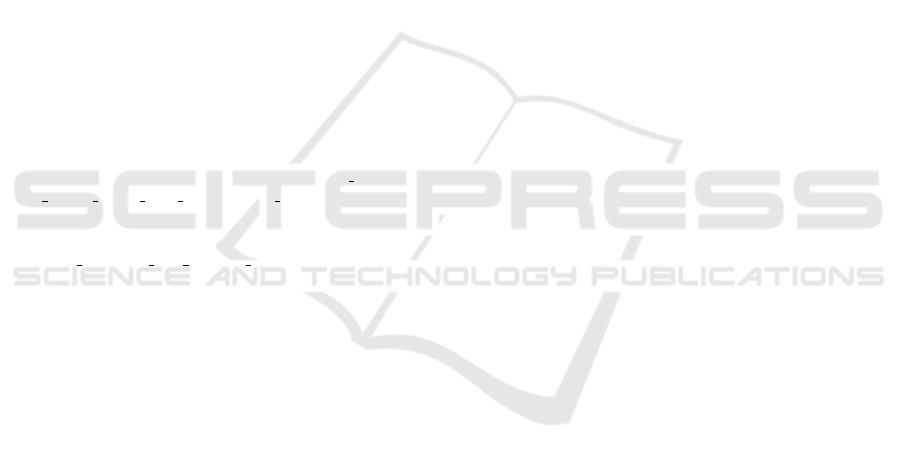
REFERENCES
Aifanti, N., Papachristou, C., and Delopoulos, A. (2010).
The mug facial expression database. In WIAMIS.
IEEE.
Avil
´
es, J., Toapanta, H., Morillo, P., and Vallejo-Huanga, D.
(2019). Dataset of Ethnic Facial Images of Ecuadorian
People.
Chen, J. M., Norman, J. B., and Nam, Y. (2021). Broaden-
ing the stimulus set: introducing the American mul-
tiracial faces database. Behavior Research Methods.
Chollet, F. (2017). Xception: Deep learning with depthwise
separable convolutions. In Proceedings of the IEEE
conference on computer vision and pattern recogni-
tion, pages 1251–1258.
Conley, M. I., Dellarco, D. V., Rubien-Thomas, E., Co-
hen, A. O., Cervera, A., Tottenham, B., and Casey,
B. (2018). The racially diverse affective expres-
sion (radiate) face stimulus set. Psychiatry Research,
270:1059–1067.
Damer, N., Saladi
´
e, A. M., Braun, A., and Kuijper, A.
(2018). Morgan: Recognition vulnerability and attack
detectability of face morphing attacks created by gen-
erative adversarial network. In 2018 IEEE 9th Inter-
national Conference on Biometrics Theory, Applica-
tions and Systems (BTAS), pages 1–10.
DeBruine, L. and Jones, B. (2017). Young Adult
White Faces with Manipulated Versions.
https://figshare.com/articles/dataset/Young Adult
White Faces with Manipulated Versions/4220517.
DeBruine, L. and Jones, B. (2021). Face Research
Lab London Set. https://figshare.com/articles/dataset/
Face Research Lab London Set/5047666.
Ferrara, M., Franco, A., and Maltoni, D. (2014). The magic
passport. In IEEE International Joint Conference on
Biometrics.
Grgic, M., Delac, K., and Grgic, S. (2011). SCface —
Surveillance Cameras Face Database. Multimedia
Tools and Applications, 51(3):863–879.
Hamza, M., Tehsin, S., Humayun, M., Almufareh, M. F.,
and Alfayad, M. (2022). A comprehensive review
of face morph generation and detection of fraudulent
identities. Applied Sciences, 12(24).
Horn, B. K. and Schunck, B. G. (1981). Determining optical
flow. Artificial Intelligence, 17(1):185–203.
International Civil Aviation Organization (2015). Doc 9303
- machine readable travel documents - part 10.
