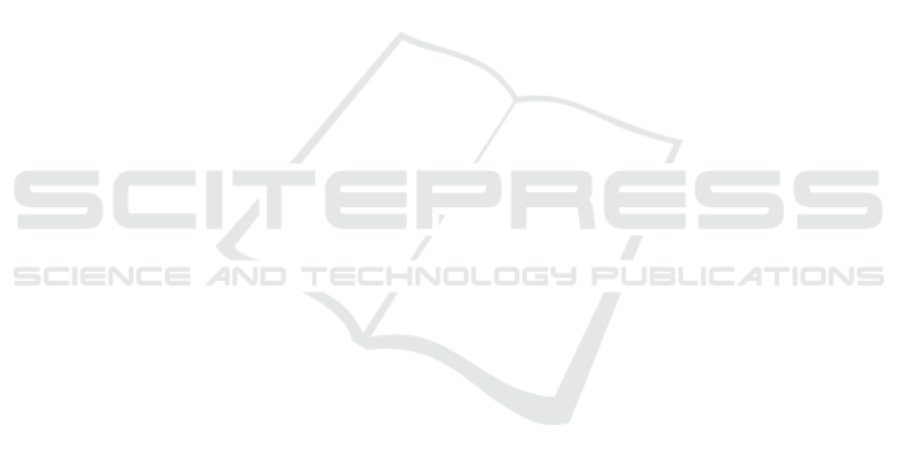
4.4.3 Visualizations
Figure 5 shows a coupling from known classes to
a new class that creates the synthesized classifiers.
Likewise the bird classes, the new class mostly looks
like the known classes that were assigned positive
weights (e.g., also tank-like vehicles), and different
from the ones that were assigned negative weights
(e.g., no barrel in front or manufactured in a differ-
ent country).
5 CONCLUSIONS
We have proposed a method that naturally ties zero-
and few-shot learning together, by synthesizing zero-
shot classifiers for new classes from classifiers from
known classes, with the option to optimize the clas-
sifiers further if a few labeled images are available.
We learned that the established classifiers are trans-
parent: their synthesis is based on a mapping that
can be visualized, which provides insights in which
known classes are used to synthesize the new classes.
The synthesis is simple and effective. We found that
it can outperform CLIP, which is a strong baseline. It
leads to better learning with as few as 1-4 training im-
ages, due to the improved starting point. Future work
includes diffusion models to derive the mapping for
synthesizing classifiers.
ACKNOWLEDGEMENTS
This material is based on research sponsored by Air
Force Research Laboratory (AFRL) under agreement
number FA8750-19-C-0514. The U.S. Government
is authorized to reproduce and distribute reprints for
Government purposes notwithstanding any copyright
notation therein. The views and conclusions con-
tained herein are those of the authors and should not
be interpreted as necessarily representing the official
policies or endorsements, either expressed or implied,
of Air Force Laboratory, DARPA or the U.S. Govern-
ment. The other sponsor is EDF: project 101103386
part of EDF-2021-DIGIT-R-2.
REFERENCES
Akata, Z., Perronnin, F., Harchaoui, Z., and Schmid, C.
(2015a). Label-embedding for image classification.
IEEE transactions on pattern analysis and machine
intelligence, 38(7):1425–1438.
Akata, Z., Reed, S., Walter, D., Lee, H., and Schiele,
B. (2015b). Evaluation of output embeddings for
fine-grained image classification. In Proceedings of
the IEEE conference on computer vision and pattern
recognition, pages 2927–2936.
Changpinyo, S., Chao, W.-L., Gong, B., and Sha, F. (2016).
Synthesized classifiers for zero-shot learning. In Pro-
ceedings of the IEEE conference on computer vision
and pattern recognition, pages 5327–5336.
Deng, J., Dong, W., Socher, R., Li, L.-J., Li, K., and Fei-
Fei, L. (2009). Imagenet: A large-scale hierarchical
image database. In 2009 IEEE conference on com-
puter vision and pattern recognition, pages 248–255.
IEEE.
Frome, A., Corrado, G. S., Shlens, J., Bengio, S., Dean,
J., Ranzato, M., and Mikolov, T. (2013). Devise: A
deep visual-semantic embedding model. Advances in
neural information processing systems, 26.
He, K., Zhang, X., Ren, S., and Sun, J. (2016). Deep resid-
ual learning for image recognition. In Proceedings of
the IEEE conference on computer vision and pattern
recognition, pages 770–778.
Huynh, D. and Elhamifar, E. (2021). Compositional
fine-grained low-shot learning. arXiv preprint
arXiv:2105.10438.
Jia, M., Tang, L., Chen, B.-C., Cardie, C., Belongie, S.,
Hariharan, B., and Lim, S.-N. (2022). Visual prompt
tuning. In European Conference on Computer Vision,
pages 709–727. Springer.
Lampert, C. H., Nickisch, H., and Harmeling, S. (2013).
Attribute-based classification for zero-shot visual ob-
ject categorization. IEEE transactions on pattern
analysis and machine intelligence, 36(3):453–465.
Liu, L., Jiang, H., He, P., Chen, W., Liu, X., Gao, J., and
Han, J. (2020). On the variance of the adaptive learn-
ing rate and beyond. In International Conference on
Learning Representations.
OpenAI (2023). ChatGPT. Accessed: 2023-04-21.
Parnami, A. and Lee, M. (2022). Learning from few exam-
ples: A summary of approaches to few-shot learning.
arXiv preprint arXiv:2203.04291.
Patterson, G. and Hays, J. (2012). Sun attribute database:
Discovering, annotating, and recognizing scene at-
tributes. In 2012 IEEE conference on computer vision
and pattern recognition, pages 2751–2758. IEEE.
Pourpanah, F., Abdar, M., Luo, Y., Zhou, X., Wang, R.,
Lim, C. P., Wang, X.-Z., and Wu, Q. J. (2022). A re-
view of generalized zero-shot learning methods. IEEE
transactions on pattern analysis and machine intelli-
gence.
Radford, A., Kim, J. W., Hallacy, C., Ramesh, A., Goh, G.,
Agarwal, S., Sastry, G., Askell, A., Mishkin, P., Clark,
J., et al. (2021). Learning transferable visual models
from natural language supervision. In International
Conference on Machine Learning, pages 8748–8763.
PMLR.
Romera-Paredes, B. and Torr, P. (2015). An embarrassingly
simple approach to zero-shot learning. In Interna-
tional conference on machine learning, pages 2152–
2161. PMLR.
VISAPP 2024 - 19th International Conference on Computer Vision Theory and Applications
56