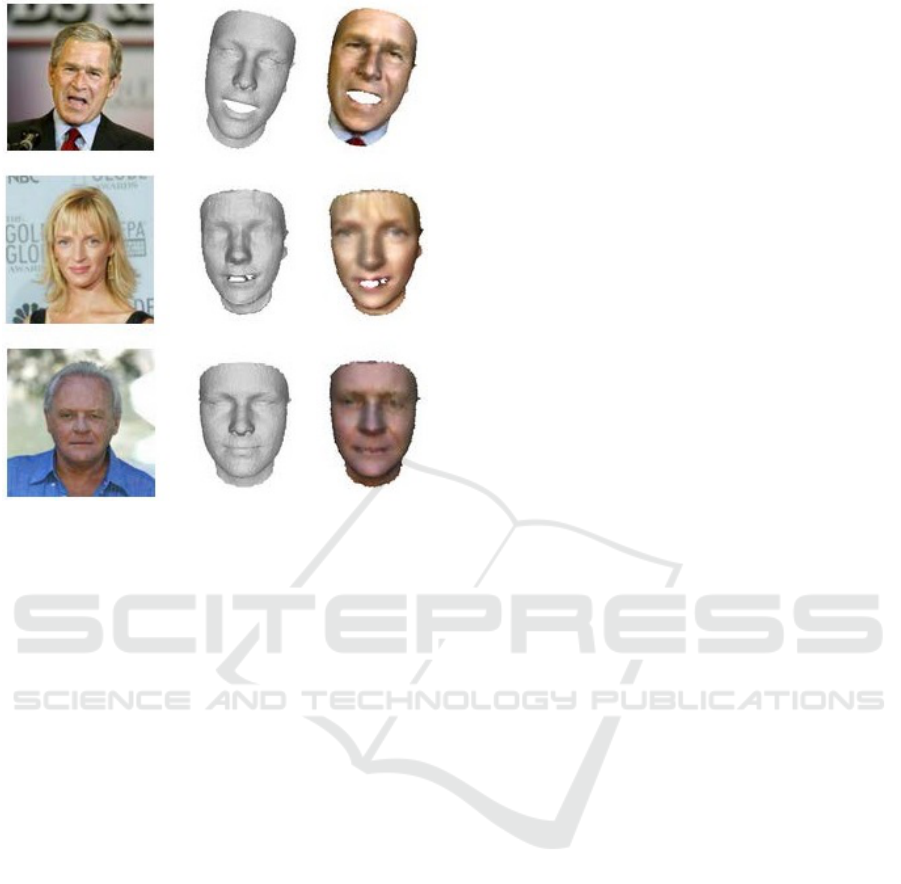
Figure 3: Original images (first column), generated shapes
(second column), and refined final output (third column) ob-
tained from the proposed pipeline.
could be assumed. The method seems particularly
suitable to provide appreciable results while avoiding
heavyweight models, e.g., Transformers (Basak et al.,
2022). Also, more powerful hardware could be ex-
ploited for testing the method with different hyperpa-
rameters and deeper architectures. Furthermore, the
details filling phase could be improved by capturing
finer texture details in the final geometry to provide
a more realistic face model. In conclusion, a com-
parative analysis will be conducted in the future: ex-
ploiting the same procedure to generate the custom
dataset, we will collect results after running different
well-known approaches on it and compare them with
the proposed solution’s ones.
ACKNOWLEDGEMENTS
This work was supported by the “Smart un-
mannEd AeRial vehiCles for Human likE moni-
toRing (SEARCHER)” project of the Italian Min-
istry of Defence (CIG: Z84333EA0D) and the re-
search leading to these results has received funding
from Project “Ecosistema dell’innovazione - Rome
Technopole” financed by EU in NextGenerationEU
plan through MUR Decree n. 1051 23.06.2022 - CUP
H33C22000420001.
REFERENCES
Avola, D., Cascio, M., Cinque, L., Fagioli, A., Foresti,
G. L., Marini, M. R., and Rossi, F. (2022). Real-
time deep learning method for automated detection
and localization of structural defects in manufac-
tured products. Computers & Industrial Engineering,
172:108512.
Avola, D., Cinque, L., Foresti, G. L., Lanzino, R., Marini,
M. R., Mecca, A., and Scarcello, F. (2023). A
novel transformer-based imu self-calibration approach
through on-board rgb camera for uav flight stabiliza-
tion. Sensors, 23(5).
Avola, D., Cinque, L., Foresti, G. L., and Marini, M. R.
(2019). An interactive and low-cost full body reha-
bilitation framework based on 3d immersive serious
games. Journal of Biomedical Informatics, 89:81–
100.
Badri, H., El Hassouni, M., and Aboutajdine, D. (2012).
Kernel-based laplacian smoothing method for 3d
mesh denoising. In Image and Signal Processing: 5th
International Conference, ICISP 2012, Agadir, Mo-
rocco, June 28-30, 2012. Proceedings 5, pages 77–84.
Springer.
Basak, S., Corcoran, P., McDonnell, R., and Schukat, M.
(2022). 3d face-model reconstruction from a single
image: A feature aggregation approach using hierar-
chical transformer with weak supervision. Neural Net-
works, 156:108–122.
Beeler, T., Bickel, B., Beardsley, P., Sumner, B., and Gross,
M. (2010). High-quality single-shot capture of facial
geometry. In ACM SIGGRAPH 2010 papers, pages
1–9.
Borji, A. (2018). Pros and cons of gan evaluation measures.
Demir, U. and Unal, G. (2018). Patch-based image in-
painting with generative adversarial networks. arXiv
preprint arXiv:1803.07422.
Goodfellow, I. J., Pouget-Abadie, J., Mirza, M., Xu, B.,
Warde-Farley, D., Ozair, S., Courville, A., and Ben-
gio, Y. (2014). Generative adversarial networks.
Huang, G. B., Mattar, M., Berg, T., and Learned-Miller,
E. (2008). Labeled faces in the wild: A database
forstudying face recognition in unconstrained envi-
ronments. In Workshop on faces in’Real-Life’Images:
detection, alignment, and recognition.
Isola, P., Zhu, J.-Y., Zhou, T., and Efros, A. A. (2017).
Image-to-image translation with conditional adversar-
ial networks. In Proceedings of the IEEE conference
on computer vision and pattern recognition, pages
1125–1134.
Jiang, D., Jin, Y., Zhang, F.-L., Zhu, Z., Zhang, Y., Tong, R.,
and Tang, M. (2023). Sphere face model: A 3d mor-
phable model with hypersphere manifold latent space
using joint 2d/3d training. Computational Visual Me-
dia, 9(2):279–296.
Joseph, V. R. (2022). Optimal ratio for data splitting. Sta-
tistical Analysis and Data Mining: The ASA Data Sci-
ence Journal, 15(4):531–538.
Kuang, H., Ding, Y., Ma, X., and Liu, X. (2019). 3d face re-
construction with texture details from a single image
FaceVision-GAN: A 3D Model Face Reconstruction Method from a Single Image Using GANs
631