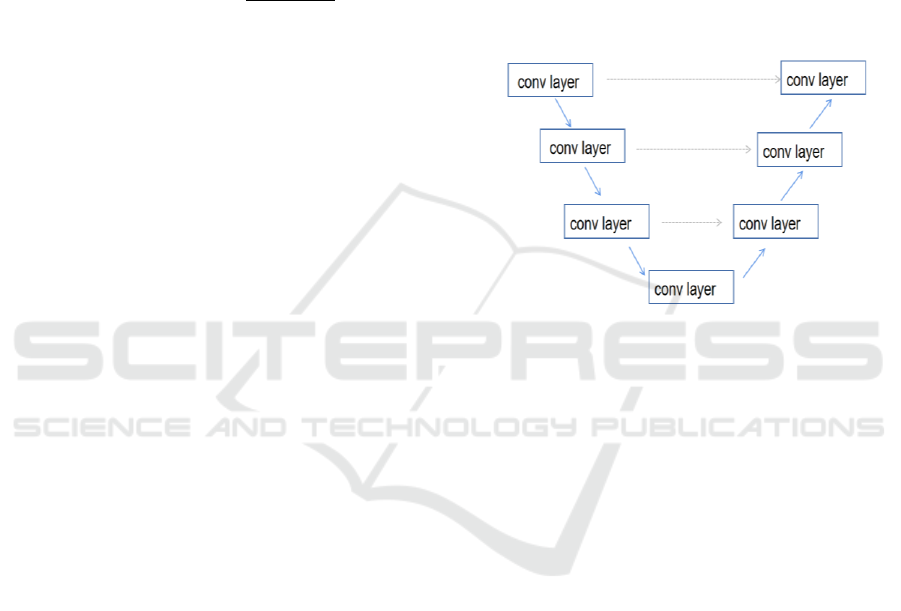
generator, making the generated images closer to the
target images. During training, L1 Loss serves as an
important feedback signal, assisting the generator in
gradually producing more realistic images.
2.2.2 L2 Loss
L2 Loss, also known as Mean Squared Error, is
indeed a loss function commonly used for regression
problems. The mathematical expression for L2 Loss
is as follows:
(2)
The advantage of L2 loss is that it is a smooth,
continuously differentiable function, which makes it
easy to handle in optimization algorithms like
gradient descent. Additionally, it is typically a convex
function, implying it has a global minimum.
However, L2 loss calculates errors using squared
terms, which means it is more sensitive to large errors
because squaring amplifies the impact of these errors.
2.2.3 GAN Loss
Adversarial Loss as one of the primary
implementations of Generator Loss, is typically
represented in the specific form of Binary Cross-
Entropy Loss. It pushes the model's training by
having the generator and discriminator engage in
mutual competition. The generator aims to create
more realistic data, while the discriminator strives to
differentiate between real and generated data. This
adversarial training approach leads to continuous
improvement in the generator's ability to produce
more authentic data. However, GAN loss training is
often less stable compared to traditional supervised
learning because the competition between the
generator and discriminator can result in oscillations
during the training process. Therefore, it's crucial to
carefully select hyperparameters and employ various
techniques to stabilize the training. Additionally,
during the experiments, we also attempted to replace
L1 Loss with L2 Loss and observed the training
results.
2.2.4 Unet
The generator in the pix2pix model uses a U-net
network architecture. It is a deep learning
convolutional neural network architecture consisting
of an encoder and a decoder. The first half is used for
feature extraction, while the second half is used for
upsampling. Its specific network architecture is
shown in Figure 3. In this architecture, the down-
sampling path consists of convolutional layers and
pooling layers, which are used to reduce image
resolution, decrease the spatial size of the image, and
simultaneously extract image features. In contrast, the
up-sampling path serves the opposite purpose and has
a complementary architecture compared to the down-
sampling path. U-Net also employs skip connections
to connect feature maps of different depths between
the encoder and decoder. This helps in transmitting
both low-level and high-level features, addressing the
common issue of information loss. The U-net
network's five pooling layers enable it to achieve
multi-scale feature recognition in images, making it
highly effective for semantic image segmentation.
Figure 3: Unet architecture (Original).
2.3 Implementation Details
In this experiment, the choice of using the Adam
optimizer is justified. Adam has relatively low
demands on storage and computational resources,
making it advantageous for deep neural networks
dealing with large-scale data and parameters.
Additionally, Adam's ability to adaptively adjust the
learning rate can accelerate the model's training
process. Simultaneously, we trained each model for a
total of 20 epochs to ensure that we could observe
variations in training results among different models
and also assess differences in training efficiency. In
the selection of hyperparameters, the experiments
conduct four different ratios of GAN Loss to L1 Loss,
namely 1:100, 1:200, 1:10, and 1:1, and observe the
impact of GAN Loss and L1 Loss on the training
results under these various ratio combinations.
3 RESULTS AND DISCUSSION
In the results section, the paper showcases and
discussed the image translation outcomes under
various loss functions. Keeping the training dataset
consistent with the pix2pix dataset and maintaining all
DAML 2023 - International Conference on Data Analysis and Machine Learning
440