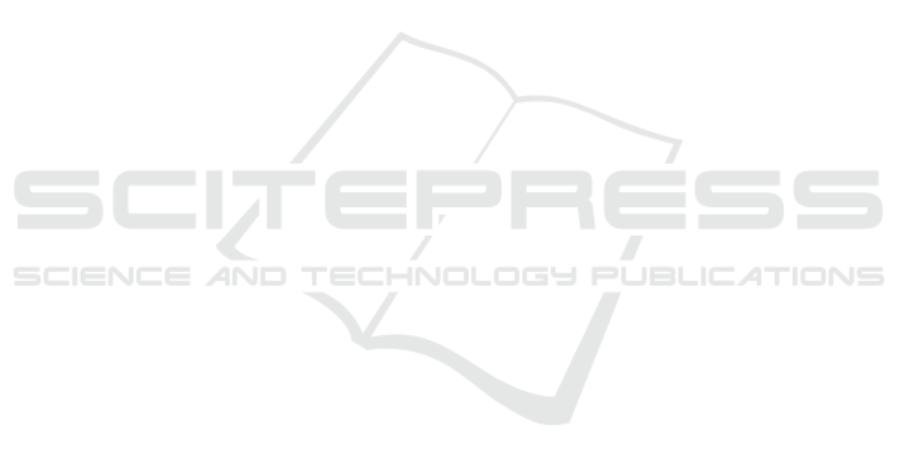
Development of a deep learning algorithm for peri-
apical disease detection in dental radiographs. Diag-
nostics, 10(6):430.
Gan, Y., Xia, Z., Xiong, J., Li, G., and Zhao, Q. (2017).
Tooth and Alveolar Bone Segmentation From Den-
tal Computed Tomography Images. IEEE Journal of
Biomedical and Health Informatics, 22(1):196–204.
He, K., Zhang, X., Ren, S., and Sun, J. (2015). Delving
deep into rectifiers: Surpassing human-level perfor-
mance on ImageNet classification. In Proceedings of
the IEEE International Conference on Computer Vi-
sion, pages 1026–1034.
Heimann, T. and Meinzer, H.-P. (2009). Statistical shape
models for 3D medical image segmentation: A review.
Medical Image Analysis, 13(4):543–563.
Jain, A., Tompson, J., Andriluka, M., Taylor, G. W., and
Bregler, C. (2014). Learning human pose estima-
tion features with convolutional networks. In 2nd In-
ternational Conference on Learning Representations
(ICLR), pages 1–11.
Khanagar, S. B., Al-Ehaideb, A., Vishwanathaiah, S., Ma-
ganur, P. C., Patil, S., Naik, S., Baeshen, H. A., and
Sarode, S. S. (2021). Scope and performance of ar-
tificial intelligence technology in orthodontic diagno-
sis, treatment planning, and clinical decision-making-
a systematic review. Journal of Dental Sciences,
16(1):482–492.
Kirnbauer, B., Hadzic, A., Jakse, N., Bischof, H., and Stern,
D. (2022). Automatic detection of periapical oste-
olytic lesions on cone-beam computed tomography
using deep convolutional neuronal networks. Journal
of Endodontics, 48(11):1434–1440.
Krois, J., Garcia Cantu, A., Chaurasia, A., Patil, R., Chaud-
hari, P. K., Gaudin, R., Gehrung, S., and Schwen-
dicke, F. (2021). Generalizability of deep learning
models for dental image analysis. Scientific Reports,
11(1):6102.
Lee, J.-H., Kim, D.-H., and Jeong, S.-N. (2020). Diagno-
sis of cystic lesions using panoramic and cone beam
computed tomographic images based on deep learn-
ing neural network. Oral Diseases, 26(1):152–158.
Lin, T., Goyal, P., Girshick, R. B., He, K., and Doll
´
ar, P.
(2017). Focal loss for dense object detection. In IEEE
International Conference on Computer Vision, pages
2999–3007. IEEE.
