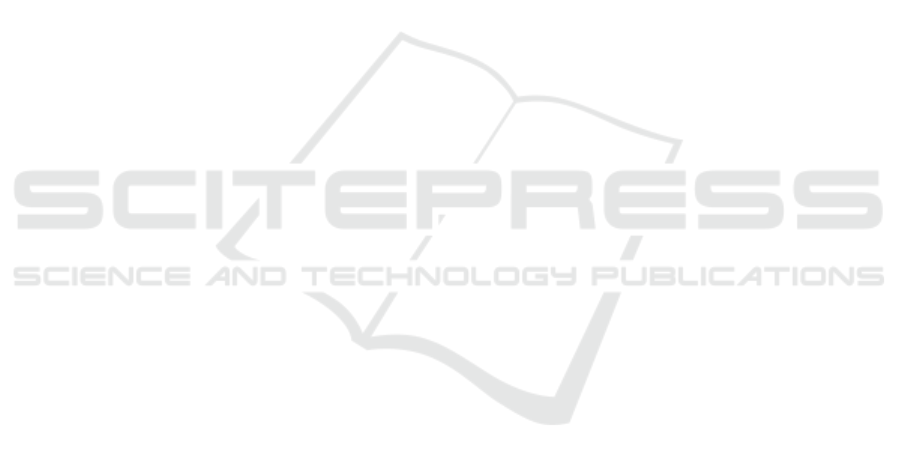
tion within GANs. As depicted in Fig.1, we present
a selection of meticulously manipulated face images,
showcasing attribute transformations from one to an-
other. Our pioneering approach leverages convolu-
tional layers within an auxiliary mapping network,
along with the integration of the CG method. These
results vividly demonstrate the precision and control
our method offers in manipulating diverse attributes,
including but not limited to smile, age, glasses, gen-
der, hair, and beard. The seamless transitions from
one attribute to another highlight the strength of our
method in disentangling complex interactions within
the latent space. These qualitative results provide
compelling visual evidence of the versatility and po-
tential of our approach for generating images that ef-
fectively reflect desired attribute changes while main-
taining the essential characteristics of the underlying
latent vectors.
6 CONCLUSION
In this paper, we presented a pioneering approach
to enhance latent space manipulation in GANs us-
ing convolutional layers and CG. Our method lever-
ages the power of auxiliary mapping to disentangle
latent semantics and achieve precise attribute manip-
ulations. Through extensive experiments on facial at-
tribute editing, we demonstrated the effectiveness and
superiority of our approach, surpassing existing state-
of-the-art methods in manipulation disentanglement
and image quality. The combination of auxiliary map-
ping and CG offers a promising direction for advanc-
ing GAN-based image generation and opens possibil-
ities for more sophisticated applications, such as gen-
erating images with multidimensional information.
REFERENCES
Abdal, R., Qin, Y., and Wonka, P. (2019). Image2stylegan:
How to embed images into the stylegan latent space?
In Proceedings of the IEEE/CVF international confer-
ence on computer vision, pages 4432–4441.
Brock, A., Donahue, J., and Simonyan, K. (2018). Large
scale gan training for high fidelity natural image syn-
thesis. arXiv preprint arXiv:1809.11096.
Cao, Q., Shen, L., Xie, W., Parkhi, O. M., and Zisserman,
A. (2018). Vggface2: A dataset for recognising faces
across pose and age. In 2018 13th IEEE international
conference on automatic face & gesture recognition
(FG 2018), pages 67–74. IEEE.
Goodfellow, I., Pouget-Abadie, J., Mirza, M., Xu, B.,
Warde-Farley, D., Ozair, S., Courville, A., and Ben-
gio, Y. (2014). Generative adversarial nets. Advances
in neural information processing systems, 27.
H
¨
ark
¨
onen, E., Hertzmann, A., Lehtinen, J., and Paris, S.
(2020). Ganspace: Discovering interpretable gan con-
trols. Advances in neural information processing sys-
tems, 33:9841–9850.
Hu, J., Shen, L., and Sun, G. (2018). Squeeze-and-
excitation networks. In Proceedings of the IEEE con-
ference on computer vision and pattern recognition,
pages 7132–7141.
Jahanian, A., Chai, L., and Isola, P. (2019). On the” steer-
ability” of generative adversarial networks. arXiv
preprint arXiv:1907.07171.
Karras, T., Aila, T., Laine, S., and Lehtinen, J. (2017). Pro-
gressive growing of gans for improved quality, stabil-
ity, and variation. arXiv preprint arXiv:1710.10196.
Karras, T., Laine, S., and Aila, T. (2019). A style-based
generator architecture for generative adversarial net-
works. In Proceedings of the IEEE/CVF conference
on computer vision and pattern recognition, pages
4401–4410.
Karras, T., Laine, S., Aittala, M., Hellsten, J., Lehtinen,
J., and Aila, T. (2020). Analyzing and improving
the image quality of stylegan. In Proceedings of the
IEEE/CVF conference on computer vision and pattern
recognition, pages 8110–8119.
Li, M., Jin, Y., and Zhu, H. (2021). Surrogate gradient field
for latent space manipulation. In Proceedings of the
IEEE/CVF Conference on Computer Vision and Pat-
tern Recognition, pages 6529–6538.
Powell, M. J. (1984). Nonconvex minimization calcula-
tions and the conjugate gradient method. In Numerical
Analysis: Proceedings of the 10th Biennial Confer-
ence held at Dundee, Scotland, June 28–July 1, 1983,
pages 122–141. Springer.
Radford, A., Metz, L., and Chintala, S. (2015). Unsu-
pervised representation learning with deep convolu-
tional generative adversarial networks. arXiv preprint
arXiv:1511.06434.
Ramachandran, P., Zoph, B., and Le, Q. V. (2017).
Searching for activation functions. arXiv preprint
arXiv:1710.05941.
Shen, Y., Zhou, B., Luo, P., and Tang, X. (2018). Facefeat-
gan: a two-stage approach for identity-preserving face
synthesis. arXiv preprint arXiv:1812.01288.
Shewchuk, J. R. et al. (1994). An introduction to the conju-
gate gradient method without the agonizing pain.
Voynov, A. and Babenko, A. (2020). Unsupervised dis-
covery of interpretable directions in the gan latent
space. In International conference on machine learn-
ing, pages 9786–9796. PMLR.
Wu, Y. and He, K. (2018). Group normalization. In Pro-
ceedings of the European conference on computer vi-
sion (ECCV), pages 3–19.
Xia, W., Yang, Y., Xue, J.-H., and Wu, B. (2021). Tedi-
gan: Text-guided diverse face image generation and
manipulation. In Proceedings of the IEEE/CVF con-
ference on computer vision and pattern recognition,
pages 2256–2265.
Yang, C., Shen, Y., and Zhou, B. (2021). Semantic hier-
archy emerges in deep generative representations for
scene synthesis. International Journal of Computer
Vision, 129:1451–1466.
Conjugate Gradient for Latent Space Manipulation
57