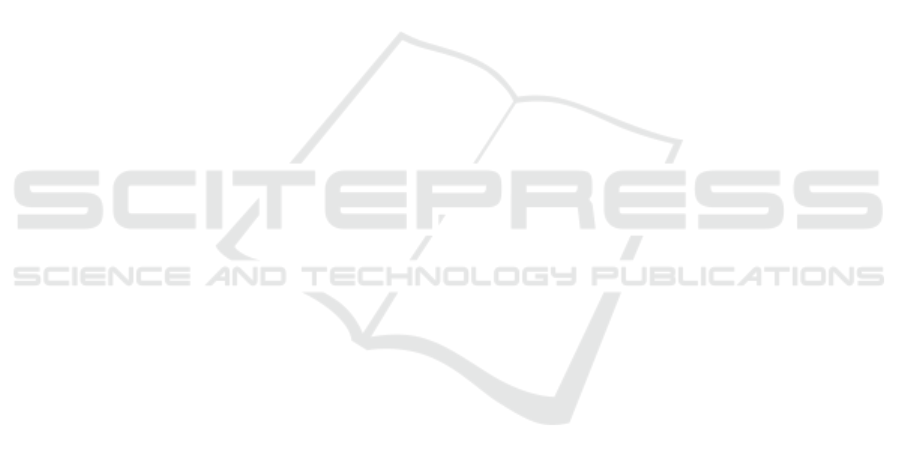
ACKNOWLEDGEMENTS
We would like to thank VLAIO and BASF Antwerpen
for funding the project (HBC.2020.2876).
REFERENCES
Agarwal, M., Singh, A., Arjaria, S., Sinha, A., and Gupta,
S. (2020). ToLeD: Tomato leaf disease detection us-
ing convolution neural network. Procedia Computer
Science, 167:293–301. International Conference on
Computational Intelligence and Data Science.
Bowles, C., Chen, L., Guerrero, R., Bentley, P., Gunn, R.,
Hammers, A., Dickie, D. A., Hern
´
andez, M. V., Ward-
law, J., and Rueckert, D. (2018). GAN augmentation:
Augmenting training data using generative adversarial
networks. arXiv preprint arXiv:1810.10863.
Connor, S. and M., K. T. (2019). A survey on image data
augmentation for deep learning. Journal of Big Data,
6(1):60.
Dawson, H. L., Dubrule, O., and John, C. M. (2023). Impact
of dataset size and convolutional neural network archi-
tecture on transfer learning for carbonate rock classi-
fication. Computers & Geosciences, 171:105284.
Goodfellow, I., Pouget-Abadie, J., Mirza, M., Xu, B.,
Warde-Farley, D., Ozair, S., Courville, A., and Ben-
gio, Y. (2014). Generative adversarial nets. In Ghahra-
mani, Z., Welling, M., Cortes, C., Lawrence, N., and
Weinberger, K., editors, Advances in Neural Infor-
mation Processing Systems, volume 27. Curran Asso-
ciates, Inc.
Hassan, M. R., Islam, M. F., Uddin, M. Z., Ghoshal, G.,
Hassan, M. M., Huda, S., and Fortino, G. (2022).
Prostate cancer classification from ultrasound and
MRI images using deep learning based explainable ar-
tificial intelligence. Future Generation Computer Sys-
tems, 127:462–472.
Kang, L.-W., Wang, I.-S., Chou, K.-L., Chen, S.-Y., and
Chang, C.-Y. (2019). Image-based real-time fire de-
tection using deep learning with data augmentation for
vision-based surveillance applications. In 2019 16th
IEEE International Conference on Advanced Video
and Signal Based Surveillance (AVSS), pages 1–4.
Karras, T., Aittala, M., Hellsten, J., Laine, S., Lehtinen, J.,
and Aila, T. (2020). Training generative adversarial
networks with limited data. In Larochelle, H., Ran-
zato, M., Hadsell, R., Balcan, M., and Lin, H., editors,
Advances in Neural Information Processing Systems,
volume 33, pages 12104–12114. Curran Associates,
Inc.
Kingma, D. P. and Welling, M. (2013). Auto-encoding vari-
ational bayes. arXiv preprint arXiv:1312.6114.
Krizhevsky, A., Sutskever, I., and Hinton, G. E. (2012). Im-
agenet classification with deep convolutional neural
networks. Advances in neural information processing
systems, 25.
Liu, B., Zhu, Y., Song, K., and Elgammal, A. (2020). To-
wards faster and stabilized GAN training for high-
fidelity few-shot image synthesis. In International
Conference on Learning Representations.
Lucic, M., Kurach, K., Michalski, M., Gelly, S., and Bous-
quet, O. (2018). Are GANs created equal? a large-
scale study. Advances in neural information process-
ing systems, 31.
Lundberg, S. M. and Lee, S.-I. (2017). A unified approach
to interpreting model predictions. Advances in neural
information processing systems, 30.
Maharana, K., Mondal, S., and Nemade, B. (2022). A
review: Data pre-processing and data augmentation
techniques. Global Transitions Proceedings, 3(1):91–
99.
Mankodiya, H., Jadav, D., Gupta, R., Tanwar, S., Hong, W.-
C., and Sharma, R. (2022). OD-XAI: Explainable AI-
based semantic object detection for autonomous vehi-
cles. Applied Sciences, 12.
Nazki, H., Lee, J., Yoon, S., and Park, D. S. (2019). Image-
to-image translation with GAN for synthetic data aug-
mentation in plant disease datasets. Smart Media
Journal, 8(2):46–57.
Ribeiro, M. T., Singh, S., and Guestrin, C. (2016). ” why
should i trust you?” explaining the predictions of any
classifier. In Proceedings of the 22nd ACM SIGKDD
international conference on knowledge discovery and
data mining, pages 1135–1144.
Schorr, C., Goodarzi, P., Chen, F., and Dahmen, T. (2021).
Neuroscope: An explainable AI toolbox for seman-
tic segmentation and image classification of convolu-
tional neural nets. Applied Sciences, 11(5):2199.
Stuyck, T., Rousseau, A.-J., Vallerio, M., and Demeester, E.
(2022). Semi-supervised cloud detection with weakly
labeled RGB aerial images using generative adversar-
ial networks. In ICPRAM, pages 630–635.
Taylor, L. and Nitschke, G. (2018). Improving deep
learning with generic data augmentation. In 2018
IEEE symposium series on computational intelligence
(SSCI), pages 1542–1547. IEEE.
Xu, F., Uszkoreit, H., Du, Y., Fan, W., Zhao, D., and Zhu, J.
(2019). Explainable AI: A brief survey on history, re-
search areas, approaches and challenges. In Natural
Language Processing and Chinese Computing: 8th
CCF International Conference, NLPCC 2019, Dun-
huang, China, October 9–14, 2019, Proceedings, Part
II 8, pages 563–574. Springer.
Impact of Using GAN Generated Synthetic Data for the Classification of Chemical Foam in Low Data Availability Environments
627