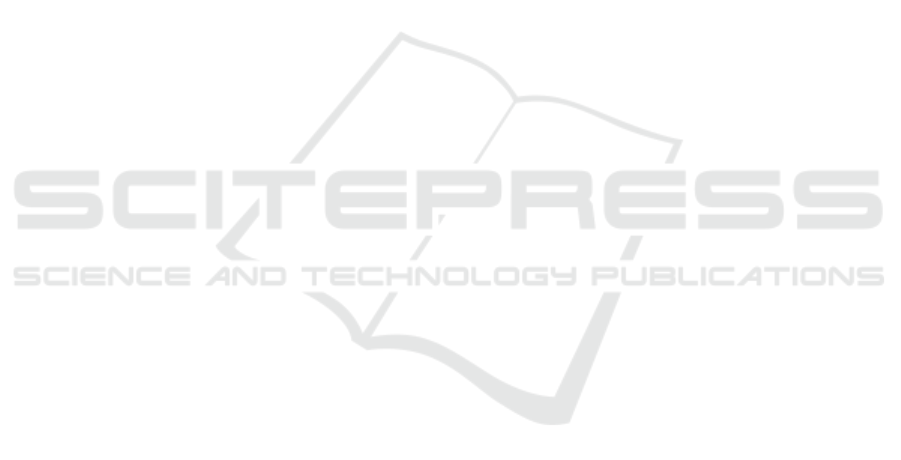
(0.0486). This outcome suggests potential limitations
in capturing fine-grained details when dealing with
Free-Form masks, resulting in larger reconstruction
errors. Consequently, in this specific task, Contextual
Attention may not be the optimal choice. In contrast,
both Gated Convolution and GC-Patch GAN methods
demonstrate lower L1 Loss and L2 Loss values.
Particularly, Gated Convolution stands out with
impressive scores, recording 0.1132 for L1 Loss and
0.0197 for L2 Loss, while GC-Patch GAN exhibits
even superior performance, with values of 0.0932 for
L1 Loss and 0.0145 for L2 Loss.
These findings underscore the enhanced efficacy
of GC-Patch GAN method in Free-Form mask image
restoration tasks, as they excel in accurately
reconstructing missing content. This advantage can
be attributed to their ability to effectively handle
Free-Form masks, enabling feature selection and
highlighting, thus mitigating reconstruction errors.
Consequently, opting for either of GC-Patch GAN
can lead to superior restoration outcomes in practical
applications.
4 CONCLUSION
This study has delved into the intricate domain of
image inpainting, with a specific emphasis on
tackling the complexities associated with Free-Form
masks. The proposed methodology, which combines
the Contextual Attention Layer, Gated Convolution,
and SN-PatchGAN, offers a comprehensive
framework to address these challenges effectively.
The GC-PatchGAN shows impressive improvements
by allowing to both capture strategic feature
information borrowing from known background
patches and selects and highlights relevant features
which lead to notable enhancements in inpainting
outcomes. Because of obtaining the continuity of
image texture, the GC-PatchGAN network can be
consistent with the overall chararteristics of the
images. Moreover, the discriminator is enhanced by a
refined focus on image details, successfully
mitigating noise irregularities across varying shapes
within the image. The generator ensures stability
when training a large number of images, and therefore
in conjunction with the discriminator can greatly
reduce the rate of loss. Extensive experiments are
meticulously conducted to evaluate the proposed
method, consistently demonstrating its exceptional
performance in diverse scenarios, including those
involving Free-Form masks and user-guided input.
These findings underscore the pivotal role of Gated
Convolution in advancing inpainting outcomes,
showcasing its effectiveness in generating high-
quality results. The future research in image
restoration will further explore the effectiveness of
image restoration for complex objects in images from
different scenarios and its applicability to high-
resolution images before super resolution, aiming to
refine and extend the model's capabilities to address
an array of challenges within the realm of image
restoration.
REFERENCES
P. F. Felzenszwalb, D. P. Huttenlocher, “Efficient Graph-
Based Image Segmentation,” International Journal of
Computer Vision, vol. 59, 2008, pp. 167-181.
A. Krizhevsky, I. Sutskever, G. E. Hinton, “ImageNet
Classification with Deep Convolutional Neural
Networks,” Advances in Neural Information
Processing Systems (NIPS), vol. 25, 2012.
D. Pathak, P. Krahenbuhl, J. Donahue, T. Darrell, A. A.
Efros, “Context Encoders: Feature Learning by
Inpainting,” In Proceedings of the IEEE Conference on
Computer Vision and Pattern Recognition (CVPR),
2016, pp. 2536-2544.
J. Long, E. Shelhamer, T. Darrell, “Fully Convolutional
Networks for Semantic Segmentation,” Proceedings of
the IEEE Conference on Computer Vision and Pattern
Recognition (CVPR), 2015, pp. 3431-3440.
L. C. Chen, G. Papandreou, F. Schroff, H. Adam,
“Rethinking Atrous Convolution for Semantic Image
Segmentation,” arXiv: 2018, unpublished.
O. Ronneberger, P. Fischer, T. Brox, “U-Net:
Convolutional Networks for Biomedical Image
Segmentation,” International Conference on Medical
Image Computing and Computer-Assisted Intervention
(MICCAI), 2015, pp. 234-241.
L. C. Chen, Y. Zhu, G. Papandreou, F. Schroff, H. Adam,
“Encoder-Decoder with Atrous Separable Convolution
for Semantic Image Segmentation,” arXiv:2017,
unpublished.
X. Wang, R. Girshick, A. Gupta, K. He, “Non-Local Neural
Networks,” Proceedings of the IEEE Conference on
Computer Vision and Pattern Recognition (CVPR),
2018, pp. 7794-7803.
A. G. Howard, M. Zhu, B. Chen, D. Kalenichenko, W.
Wang, T. Weyand, M. Andreetto, H. Adam,
“MobileNets: Efficient Convolutional Neural Networks
for Mobile Vision Applications,” arXiv:2017,
unpublished.
Dataset,
https://www.kaggle.com/datasets/arnaud58/landscape-
pictures.
DAML 2023 - International Conference on Data Analysis and Machine Learning
406