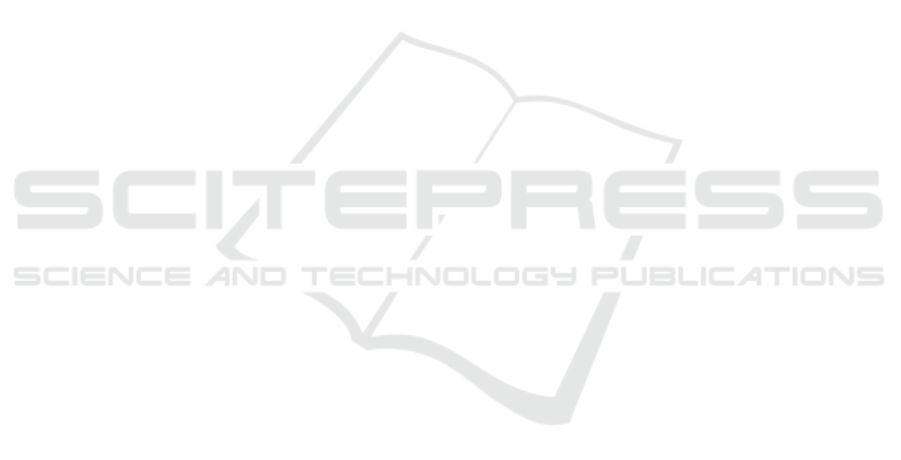
the transfer intensity, similar to our understanding of
the deadweight force in the life insurance actuarial,
then similarly
represents the intensity of the initial
state of i, and at the next moment the state of j. The
transfer probability between states is the probability
that the initial state is i, and at the next moment the
state is j. The first letter or number is generally a
transferable state, and the second letter or number is
generally a non-transferable state.
5 CONCLUSION
This paper uses traditional insurance pricing
calculations to see that premiums gradually increase
with age, peaking in the 61-70 age group. With age,
the body's function declines, immunity weakens, and
the risk of malignant tumors increases, so the premium
calculation results are in line with common sense.
Through the case study in this paper, this paper
found that this paper can develop and optimize in the
following areas in the future. In terms of data-driven
precision pricing, with the development of big data
technology, actuarial research on insurance pricing
will pay more attention to the in-depth analysis of the
incidence rate, mortality rate, and treatment cost of
patients with malignant tumors by using big data
technology, so as to realize the precision of pricing
malignant tumor insurance. By mining and analyzing
a large amount of data, insurance companies can be
provided with a more scientific and reasonable basis
for pricing, reducing the risk exposure of insurance
companies and improving the competitiveness of
insurance products. In terms of comprehensive
protection solutions, future actuarial research on
insurance pricing will focus more on the development
of comprehensive malignant tumor insurance products,
covering a variety of treatment modalities, such as
chemotherapy, radiotherapy and surgery, to provide
customers with all-around protection. In addition,
cooperation with related industries such as medical
care and rehabilitation can also be considered to
provide customers with a full range of services from
prevention, and treatment to rehabilitation. Finally, in
terms of policy support and regulation, government
policy support and regulation in malignant tumor
insurance pricing will have a positive impact on
actuarial research. The government can encourage
insurance companies to develop insurance products
for malignant tumors by adjusting tax policies and
providing financial subsidies. The theory of major
disease insurance pricing based on the Markov model
can be used as a basic model for practical application.
Under the premise of data support, according to the
algorithmic process of this paper, more states (e.g.,
healthy, mild, moderate, severe, and death) can be
considered to be added, which has a positive reference
significance to the industry's pricing of critical illness
insurance.
In conclusion, future actuarial research on
insurance pricing in China in terms of malignancy
insurance pricing is expected to achieve precision,
personalization, synthesis, innovation, and
standardization to meet market demand, reduce
patient burden, and promote the healthy development
of the insurance market.
REFERENCES
S. M. Adyani, E Gol-Alizadeh. The Need for
Complementary Health Insurance in Iran and
Suggestions for Its Development. Hospital Practices and
Research, 2018, pp. 146.
C. C. Koay. Development of Critical Illness Insurance in
Selected Markets, 2003.
F. Baione, S. Levantesi. A health insurance pricing model
based on prevalence rates: Application to critical illness
insurance. insurance, Insurance Mathematics and
Economics, 2014, vol. 58, pp. 174-184.
BL. Jone. Modeling Multi-State Processes using a Markov
Assumption. Actuarial Research Clearing House,1993,
vol.1.
E. Pitacco. Actuarial models for pricing disability benefits:
Towards a unifying approach. Insurance: Mathematics
and Economics, 1995, vol. 16, pp. 39-62.
M. C. Christiansen. Multistate Models in Health Insurance.
AStA Advances in Statistical Analysis, 2012, vol. 96,
no. 2, pp. 155‒186.
Z. Hailong. Research on pricing of critical illness insurance
under the new critical illness table. Shanxi University of
Finance and Economics, 2023.
S. Yaling. Pricing research on China's critical illness
insurance under the new critical illness table. Hunan
University, 2022.
US. Pasaribu, H. Husniah, RRKN. Sari, et al. Pricing critical
illness insurance premiums using multiple state
continous markov chain model. Journal of Physics:
Conference Series. IOP Publishing, 2019, vol. 1366, no.
1, 012112.
Q. Chunjuan, L. Yinhuan, T. Xinyue, et al. A study on
pricing of critical illness insurance in the framework of
Markov modeling-estimation based on mortality
effectiveness and morbidity intensity. Statistical
Research, 2023, vol. 40, no. 05, pp. 152-160.
Critical Illness Insurance Pricing and Markov Optimization Model: Based on the Analysis of Severe Malignancies in China
65