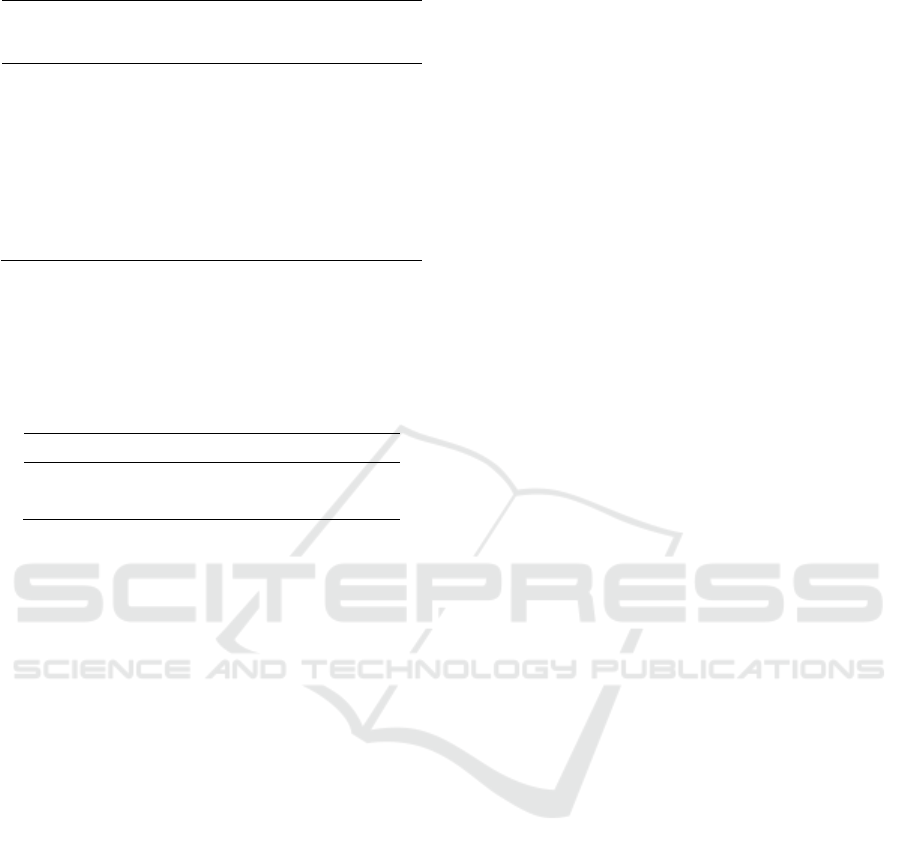
Table 3: Prediction of the Brith Rate from 2021 to 2027.
Table 4 shows observed and projected values for
2021 and 2022. Predicted values are higher than
observed values. The difference between the two
predictions is about two thousandths of a cent.
Table 4: Prediction of the Brith Rate from 2021 to 2027.
4 CONCLUSION
The study builds an ARIMA model on the time series
created from 74 data points to predict trends in
fertility, and screen for the optimal model using the
AIC criterion. The ARIMA (0,1,2) model has the
lowest AIC value of 357.08. The model is used to
predict future fertility trends and it is found that the
fertility rate does not show a short-term upward trend.
Birth rates are all below 7 per 1,000 live births for the
next five years. Re-modelling excluding the data
points after the policy change results in a birth rate
above 8 per 1,000 in 2021 and 2022. The projections
for 2021 and 2022 are significantly higher than the
actual values. This result suggests that policy changes
have failed to raise the birth rate. The rate of fertility
decline in China is accelerating. It also shows that
China's social problems have not been solved.
Time series analysis is good at extracting seasonal
and trend information. The analysis of external
factors is not perfect. The reason for the decline in the
birth rate may have been influenced by external
factors, such as new crown pneumonia. For the
impact of policy, this paper also fails to take into
account in the form of variables. For these reasons,
the accuracy of the model may be reduced. It is hoped
that the study will inform policy development and
allow for more accurate modelling in the future.
REFERENCES
J. Y. Zhao and X. Sun, “Age-specific fertility projections
based on combined fertility models,” Statistics and
Decision Making. vol. 13, pp. 102-105, 2015.
Y. B. Wang, S. T. Ma, H. Wang and J. Yuan, “Changes in
fertility levels of women and future population
projections in China,” China Health Statistics, pp. 34,
2017.
A. Yage, “Analysis of China's population projection based
on changes in population fertility policy,” Liaoning
University. 2017.
Y. W. Wang, “Forecasting and analysis of China's two-
child fertility rate based on functional data mode,” East
China Normal University. 2018.
J. L. Xue, “Analysis of world population fertility change
and its influencing factors-And judgement on China's
future fertility,” Journal of Xi'an Finance and
Economics College. Vol. 31(06), pp. 100-106, 2018.
W. X. Zheng, X. Wu and Y. Liu, “Survey research on two-
child birth intention and influencing factors of highly
educated group--Taking a university in Weifang as an
example,” Industry and Technology Forum. vol.
18(24), pp. 88-90, 2019.
M. G. Wang, “Retrospective and predictive study of
fertility in China from 2003 to 2018,” Science News.
Vol. 02, pp. 147, 2020.
X. J. Li, “Estimation, forecasting and analysis of
influencing factors of provincial fertility rate in
China,” Northeast University of Finance and
Economics. 2021.
J. X. Wang, et al. “Research on forecasting based on
machine learning algorithms-An example of
forecasting the number of aged population in China,”
China Information. Vol. 05, pp. 48-49, 2023.
J. Y. Chen, “A study on the population, structure and
influence of family planning policy adjustment,”
Proceedings of the 2016 International Conference on
Education, Sports, Arts and Management Engineering.
2016.
Y. Tao, et al. “The Impact of Population Aging in China
Based on Multiple Linear Regression Mode,” 4th
International Conference on Advanced Education and
Management (ICAEM 2017). 2017.
P. Y. Yan, “Forecast of China's Future Population under
the Universal Two-child Policy,” Proceedings of the
4th Workshop on Advanced Research and Technology
in Industry (WARTIA 2018). 2018.
DAML 2023 - International Conference on Data Analysis and Machine Learning
70