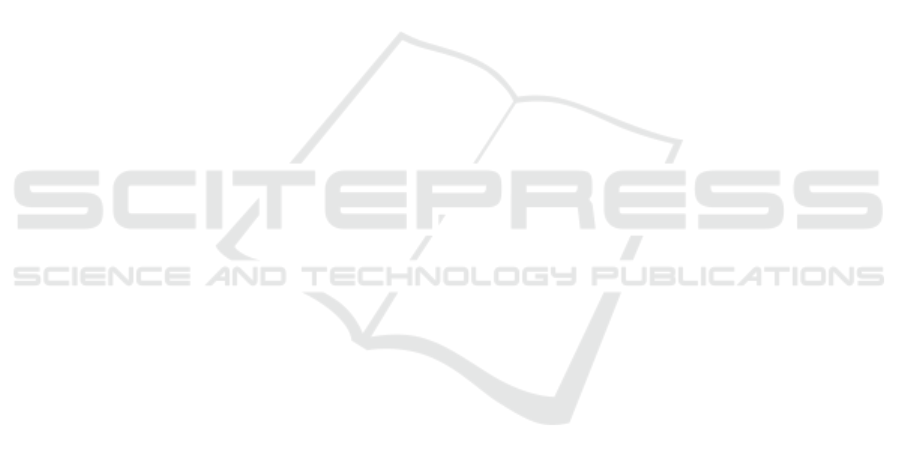
learning of semantic features. Advances in Neural In-
formation Processing Systems, 32.
Fang, C., Xu, Y., and Rockmore, D. N. (2013). Unbiased
metric learning: On the utilization of multiple datasets
and web images for softening bias. In Proceedings of
the IEEE International Conference on Computer Vi-
sion, pages 1657–1664.
Gal, Y. and Ghahramani, Z. (2016). Dropout as a bayesian
approximation: Representing model uncertainty in
deep learning. In international conference on machine
learning, pages 1050–1059. PMLR.
Ghifary, M., Kleijn, W. B., Zhang, M., and Balduzzi, D.
(2015). Domain generalization for object recognition
with multi-task autoencoders. In Proceedings of the
IEEE international conference on computer vision,
pages 2551–2559.
Grandvalet, Y. and Bengio, Y. (2004). Semi-supervised
learning by entropy minimization. Advances in neural
information processing systems, 17.
Gulrajani, I. and Lopez-Paz, D. (2020). In search
of lost domain generalization. arXiv preprint
arXiv:2007.01434.
He, K., Zhang, X., Ren, S., and Sun, J. (2016a). Deep resid-
ual learning for image recognition. In Proceedings of
the IEEE conference on computer vision and pattern
recognition, pages 770–778.
He, K., Zhang, X., Ren, S., and Sun, J. (2016b). Deep resid-
ual learning for image recognition. In CVPR, pages
770–778.
Hoffman, J., Tzeng, E., Darrell, T., and Saenko, K. (2017).
Simultaneous deep transfer across domains and tasks.
Domain Adaptation in Computer Vision Applications,
pages 173–187.
Huang, Z., Wang, H., Xing, E. P., and Huang, D. (2020).
Self-challenging improves cross-domain generaliza-
tion.
Kendall, A. and Cipolla, R. (2016). Modelling uncertainty
in deep learning for camera relocalization. In 2016
IEEE international conference on Robotics and Au-
tomation (ICRA), pages 4762–4769. IEEE.
Kendall, A. and Gal, Y. (2017). What uncertainties do we
need in bayesian deep learning for computer vision?
Advances in neural information processing systems,
30.
Khan, A., AlBarri, S., and Manzoor, M. A. (2022a). Con-
trastive self-supervised learning: a survey on different
architectures. In 2022 2nd International Conference
on Artificial Intelligence (ICAI), pages 1–6. IEEE.
Khan, A., Khattak, M. U., and Dawoud, K. (2022b). Ob-
ject detection in aerial images : A case study on per-
formance improvement. In 2022 International Con-
ference on Artificial Intelligence of Things (ICAIoT),
pages 1–9.
