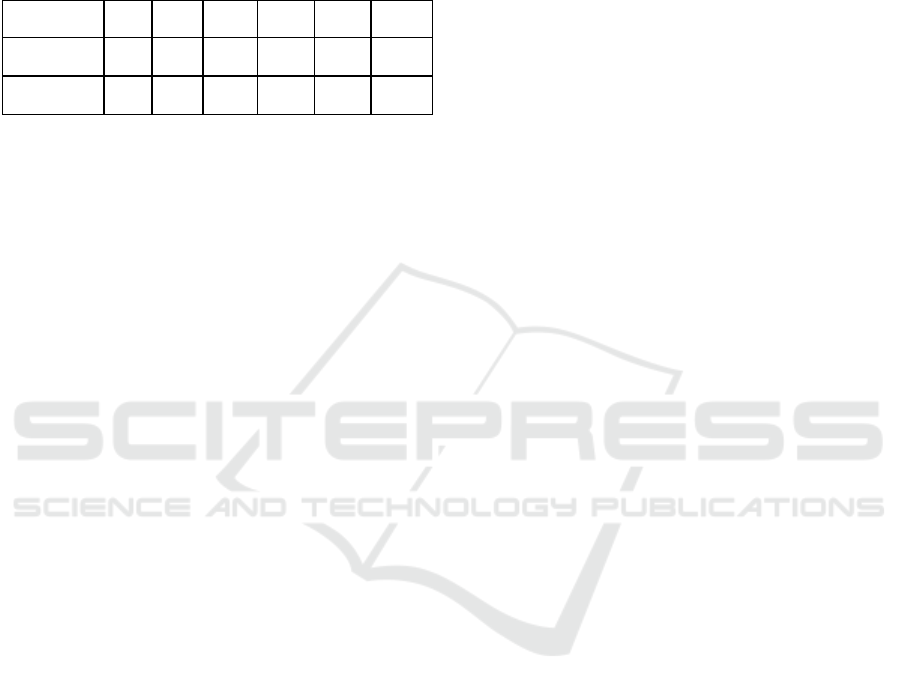
Perceptual Index (PI) denotes Perceptual Index, while
PieAPP signifies Perceptual Image-Error Assessment
through Pairwise Preference. Notably, an asterisk
symbol indicates that smaller values indicate superior
performance. Comparative results of the two models
are provided in Table 1.
Table 1: Comparative Results of Two Models.
In terms of quantitative quality (PSNR and SSIM),
the "L1+VGG+PieAPP" loss produced better results.
This means clearer, sharper images with higher
contrast and better structural similarity. Also, the
improved model performs better on LPIPS DMOS and
PieAPP metrics. An image with a low score is more
likely to be accurate, harder for a human to tell apart,
and boost user happiness. In addition, low scores help
reduce storage and bandwidth requirements for image
transmission. In addition, it creates a reconstruction
image with more accurate texture features based on the
results of subjective human eye observation. Different
metrics are more applicable in different contexts, so it
is often necessary to consider multiple metrics to fully
assess image quality. The performance of the improved
model is still inferior to the original model in terms of
PI, and how to improve the performance in terms of PI
will be a goal for the future. In summary, the improved
ESRGAN model with an additional PieAPP loss has
better performance for face restoration than the
original ESRGAN model.
4 CONCLUSION
This study explored the fascinating field of face picture
super-resolution reconstruction with the goal of
improving the visual appeal and perceptual accuracy of
facial images. The proposed method, an improved
ESRGAN model integrated with an innovative PieAPP
loss function, has been thoroughly examined and
showcased as a powerful tool for face restoration. The
loss function is proposed to analyze and refine the
super-resolution process. Leveraging the removal of
Batch Normalization layers and the incorporation of
the RRDB in the generator's architecture, as well as
introducing the PieAPP loss, the model is designed to
produce clearer and more natural high-resolution facial
images. The effectiveness of the suggested strategy is
assessed by extensive experiments using a variety of
evaluation indices. The results consistently
demonstrated that our "L1+VGG+PieAPP" loss
function outperforms the original ESRGAN model
across various quantitative metrics, including PSNR,
SSIM, LPIPS, DMOS, and PieAPP, resulting in
superior perceptual image quality and user satisfaction.
In the future, this research will turn its attention to
optimizing loss functions as the primary research
objective for the next stage. In an effort to improve the
field of face image super-resolution and contribute to
the creation of more accurate and aesthetically
acceptable facial reconstructions, the emphasis will be
on the in-depth investigation of alternative loss
functions designed expressly for face image super-
resolution.
REFERENCES
Y. Hong, U. Hwang, J. Yoo, et al. “How generative
adversarial networks and their variants work: An
overview,” ACM Computing Surveys (CSUR), vol. 52,
2019, pp. 1-43.
J. Lin, T. Zhou, Z. Chen, “Multi-scale face restoration with
sequential gating ensemble network,” Proceedings of
the AAAI Conference on Artificial Intelligence, vol. 32,
2018.
M. Cao, Z. Liu, X. Huang, et al. “Research for face image
super-resolution reconstruction based on wavelet
transform and SRGAN,” 2021 IEEE 5th Advanced
Information Technology, Electronic and Automation
Control Conference (IAEAC). IEEE, vol. 5, 2021, pp.
448-451.
T. Karras, M. Aittala, S. Laine, et al. “Alias-free generative
adversarial networks,” Advances in Neural Information
Processing Systems, vol. 34, 2021, pp. 852-863.
N. Zhang, Y. Wang, X. Zhang, et al. “An unsupervised
remote sensing single-image super-resolution method
based on generative adversarial network,” IEEE Access,
vol. 8, 2020, pp. 29027-29039.
J. A. Parker, R. V. Kenyon, D. E. Troxel, “Comparison of
interpolating methods for image resampling,” IEEE
Transactions on medical imaging, vol. 2, 1983, pp. 31-
39.
T. Tong, G. Li, X. Liu, et al. “Image super-resolution using
dense skip connections,” Proceedings of the IEEE
international conference on computer vision, 2017, pp.
4799-4807.
C. Ledig, L. Theis, F. Huszár, et al. “Photo-realistic single
image super-resolution using a generative adversarial
network,” Proceedings of the IEEE conference on
computer vision and pattern recognition, 2017, pp.
4681-4690.
Y. Choi, H. Park, “Improving ESRGAN with an additional
image quality loss,” Multimedia Tools and
Applications, vol. 82, 2023, pp. 3123-3137.
Face Image Super-Resolution Reconstruction Based on the Improved ESRGAN
475