International Civil Aviation Organization (2018). Technical
report - portrait quality (reference facial images for
mrtd).
International Organization for Standardization (2017).
Iso/iec 30107-3:2017 information technology – bio-
metric presentation attack detection – part 3: Testing
and reporting.
Karras, T., Laine, S., Aittala, M., Hellsten, J., Lehtinen, J.,
and Aila, T. (2020). Analyzing and improving the
image quality of stylegan. In 2020 IEEE/CVF Con-
ference on Computer Vision and Pattern Recognition
(CVPR), pages 8107–8116.
Kasi
´
nski, A., Florek, A., and Schmidt, A. (2008). The PUT
face database. Image Processing and Communica-
tions, 13:59–64.
Kazemi, V. and Sullivan, J. (2014). One millisecond face
alignment with an ensemble of regression trees. In
Proceedings of the IEEE Conference on Computer Vi-
sion and Pattern Recognition (CVPR).
King, D. E. (2009). Dlib-ml: A machine learning toolkit.
Journal of Machine Learning Research, 10(60):1755–
1758.
Lakshmi, Wittenbrink, Correll, and Ma (2020). The In-
dia Face Set: International and Cultural Boundaries
Impact Face Impressions and Perceptions of Category
Membership. Frontiers in Psychology.
Ma, Kantner, and Wittenbrink (2020). Chicago Face
Database: Multiracial Expansion. Behavior Research
Methods.
Ma, D., Correll, J., and Wittenbrink, B. (2015). The
Chicago face database: A free stimulus set of faces
and norming data. Behavior Research Methods, 47.
Makrushin, A., Neubert, T., and Dittmann, J. (2017). Au-
tomatic generation and detection of visually fault-
less facial morphs. In VISIGRAPP, pages 39–50.
SciTePress.
Malolan, B., Parekh, A., and Kazi, F. (2020). Explain-
able deep-fake detection using visual interpretability
methods. In 2020 3rd International Conference on In-
formation and Computer Technologies (ICICT), pages
289–293.
Neubert, T., Makrushin, A., Hildebrandt, M., Kraetzer, C.,
and Dittmann, J. (2018). Extended stirtrace bench-
marking of biometric and forensic qualities of mor-
phed face images. IET Biometrics, 7(4):325–332.
Ngan, M., Patrick, G., Hanaoka, K., and Kuo, J. (2023).
Face recognition vendor test (frvt) part 4: Morph -
performance of automated face morph detection.
Paige, C. C. and Saunders, M. A. (1975). Solution of sparse
indefinite systems of linear equations. SIAM Journal
on Numerical Analysis, 12(4):617–629.
Phillips, P., Flynn, P., Scruggs, T., Bowyer, K., Chang, J.,
Hoffman, K., Marques, J., Min, J., and Worek, W.
(2005). Overview of the face recognition grand chal-
lenge. In CVPR’05, volume 1, pages 947–954 vol. 1.
Phillips, P., Wechsler, H., Huang, J., and Rauss, P. J. (1998).
The feret database and evaluation procedure for face-
recognition algorithms. Image and Vision Computing,
16(5):295–306.
Ramachandra, R., Raja, K., and Busch, C. (2016). Detect-
ing morphed face images. In Proc. International Con-
ference on Biometrics Theory, Applications and Sys-
tems (BTAS).
Ramachandra, R., Venkatesh, S., Raja, K., and Busch, C.
(2019). Towards making morphing attack detection
robust using hybrid scale-space colour texture fea-
tures. In IEEE 5th International Conference on Iden-
tity, Security, and Behavior Analysis, pages 1–8.
Sarkar, E., Korshunov, P., Colbois, L., and Marcel, S.
(2022). Are gan-based morphs threatening face recog-
nition? In ICASSP 2022 - 2022 IEEE International
VISAPP 2024 - 19th International Conference on Computer Vision Theory and Applications
280