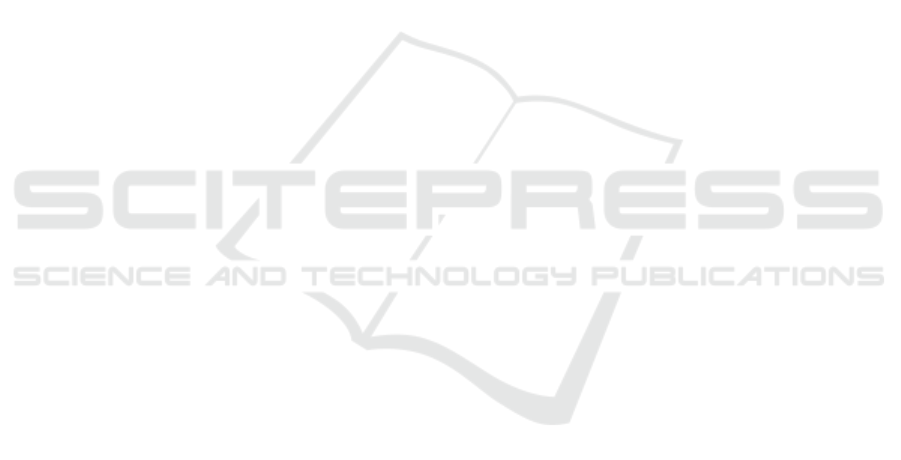
ACKNOWLEDGEMENTS
This work was partially funded within the Electronic
Components and Systems for European Leadership
(ECSEL) joint undertaking in collaboration with the
European Union’s H2020 Framework Program and
the Federal Ministry of Education and Research of the
Federal Republic of Germany (BMBF) under grant
agreement 16ESE0424/GA826600 (VIZTA), and par-
tially funded by the German Ministry of Educa-
tion and Research (BMBF) under Grant Agreement
01IW20002 (SocialWear).
REFERENCES
Agresti, G., Minto, L., Marin, G., and Zanuttigh, P. (2017).
Deep learning for confidence information in stereo
and tof data fusion. In IEEE International Con-
ference on Computer Vision Workshops (CVPR-W),
pages 697–705.
Barchid, S., Mennesson, J., and Dj
´
eraba, C. (2021). Review
on indoor rgb-d semantic segmentation with deep con-
volutional neural networks. In International Confer-
ence on Content-Based Multimedia Indexing (CBMI),
pages 1–4. IEEE.
Cao, J., Leng, H., Lischinski, D., Cohen-Or, D., Tu, C.,
and Li, Y. (2021). Shapeconv: Shape-aware convo-
lutional layer for indoor rgb-d semantic segmentation.
In IEEE/CVF International Conference on Computer
Vision (ICCV), pages 7088–7097.
Chen, Y., Mensink, T., and Gavves, E. (2019). 3d neigh-
borhood convolution: learning depth-aware features
for rgb-d and rgb semantic segmentation. In Inter-
national Conference on 3D Vision (3DV), pages 173–
182. IEEE.
Cheng, Y., Cai, R., Li, Z., Zhao, X., and Huang, K. (2017).
Locality-sensitive deconvolution networks with gated
fusion for rgb-d indoor semantic segmentation. In
IEEE Conference on Computer Vision and Pattern
Recognition (CVPR), pages 3029–3037.
Crawshaw, M. (2020). Multi-task learning with deep
neural networks: A survey. arXiv preprint
arXiv:2009.09796.
Hahne, U. (2012). Real-time depth imaging. PhD thesis,
Berlin Institute of Technology.
Hazirbas, C., Ma, L., Domokos, C., and Cremers, D.
(2017). Fusenet: Incorporating depth into semantic
segmentation via fusion-based cnn architecture. In
Asian Conference on Computer Vision, 2016, pages
213–228. Springer.
He, K., Zhang, X., Ren, S., and Sun, J. (2015). Delv-
ing deep into rectifiers: Surpassing human-level per-
formance on imagenet classification. In Proceedings
of the IEEE international conference on computer vi-
sion, pages 1026–1034.
Jiao, J., Wei, Y., Jie, Z., Shi, H., Lau, R. W., and Huang,
T. S. (2019). Geometry-aware distillation for indoor
semantic segmentation. In IEEE Conference on Com-
puter Vision and Pattern Recognition (CVPR).
Katrolia, J. S., El-Sherif, A., Feld, H., Mirbach, B., Ram-
bach, J. R., and Stricker, D. (2021a). Ticam: A time-
of-flight in-car cabin monitoring dataset. In British
Machine Vision Conference (BMVC), page 277.
Katrolia, J. S., Kr
¨
amer, L., Rambach, J., Mirbach, B., and
Stricker, D. (2021b). Semantic segmentation in depth
data: A comparative evaluation of image and point
cloud based methods. In 2021 IEEE International
Conference on Image Processing (ICIP), pages 649–
653. IEEE.
Levin, A., Lischinski, D., and Weiss, Y. (2004). Coloriza-
tion using optimization. ACM Transactions on Graph-
ics, 23.
Lim, G. M., Jatesiktat, P., Kuah, C. W. K., and Ang,
W. T. (2019). Hand and object segmentation from
depth image using fully convolutional network. In
International Conference of the IEEE Engineering in
Medicine and Biology Society (EMBC), pages 2082–
2086. IEEE.
Lorenti, L., Giacomantone, J., and Bria, O. N. (2018). Un-
supervised tof image segmentation through spectral
clustering and region merging. Journal of Computer
Science & Technology, 18.
Mao, J., Li, J., Li, F., and Wan, C. (2020). Depth image
inpainting via single depth features learning. In In-
ternational Congress on Image and Signal Process-
ing, BioMedical Engineering and Informatics (CISP-
BMEI), pages 116–120. IEEE.
Misra, I., Shrivastava, A., Gupta, A., and Hebert, M. (2016).
Cross-stitch networks for multi-task learning. In Pro-
ceedings of the IEEE conference on computer vision
and pattern recognition, pages 3994–4003.
Rezaei, M., Farahanipad, F., Dillhoff, A., Elmasri, R., and
Athitsos, V. (2021). Weakly-supervised hand part seg-
mentation from depth images. In PErvasive Technolo-
gies Related to Assistive Environments, pages 218–
225.
Schneider, P., Anisimov, Y., Islam, R., Mirbach, B., Ram-
bach, J., Stricker, D., and Grandidier, F. (2022a).
Timo—a dataset for indoor building monitoring with
a time-of-flight camera. Sensors, 22(11):3992.
Schneider, P., Rambach, J., Mirbach, B., and Stricker,
D. (2022b). Unsupervised anomaly detection from
time-of-flight depth images. In Proceedings of the
IEEE/CVF Conference on Computer Vision and Pat-
tern Recognition, pages 231–240.
Song, H., Liu, Z., Du, H., Sun, G., Le Meur, O., and Ren, T.
(2017). Depth-aware salient object detection and seg-
mentation via multiscale discriminative saliency fu-
sion and bootstrap learning. IEEE Transactions on
Image Processing, 26(9):4204–4216.
Su, S., Heide, F., Swanson, R., Klein, J., Callenberg, C.,
Hullin, M., and Heidrich, W. (2016). Material clas-
sification using raw time-of-flight measurements. In
IEEE Conference on Computer Vision and Pattern
Recognition (CVPR), pages 3503–3511.
Wang, W. and Neumann, U. (2018). Depth-aware cnn for
Achieving RGB-D Level Segmentation Performance from a Single ToF Camera
177