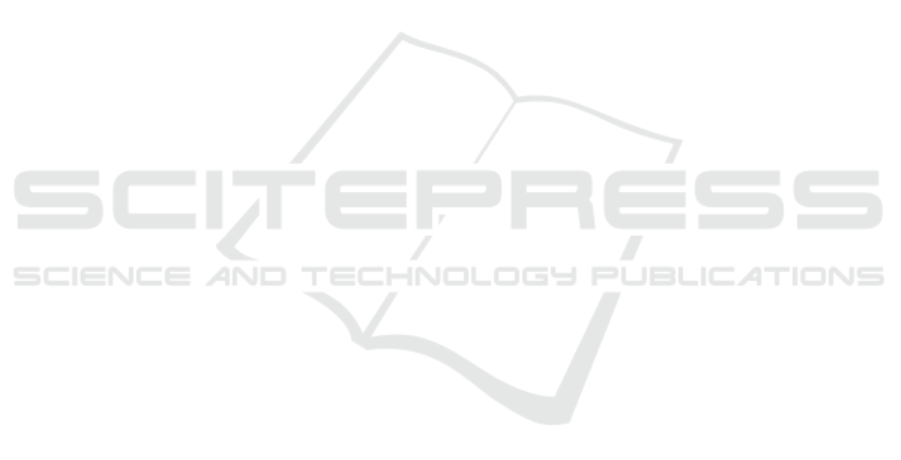
errors arising from artificial intelligence technology.
Despite these legal impediments, the promising
potential of AI/ML in pharmacovigilance remains
evident, prompting a critical examination of how to
harness these technologies effectively to construct a
future fit for purpose. Establishing a seamlessly
connected system for the flow of inputs and outputs
across diverse data systems emerges as a critical
imperative. Such a system would not only foster an
interactive continual learning solution but also
enhance the understanding of the benefit–risk profiles
of medicines and vaccines. Additionally, it would
empower prescribers, patients, and other stakeholders
to obtain pertinent information and pose inquiries as
needed, thereby contributing to a more informed and
responsive healthcare ecosystem.
REFERENCES
Caster, O. (2018). Benefit-Risk Assessment in
Pharmacovigilance. In A. Bate (Ed.), Evidence-Based
Pharmacovigilance (Methods in Pharmacology and
Toxicology). Humana Press.
https://doi.org/10.1007/978-1-4939-8818-1_13
Coleman, T. S. (2012). Quantitative Risk Management: A
Practical Guide to Financial Risk, (B. Litterman,
Foreword).
Deng, L., & Chang, Y. (2022). Risk Management of
Investment Projects Based on Artificial Neural
Network. Wireless Communications and Mobile
Computing, 2022, Article ID 5606316, 13 pages.
https://doi.org/10.1155/2022/5606316
Huysentruyt, K., Kjoersvik, O., Dobracki, P., Savage, E.,
Mishalov, E., Cherry, M., Leonard, E., Taylor, R.,
Patel, B., & Abatemarco, D. (2021). Validating
Intelligent Automation Systems in Pharmacovigilance:
Insights from Good Manufacturing Practices. Drug
Safety, 44(3), 261-272. https://doi.org/10.1007/s40264-
020-01030-2
Pascarella, G., Rossi, M., Montella, E., Capasso, A., De
Feo, G., Botti, G., Nardone, A., Montuori, P., Triassi,
M., D'Auria, S., & Morabito, A. (2021, July 8). Risk
Analysis in Healthcare Organizations: Methodological
Framework and Critical Variables. Risk Management
and Healthcare Policy, 14, 2897-2911.
https://doi.org/10.2147/RMHP.S309098
Hamid, A. A. A., Rahim, R., & Teo, S. P. (2022).
