linear regression model's adeptness in
predicting churn scores, coupled with the insights
derived, equips telecom providers with actionable
intelligence for crafting targeted retention strategies.
By leveraging this predictive tool, providers can
mitigate churn risks and bolster customer loyalty,
thereby navigating the dynamic telecommunications
landscape with acumen. This study serves as a
steppingstone towards more advanced predictive
models and broader comparative studies in the future.
REFERENCES
F. Reichheld, R. G. Markey Jr, and C. Hopton, “The loyalty
effect-the relationship between loyalty and profits”.
European business journal, vol. 12, no. 3, pp. 134, 2000.
Y. Duan, and Z. W. Ras, “Recommendation system for
improving churn rate based on action rules and
sentiment mining”. International Journal of Data
Mining, Modelling and Management, vol. 14, no. 4, pp.
287-308, 2022.
A. Payne, and P. Frow, “Customer relationship
management: Strategy and implementation”. In The
Marketing Book. Routledge, pp. 439-466, 2016.
PricewaterhouseCoopers. “Experience is everything:
Here’s how to get it right”. 2018.
H. S. Kim, and N. Kwon, “The advantage of network size
in acquiring new subscribers: a conditional logit
analysis of the Korean mobile telephony market”.
Information economics and policy, vol 15, no. 1, pp. 17-
33, 2003.
J. Lee, and L Feick, “The impact of switching costs on the
customer satisfaction ‐ loyalty link: mobile phone
service in France”. Journal of services marketing, vol.
15, no. 1, pp. 35-48. 2001.
J. H. Ahn, S. P. Han, and Y. S. Lee, “Customer churn
analysis: Churn determinants and mediation effects of
partial defection in the Korean mobile
telecommunications service industry”.
Telecommunications policy, vol. 30, no. 10-11, pp.
552-568, 2006.
A. Amin, S. Anwar, A. Adnan, M. Nawaz, K. Alawfi, A.
Hussain and K. Huang, “Customer churn prediction in
the telecommunication sector using a rough set
approach”. Neurocomputing, vol. 237, pp. 242-254,
2017.
L. M. Davis, and K. N. Lemon, “The wow factor: Creating
value through win-back offers to reacquire lost
customers”. Journal of Retailing, vol. 83, no. 1, pp. 47-
64, 2007.
S. Nasır, “Customer retention strategies and customer
loyalty”. In Advertising and Branding: Concepts,
Methodologies, Tools, and Applications. pp. 1178-
1201. 2017.
S. K. Parahoo, J. M. Aurifeille, and S. K. Sobhee,
“Contractual loyalty: leveraging partnerships to achieve
customer loyalty in global markets”. Globalization and
Partnerships: Features of Business Alliances and
International Cooperation, 2007.
DAML 2023 - International Conference on Data Analysis and Machine Learning
514