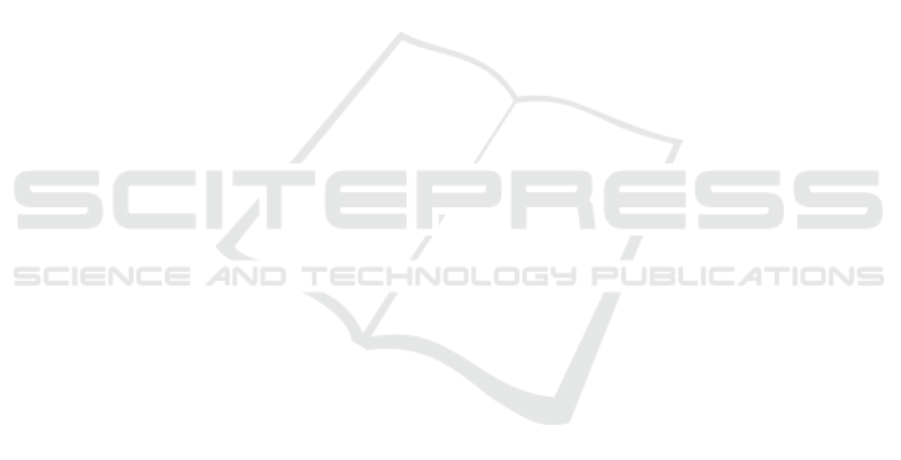
higher than that of the second model, and it exhibits
greater fluctuations. Similarly, the generator loss for
the first model shows more significant fluctuations and
has a substantially higher absolute value compared to
the second model. This means that the training dataset
for the second model can be more conducive to training
the model. Using PSNR index to measure the SR
results of the two models, the first model is slightly
lower than the second model. This demonstrates that
the ratio between the SR result and the original image
is greater, indicating an improved quality of the
generated image. This means that training on a single
animal dataset and using the generated model to SR
process images of the corresponding species will be
slightly better. In summary, the results suggest that it
is better to use single-species datasets to train models
and process images of that species.
4 CONCLUSION
The purpose of this study is to compare the SR effects
of models trained on different training datasets on the
same target dataset. Specifically, it is investigated
whether the models trained on the training dataset of
the same kind of animals have better SR effect on the
target dataset of the same kind of animals. In this
experiment, the SR model based on ESRGAN is
established and a dataset consisting of pictures of
various animals and a dataset consisting only of
pictures of dogs are trained separately. By comparing
the change of the loss value of the discriminator and
the generator during the training process, this
experiment can judge the training effect of the model
and the training time required. Finally, SR processing
is performed on the same target dataset composed of
dog pictures and the SR effects are compared. The
results show that the SR effect of the models trained
with different training sets is not the same when the SR
processing of a certain kind of animal image is needed.
The model trained with the same type of animal images
as the target dataset can have a better SR effect on the
target animal images. At the same time, images
consisting of a single species lead to faster training of
models with less loss. In the future, this experiment
will also consider removing the background of the
dataset to obtain better experimental results, use more
kinds of training dataset to compare the experimental
effects and consider using more metrics to measure the
SR effect of the images.
REFERENCES
A. Sharma, B. P. Shrivastava, “Different Techniques of
Image SR Using Deep Learning: A Review,” IEEE
Sensors Journal, vol. 23, 2022, pp. 1724-1733.
M. Frid-Adar, I. Diamant, E. Klang, et al. “GAN-based
synthetic medical image augmentation for increased
CNN performance in liver lesion classification,”
Neurocomputing, vol. 321, 2018, pp. 321-331.
Q. Yang, Y. Ma, F. Chen, et al. “Recent advances in photo-
activated sulfate radical-advanced oxidation process
(SR-AOP) for refractory organic pollutants removal in
water,” Chemical Engineering Journal, vol. 378, 2019,
pp. 122149.
K. Schawinski, C. Zhang, H. Zhang, et al. “Generative
adversarial networks recover features in astrophysical
images of galaxies beyond the deconvolution limit,”
Monthly Notices of the Royal Astronomical Society:
Letters, vol. 467, 2017, pp. 110-114.
R. Keys, “Cubic convolution interpolation for digital image
processing,” IEEE transactions on acoustics, speech,
and signal processing, vol. 29, 1981, pp. 1153-1160.
A. Sharma, B.P. Shrivastava, “Different Techniques of
Image SR Using Deep Learning: A Review,” IEEE
Sensors Journal, vol. 23, 2022, pp. 1724-1733.
M.N Favorskaya, A.I. Pakhirka, “SF-SRGAN: Progessive
GAN-based Face Hallucination,” The International
Archives of the Photogrammetry, Remote Sensing and
Spatial Information Sciences, vol. 48, 2023, pp. 47-52.
X. Wang, K. Yu, S. Wu, et al. “Esrgan: Enhanced super-
resolution generative adversarial networks,”
Proceedings of the European conference on computer
vision (ECCV) workshops, 2018.
J. Yang, J. Wright, T. Huang, et al. “Image super-resolution
as sparse representation of raw image patches,” 2008
IEEE conference on computer vision and pattern
recognition. IEEE, 2008, pp. 1-8.
Dataset
https://www.kaggle.com/datasets/iamsouravbanerjee/a
nimal-image-dataset-90-different-animals
J. Jiang, L. Zhao, Y. Jiao, “Research on Image Super-
resolution Reconstruction Based on Deep Learning,”
International Journal of Advanced Network, Monitoring
and Controls, vol. 7, 2022, pp. 1-21.
Super-Resolution Analysis of Animal Images Based on ESRGAN Model
437