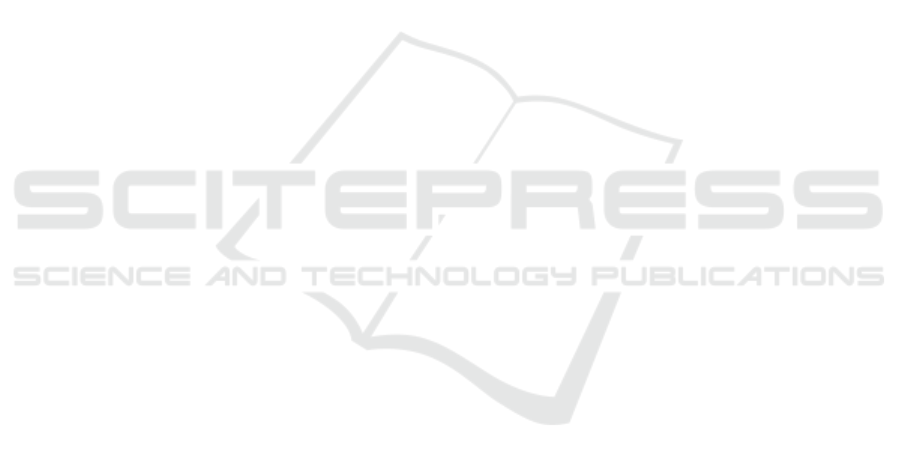
work, we will look into the possibility of improving
these functions with the use of multi-processing or by
vectorizing the computations.
6 CONCLUSION
In this paper, we modeled the transformations that
iPSC-ECs undergo in the process of tumor angiogen-
esis in a microfluidics environment. The angiogen-
esis is guided by a gradient of VEGF. The model’s
behaviour was tested in various scenarios. Moreover,
sensitivity analysis and scalability analysis are con-
ducted to evaluate its performance.
The diffusion models were varied to find the most
realistic one for model simulation. The diffusion
approximation through random particle movement
reached results that were very similar to those with
normal diffusion. It suggests that random diffusion
can be used to approximate the VEGF diffusion. The
non-symmetric formation of branches is also more
comparable to the chaotic branching seen in the in
vitro models. However, both approaches have their
drawbacks. Where the normal diffusion is too perfect
to be realistic, the random diffusion may result in too
large ‘jumps’ of particles to be realistic. In addition,
we found that the initial amount of VEGF does not in-
fluence the speed of angiogenesis when using random
diffusion. But it is an essential parameter for regular
diffusion, where, with too low values of initial VEGF,
the angiogenesis does not start at all.
In this work, we used a rather simplified model
for cell movement, which only allows cells to move
upward. Side-way movement of tip cells should be
included in the future to make the model more real-
istic. Furthermore, the direction of motion should be
decided by the concentration of VEGF, while preserv-
ing contact with stalk cells.
Another limitation is the scale of the model with
a grid size 11x15. It is relatively small to indicate the
contribution of parameters to the uncertainty of the
output of the model. A larger grid size is preferred
for the sensitivity analysis. However, in scalability
analysis, we observed that the current implementation
has an exponential increase of running time when the
grid size grows logarithmically. In the future work,
functions with heavy computations should be further
investigated and the limitation on scalability of the
model should be solved using parallel computing.
REFERENCES
Bookholt, F. D., Monsuur, H. N., Gibbs, S., and Vermolen,
F. J. (2016). Mathematical modelling of angiogene-
sis using continuous cell-based models. Biomechanics
and Modeling in Mechanobiology, 15(6):1577–1600.
Borgonovo, E. (2007). A new uncertainty importance
measure. Reliability Engineering & System Safety,
92(6):771–784.
Carmeliet, P. and Jain, R. (2000). Carmeliet, p & jain, rk.
angiogenesis in cancer and other disease. nature, 407:
249-257. Nature, 407:249–57.
Carvalho, R. V., Verbeek, F. J., and Coelho, C. J. (2018).
Bio-modeling Using Petri Nets: A Computational Ap-
proach, pages 3–26. Springer International Publish-
ing, Cham.
Chaouiya, C. (2007). Petri net modelling of biological net-
works. Briefings in Bioinformatics, 8(4):210–219.
Chaouiya, C., Remy, E., and Thieffry, D. (2008). Petri net
modelling of biological regulatory networks. Journal
of Discrete Algorithms, 6(2):165–177. Selected pa-
pers from CompBioNets 2004.
Folkman, J. (2002). Role of angiogenesis in tumor growth
and metastasis. Seminars in Oncology, 29(6, Supple-
ment 16):15–18.
Harris, C. R., Millman, K. J., van der Walt, S. J., Gommers,
R., Virtanen, P., Cournapeau, D., Wieser, E., Taylor,
J., Berg, S., Smith, N. J., Kern, R., Picus, M., Hoyer,
S., van Kerkwijk, M. H., Brett, M., Haldane, A., del
R
´
ıo, J. F., Wiebe, M., Peterson, P., G
´
erard-Marchant,
P., Sheppard, K., Reddy, T., Weckesser, W., Abbasi,
H., Gohlke, C., and Oliphant, T. E. (2020). Array pro-
gramming with NumPy. Nature, 585(7825):357–362.
Hill, C. (2017). A very simple 2-d diffusion model.
Liu, F., Heiner, M., and Gilbert, D. (2017). Coloured Petri
nets for multilevel, multiscale and multidimensional
modelling of biological systems. Briefings in Bioin-
formatics, 20(3):877–886.
Matsuya, K., Yura, F., Mada, J., Kurihara, H., and Toki-
hiro, T. (2016). A discrete mathematical model for
angiogenesis. SIAM Journal on Applied Mathematics,
76(6):2243–2259.
Peirce, S. (2008). Computational and mathematical model-
ing of angiogenesis. Microcirculation (New York, N.Y.
: 1994), 15:739–51.
Phillips, C. M., Lima, E. A. B. F., Woodall, R. T., Brock, A.,
and Yankeelov, T. E. (2020). A hybrid model of tumor
growth and angiogenesis: In silico experiments. Plos
One, 15(4).
Pommereau, F. (2015). SNAKES: A flexible high-level
petri nets library (tool paper). In Application and The-
ory of Petri Nets and Concurrency, pages 254–265.
Springer International Publishing.
Querfeld, U., Mak, R., and Pries, A. (2020). Microvascular
disease in chronic kidney disease: The base of the ice-
berg in cardiovascular comorbidity. Clinical Science,
134:1333–1356.
Sainson, R. and Harris, A. (2008). Regulation of angiogen-
esis by homotypic and heterotypic notch signalling in
Modeling iPSC-Derived Endothelial Cell Transition in Tumor Angiogenesis Using Petri Nets
345