Khan, M. H., Zaidi, T., Khan, S., and Khan, F. S. (2021).
Mode-guided feature augmentation for domain gener-
alization. In Proc. Brit. Mach. Vis. Conf.
Kim, D., Yoo, Y., Park, S., Kim, J., and Lee, J. (2021). Self-
reg: Self-supervised contrastive regularization for do-
main generalization. In Proceedings of the IEEE/CVF
International Conference on Computer Vision, pages
9619–9628.
Krizhevsky, A., Sutskever, I., and Hinton, G. E. (2017). Im-
agenet classification with deep convolutional neural
networks. Communications of the ACM, 60(6):84–90.
Lakshminarayanan, B., Pritzel, A., and Blundell, C. (2017).
Simple and scalable predictive uncertainty estimation
using deep ensembles. Advances in neural informa-
tion processing systems, 30.
Li, D., Yang, Y., Song, Y.-Z., and Hospedales, T. M. (2017).
Deeper, broader and artier domain generalization. In
Proceedings of the IEEE international conference on
computer vision, pages 5542–5550.
Lin, L., Xie, H., Yang, Z., Sun, Z., Liu, W., Yu, Y., Chen,
W., Yang, S., and Xie, D. (2021). Semi-supervised
domain generalization in real world: New benchmark
and strong baseline. arXiv preprint arXiv:2111.10221.
Muandet, K., Balduzzi, D., and Sch
¨
olkopf, B. (2013). Do-
main generalization via invariant feature representa-
tion. In International conference on machine learning,
pages 10–18. PMLR.
Nesterov, Y. E. (1983). A method of solving a con-
vex programming problem with convergence rate
o\bigl(kˆ2\bigr). In Doklady Akademii Nauk, volume
269, pages 543–547. Russian Academy of Sciences.
Qi, L., Yang, H., Shi, Y., and Geng, X. (2022). Multimatch:
Multi-task learning for semi-supervised domain gen-
eralization. arXiv preprint arXiv:2208.05853.
Rizve, M. N., Duarte, K., Rawat, Y. S., and Shah, M.
In defense of pseudo-labeling: An uncertainty-aware
pseudo-label selection framework for semi-supervised
learning. In International Conference on Learning
Representations.
Robbins, H. and Monro, S. (1951). A stochastic approxi-
mation method. The annals of mathematical statistics,
pages 400–407.
Sajjadi, M., Javanmardi, M., and Tasdizen, T. (2016). Reg-
ularization with stochastic transformations and pertur-
bations for deep semi-supervised learning. Advances
in neural information processing systems, 29.
Shankar, S., Piratla, V., Chakrabarti, S., Chaudhuri, S.,
Jyothi, P., and Sarawagi, S. (2018). Generalizing
across domains via cross-gradient training. arXiv
preprint arXiv:1804.10745.
Shu, Y., Cao, Z., Wang, C., Wang, J., and Long, M. (2021).
Open domain generalization with domain-augmented
meta-learning. In Proceedings of the IEEE/CVF Con-
ference on Computer Vision and Pattern Recognition,
pages 9624–9633.
Smith, L. and Gal, Y. (2018). Understanding measures of
uncertainty for adversarial example detection. arXiv
preprint arXiv:1803.08533.
Sohn, K., Berthelot, D., Carlini, N., Zhang, Z., Zhang, H.,
Raffel, C. A., Cubuk, E. D., Kurakin, A., and Li, C.-L.
(2020). Fixmatch: Simplifying semi-supervised learn-
ing with consistency and confidence. Advances in neu-
ral information processing systems, 33:596–608.
Tarvainen, A. and Valpola, H. (2017). Mean teachers are
better role models: Weight-averaged consistency tar-
gets improve semi-supervised deep learning results.
Improving Pseudo-Labelling and Enhancing Robustness for Semi-Supervised Domain Generalization
299