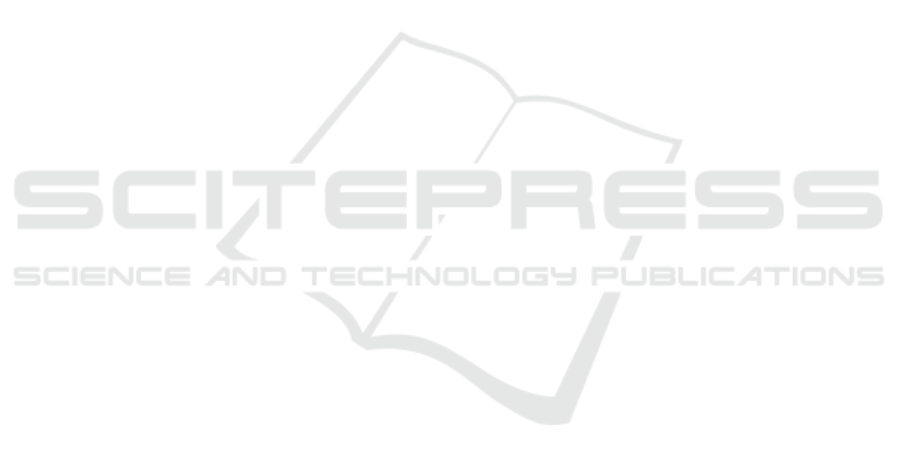
REFERENCES
Al-Azawi, M. A. (2021). Saliency-based image retrieval
as a refinement to content-based image retrieval. EL-
CVIA: Electronic Letters on Computer Vision and Im-
age Analysis, 20(1):0001–15.
”Bel
´
em, F., Guimar
˜
aes, S. J., and F., Falc
˜
ao, A. X.
(2019). Superpixel segmentation by object-based it-
erative spanning forest. In Progress in Pattern Recog-
nition, Image Analysis, Computer Vision, and Appli-
cations, pages 334–341. Springer International Pub-
lishing.
Bel
´
em, F., Melo, L., Guimar
˜
aes, S., and Falc
˜
ao, A. (2019).
The importance of object-based seed sampling for su-
perpixel segmentation. In Proc. 32nd Conf. Graphics
Pattern Images (SIBGRAPI), pages 108–115. To ap-
pear.
Cheng, M., Mitra, N., Huang, X., Torr, P., and Hu, S.
(2014). Global contrast based salient region detection.
IEEE Transactions on Pattern Analysis and Machine
Intelligence, 37(3):569–582.
Falc
˜
ao, A. X., Stolfi, J., and Lotufo, R. A. (2004). The
image foresting transform: Theory, algorithms, and
applications. IEEE Trans. Pattern Anal. Mach. Intell.,
26:19–29.
He, K., Zhang, X., Ren, S., and Sun, J. (2016). Deep resid-
ual learning for image recognition. In Proceedings of
the IEEE conference on computer vision and pattern
recognition, pages 770–778.
He, S., Lau, R. W., Liu, W., Huang, Z., and Yang, Q. (2015).
Supercnn: A superpixelwise convolutional neural net-
work for salient object detection. International jour-
nal of computer vision, 115(3):330–344.
Huang, G., Liu, Z., Van Der Maaten, L., and Weinberger, K.
(2017). Densely connected convolutional networks.
In Proceedings of the IEEE conference on computer
vision and pattern recognition, pages 4700–4708.
Iqbal, E., Niaz, A., Memon, A., Asim, U., and Choi, K.
(2020). Saliency-driven active contour model for im-
age segmentation. IEEE Access, 8:208978–208991.
Jiang, P., Ling, H., Yu, J., and Peng, J. (2013). Salient
region detection by ufo: Uniqueness, focusness and
objectness. In Proceedings of the IEEE international
conference on computer vision, pages 1976–1983.
Li, L., Chai, X., Zhao, S., Zheng, S., and Su, S.
