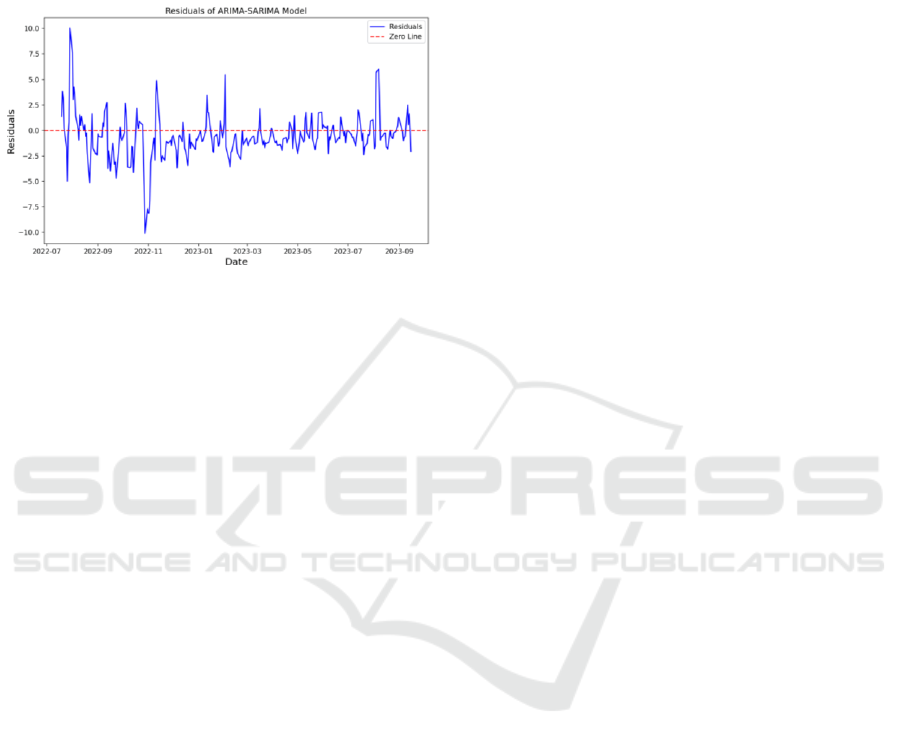
3.3 Evaluation
Fig. 9 displays the residual plot of the hybrid model,
demonstrating properties such as zero mean and
randomness. Fig. 9 depicts the residuals obtained
from the predictions for AMZN stock.
Figure 9: Residuals of ARIMA-SARIMA Model. (Picture
credit: Original).
The Mean Squared Error (MSE) of the hybrid
model obtained through rolling forecasting and cross-
validation is consistently less than 2.21. The author
conducted numerous model training for the AMZN
stock and calculated the average MSE from each
iteration. The average MSE of the hybrid model is
2.1914293478806233.
Under otherwise identical conditions, the average
MSE for predictions using a single ARIMA model i
s 2.579234434378756, and for a single SARIMA mo
del, it is 2.3328237024728707.
4 DISCUSSION
Numerous models have been developed in the
financial literature to predict return and volatility, but
the ARIMA model is the most widely used, which was
published by Arnerić & Poklepović in 2016 (Arnerić
et al 2014). Nevertheless, the ARIMA-SARIMA
hybrid model outperforms single ARIMA or
SARIMA models in terms of predictive capability.
This indicates that in stock prediction, there is a
combination of seasonal and non-seasonal factors.
However, the author reckons that hybridizing ARIMA
and SARIMA models might be a somewhat
adventurous endeavor. The hybrid model in this paper
precisely complements the seasonal factors that
ARIMA lacks with SARIMA and compensates for the
non-seasonal factors that SARIMA overlooks with
ARIMA. Through the assumed research and empirical
simulation analysis of the aforementioned quantitative
environment, the hybrid model proposed in the paper
yielded relatively favorable results in specific trading
contexts. This suggests that employing tools for
quantitative trading offers certain advantages.
Quantitative trading, due to its reliance on the rapid
and robust computational capabilities of computers,
holds an absolute advantage in market breadth
analysis (Li and Xia 2023). However, the factors
influencing stock price trends in the actual market are
intricate and diverse. Therefore, if various political
and economic factors affecting the stock market are
considered alongside technical indicators as input
variables, better results may be obtained (Agrawal et
al 2013).
5 CONCLUSION
The stock price prediction model has been effectively
deployed using the aforementioned techniques, with
feature engineering emerging as a crucial step in
enhancing prediction accuracy for this regression
problem. In an effort to enhance stock price prediction
accuracy, this study aspires to introduce a fresh
perspective. Nonetheless, given the multitude of
factors influencing stock prices, the current model's
performance may not be entirely satisfactory. To
enhance the model's effec-tiveness, future research
should consider incorporating a wider array of factors.
REFERENCES
S. Ren, X. Wang, X. Zhou, Y. Zhou, “A novel hybrid model
for stock price forecasting integrating Encoder Forest
and Informer,” Expert Systems with Applications,
Volume 234, 2023, 121080, ISSN 0957-4174,
https://doi.org/10.1016/j.eswa.2023.121080.
F.X. Deng, “Application of LSTM Neural Network in Stoc
k Price Trend Prediction,” Master's thesis, Guangdong
University of Foreign Studies, 2019, https://kns.cnki.n
et/kcms2/article/abstract?v=InnWydrwIfKm2Z35Pntd
CsfBawT604FUNihTlaJLVNqnhwfaWN2kRbqki1Rd
MbUheTK2Jlwt62CDDuzq1y4XMGZfX6rsdojNBX4
W5a9KbSVOrrjLI6JZT74wL9yytntgCzw4WYEVzXo
=&uniplatform=NZKPT&language=CHS.
H. Herwartz, “Stock return prediction under GARCH — An
empirical assessment,” International Journal of
Forecasting, vol. 33, pp. 569-580, 2017.
W. Budiharto, “Data science approach to stock prices
forecasting in Indonesia during Covid-19 using Long
Short-Term Memory (LSTM),” Journal of Big Data,
vol. 8, 2021.
J. Pomponi, S. Scardapane, and A. Uncini, “Structured
Ensembles: an Approach to Reduce the Memory
Footprint of Ensemble Methods,” Neural Networks,
vol. 144, pp. 407-418, 2021.
Y. Yun and Y. Rui, “Application Validity Test of RSI Indi
cator in Bond Trading Decision,” Modern Business, no
DAML 2023 - International Conference on Data Analysis and Machine Learning
52