Orhan, K., Bayrakdar, I., Ezhov, M., Kravtsov, A., and
¨
Ozy
¨
urek, T. (2020). Evaluation of artificial intelli-
gence for detecting periapical pathosis on cone-beam
computed tomography scans. International Endodon-
tic Journal, 53(5):680–689.
Pauwels, R., Brasil, D. M., Yamasaki, M. C., Jacobs, R.,
Bosmans, H., Freitas, D. Q., and Haiter-Neto, F.
(2021). Artificial intelligence for detection of periapi-
cal lesions on intraoral radiographs: Comparison be-
tween convolutional neural networks and human ob-
servers. Oral Surgery, Oral Medicine, Oral Pathology
and Oral Radiology, 131(5):610–616.
Payer, C., Stern, D., Bischof, H., and Urschler, M. (2019).
Integrating spatial configuration into heatmap regres-
sion based CNNs for landmark localization. Medical
Image Analysis, 54:207–219.
Payer, C., Stern, D., Bischof, H., and Urschler, M. (2020).
Coarse to fine vertebrae localization and segmentation
with SpatialConfiguration-Net and U-Net. In VISI-
GRAPP (5: VISAPP), pages 124–133.
Ronneberger, O., Fischer, P., and Brox, T. (2015). U-
net: Convolutional networks for biomedical im-
age segmentation. In Medical Image Computing
and Computer-Assisted Intervention – MICCAI 2015,
pages 234–241. Springer International Publishing.
Setzer, F. C., Shi, K. J., Zhang, Z., Yan, H., Yoon, H., Mup-
parapu, M., and Li, J. (2020). Artificial intelligence
for the computer-aided detection of periapical lesions
in cone-beam computed tomographic images. Journal
of Endodontics, 46(7):987–993.
Taghanaki, S. A., Zheng, Y., Zhou, S. K., Georgescu, B.,
Sharma, P., Xu, D., Comaniciu, D., and Hamarneh, G.
(2019). Combo loss: Handling input and output im-
balance in multi-organ segmentation. Computerized
Medical Imaging and Graphics, 75:24–33.
Umer, F. and Habib, S. (2022). Critical analysis of artificial
intelligence in endodontics: a scoping review. Journal
of Endodontics, 48(2):152–160.
Unterpirker, W., Ebner, T.,
ˇ
Stern, D., and Urschler, M.
(2015). Automatic third molar localization from 3D
MRI using random regression forests. In 19th Interna-
tional Conference on Medical Image Understanding
and Analysis (MIUA), pages 195–200, Lincoln, UK.
Urschler, M., Ebner, T., and
ˇ
Stern, D. (2018). Integrating
geometric configuration and appearance information
into a unified framework for anatomical landmark lo-
calization. Medical Image Analysis, 43:23–36.
Urschler, M., Leitinger, G., and Pock, T. (2014). Interac-
tive 2D/3D image denoising and segmentation tool for
medical applications. In Proceedings MICCAI Work-
shop Interactive Medical Image Computation (IMIC).
Yushkevich, P. A., Piven, J., Cody Hazlett, H., Gim-
pel Smith, R., Ho, S., Gee, J. C., and Gerig, G.
(2006). User-guided 3D active contour segmentation
of anatomical structures: Significantly improved effi-
ciency and reliability. Neuroimage, 31(3):1116–1128.
Zhang, J., Liu, M., and Shen, D. (2017). Detecting
anatomical landmarks from limited medical imaging
data using two-stage task-oriented deep neural net-
works. IEEE Transactions on Image Processing,
26(10):4753–4764.
Zheng, Z., Yan, H., Setzer, F. C., Shi, K. J., Mupparapu,
M., and Li, J. (2020). Anatomically constrained deep
learning for automating dental cbct segmentation and
lesion detection. IEEE Transactions on Automation
Science and Engineering, 18(2):603–614.
Teeth Localization and Lesion Segmentation in CBCT Images Using SpatialConfiguration-Net and U-Net
297