Pharmacovigilance and Its Importance for Primary
Health Care Professionals. Korean Journal of Family
Medicine, 43(5), 290-295.
https://doi.org/10.4082/kjfm.21.0193
Expert Group on Clinical Trials. (2017, April 25). Risk
proportionate approaches in clinical trials:
Recommendations of the expert group on clinical trials
for the implementation of Regulation (EU) No
536/2014 on clinical trials on medicinal products for
human use.
Randeep, V. C., Divya, P., Susmita, A., Sushmitha, P.,
Ramya, Ch., & Chandini, K. (2022, December 12).
Automation in pharmacovigilance: Artificial
intelligence and machine learning for patient safety.
Journal of Innovations in Applied Pharmaceutical
Science (JIAPS), 7(3), 118-122.
https://doi.org/10.37022/jiaps.v7i3.37 Hillson, D.
(2023). The Risk Management Handbook: A Practical
Guide to Managing the Multiple Dimensions of Risk
(6th ed.). Routledge.
Ito, S., & Narukawa, M. (2022). Estimation of the Under-
Reporting of Suspected Serious Adverse Drug
Reactions in Japan Using An Interrupted Time Series
Analysis. Therapeutic Innovation & Regulatory
Science, 56(2), 358–365.
https://doi.org/10.1007/s43441-022-00379-z
García-Abeijon, P., Costa, C., Taracido, M., Herdeiro, M.
T., Torre, C., & Figueiras, A. (2023). Factors
Associated with Underreporting of Adverse Drug
Reactions by Health Care Professionals: A Systematic
Review Update. Drug Safety, 46(7), 625–636.
https://doi.org/10.1007/s40264-023-01302-7
Drukker, K., Chen, W., Gichoya, J., Gruszauskas, N.,
Kalpathy-Cramer, J., Koyejo, S., Myers, K., Sá, R.C.,
Sahiner, B., Whitney, H., Zhang, Z., & Giger, M.
(2023). Toward fairness in artificial intelligence for
medical image analysis: Identification and mitigation of
potential biases in the roadmap from data collection to
model deployment. Journal of Medical Imaging
(Bellingham), 10(6), 061104. doi:
10.1117/1.JMI.10.6.061104.
Sartori, D., Aronson, J. K., & Onakpoya, I. J. (2020).
Signals of adverse drug reactions communicated by
pharmacovigilance stakeholders: Protocol for a scoping
review of the global literature. Systematic Reviews,
9(1), 180. doi: 10.1186/s13643-020-01429-z.
Garcia-Agundez, A., García-Martín, E., & Eickhoff, C.
(2022, May 20). Editorial: The Potential of Machine
Learning in Pharmacogenetics, Pharmacogenomics and
Pharmacoepidemiology. Frontiers in Pharmacology,
13, 928527. https://doi.org/10.3389/fphar.2022.928527
Wewering, S., Pietsch, C., Sumner, M., Markó, K., Lülf-
Averhoff, A. T., & Baehrens, D. (2022, June). Machine
learning approach to identify adverse events in
scientific biomedical literature. Clinical and
Translational Science, 15(6), 1500-1506.
https://doi.org/10.1111/cts.13268
Yang, S., & Kar, S. (2023). Application of artificial
intelligence and machine learning in early detection of
adverse drug reactions (ADRs) and drug-induced
toxicity. Artificial Intelligence Chemistry, 1(2),
100011. https://doi.org/10.1016/j.aichem.2023.100011
Kim, H. R., Sung, M., Park, J. A., Jeong, K., Kim, H. H.,
Lee, S., & Park, Y. R. (2022). Analyzing adverse drug
reaction using statistical and machine learning
methods: A systematic review. Medicine (Baltimore),
101(25), e29387.
https://doi.org/10.1097/MD.0000000000029387
Lee, J. E., Kim, J. H., Bae, J. H., et al. (2022). Detecting
early safety signals of infliximab using machine
An Epistemological Approach to Risk Assessment in Pharmacovigilance and Mitigation Through Artificial Intelligence
35