(2018). Saliency optimization and integration via
iterative bootstrap learning. International Journal
of Pattern Recognition and Artificial Intelligence,
32(09):1859016.
Liu, J., Hou, Q., Cheng, M., Feng, J., and Jiang, J. (2019). A
simple pooling-based design for real-time salient ob-
ject detection. In Proceedings of the IEEE/CVF Con-
ference on Computer Vision and Pattern Recognition,
pages 3917–3926.
Liu, N. and Han, J. (2016). Dhsnet: Deep hierarchical
saliency network for salient object detection. In Pro-
ceedings of the IEEE conference on computer vision
and pattern recognition, pages 678–686.
Luo, Z., Mishra, A., Achkar, A., Eichel, J., Li, S., and
Jodoin, P. (2017). Non-local deep features for salient
object detection. In Proceedings of the IEEE Con-
ference on computer vision and pattern recognition,
pages 6609–6617.
Miao, Z., Tingwei, L., Yongri, P., ShunYu, Y., and Huchuan,
L. (2021). Auto-msfnet: Search multi-scale fusion
network for salient object detection. In ACM Multi-
media Conference 2021, page 667–676.
Peng, H., Li, B., Ling, H., Hu, W., Xiong, W., and May-
bank, S. (2016). Salient object detection via structured
matrix decomposition. IEEE transactions on pattern
analysis and machine intelligence, 39(4):818–832.
Qin, X., Zhang, Z., Huang, C., Dehghan, M., Zaiane, O. R.,
and Jagersand, M. (2020). U2-net: Going deeper with
nested u-structure for salient object detection. Pattern
Recognition, 106:107404.
Qin, X., Zhang, Z., Huang, C., Gao, C., Dehghan, M.,
and Jagersand, M. (2019). Basnet: Boundary-
aware salient object detection. In Proceedings of the
IEEE/CVF Conference on Computer Vision and Pat-
tern Recognition, pages 7479–7489.
Qin, Y., Lu, H., Xu, Y., and Wang, H. (2015). Saliency
detection via cellular automata. In Proceedings of
the IEEE Conference on Computer Vision and Pattern
Recognition, pages 110–119.
Singh, V. K. and Kumar, N. (2020). Saliency bagging: a
novel framework for robust salient object detection.
The Visual Computer, 36(7):1423–1441.
Vargas-Mu
˜
noz, J. E., Chowdhury, A., Alexandre, E.,
Galv
˜
ao, F., Miranda, P., and Falc
˜
ao, A. (2019). An it-
erative spanning forest framework for superpixel seg-
mentation. IEEE Transactions on Image Processing,
28(7):3477–3489.
Wang, J., de Melo Joao, L., Falc
˜
ao, A., Kosinka, J., and
Telea, A. (2021a). Focus-and-context skeleton-based
image simplification using saliency maps. In VISI-
GRAPP (4: VISAPP), pages 45–55.
Wang, W., Lai, Q., Fu, H., Shen, J., Ling, H., and Yang,
R. (2021b). Salient object detection in the deep learn-
ing era: An in-depth survey. IEEE Transactions on
Pattern Analysis and Machine Intelligence.
Yang, C., Zhang, L., Lu, H., Ruan, X., and Yang, M. (2013).
Saliency detection via graph-based manifold ranking.
In Computer Vision and Pattern Recognition (CVPR),
2013 IEEE Conference on, pages 3166–3173. IEEE.
Zhang, P., Wang, D., Lu, H., Wang, H., and Ruan, X.
(2017). Amulet: Aggregating multi-level convolu-
tional features for salient object detection. In Proceed-
ings of the IEEE International Conference on Com-
puter Vision, pages 202–211.
Zhao, J., Liu, J., Fan, D., Cao, Y., Yang, J., and Cheng,
M. (2019). Egnet: Edge guidance network for salient
object detection. In Proceedings of the IEEE/CVF
International Conference on Computer Vision, pages
8779–8788.
VISAPP 2024 - 19th International Conference on Computer Vision Theory and Applications
308