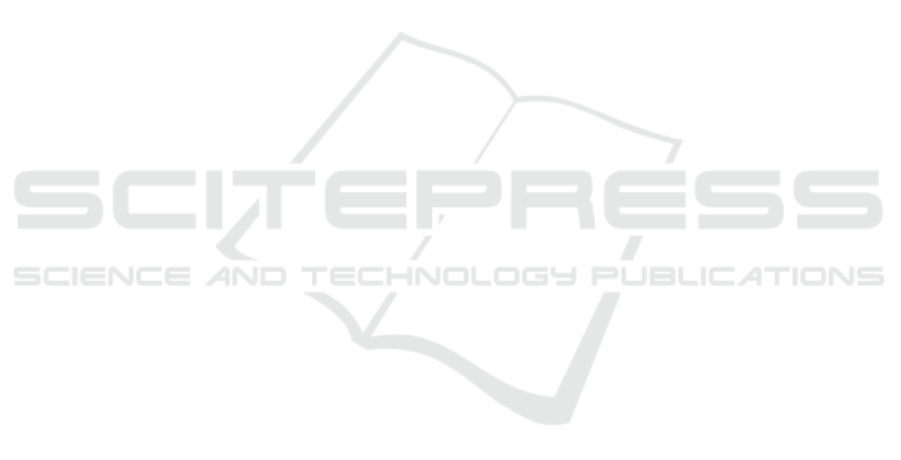
rection for future work, further refinements and opti-
mization of the framework can be explored alongside
more sophisticated travel cost estimation based on
robot motion profiles, integration of obstacle avoid-
ance modules, and more use-case experiments. More-
over, investigations into end-to-end approaches of hu-
man intention forecasting for robot task sequencing
may prove to be effective.
REFERENCES
Ajoudani, A., Zanchettin, A. M., Ivaldi, S., Albu-Sch
¨
affer,
A., Kosuge, K., and Khatib, O. (2018). Progress
and prospects of the human–robot collaboration. Au-
tonomous Robots, 42:957–975.
Alatartsev, S., Belov, A., Nykolaychuk, M., and Ortmeier,
F. (2014). Robot trajectory optimization for the re-
laxed end-effector path. In 2014 11th Int. Conf.
on Informatics in Control, Automation and Robotics
(ICINCO), volume 1, pages 385–390. IEEE.
Alatartsev, S., Stellmacher, S., and Ortmeier, F. (2015).
Robotic task sequencing problem: A survey. Journal
of intelligent & robotic systems, 80:279–298.
Ata, A. A. (2007). Optimal trajectory planning of manipu-
lators: a review. Journal of Engineering Science and
technology, 2(1):32–54.
Chen, G., Xing, S., Chen, Z., Wang, Y., Li, K., Li, Y., Liu,
Y., Wang, J., Zheng, Y.-D., Huang, B., et al. (2022).
Internvideo-ego4d: A pack of champion solutions to
ego4d challenges. arXiv preprint arXiv:2211.09529.
Cheng, Y. and Tomizuka, M. (2021). Long-term trajectory
prediction of the human hand and duration estimation
of the human action. IEEE Robotics and Automation
Letters, 7(1):247–254.
Da Silva, R. F. and Urrutia, S. (2010). A general vns heuris-
tic for the traveling salesman problem with time win-
dows. Discrete Optimization, 7(4):203–211.
Dubowsky, S. and Blubaugh, T. (1989). Planning time-
optimal robotic manipulator motions and work places
for point-to-point tasks. IEEE Transactions on
Robotics and Automation, 5(3):377–381.
Edan, Y., Flash, T., Peiper, U. M., Shmulevich, I., and
Sarig, Y. (1991). Near-minimum-time task planning
for fruit-picking robots. IEEE transactions on robotics
and automation, 7(1):48–56.
Fan, C., Lee, J., and Ryoo, M. S. (2018). Forecasting hands
and objects in future frames. In Proc. of the European
Conf. on Computer Vision (ECCV) Workshops.
Fan, J., Zheng, P., and Li, S. (2022). Vision-based holistic
scene understanding towards proactive human–robot
collaboration. Robotics and Computer-Integrated
Manufacturing, 75.
Grauman, K., Westbury, A., Byrne, E., Chavis, Z., Furnari,
A., Girdhar, R., Hamburger, J., Jiang, H., Liu, M., Liu,
X., et al. (2022). Ego4d: Around the world in 3,000
hours of egocentric video. In Proc. of the IEEE/CVF
Conf. on Computer Vision and Pattern Recognition,
pages 18995–19012.
Kolakowska, E., Smith, S. F., and Kristiansen, M. (2014).
Constraint optimization model of a scheduling prob-
lem for a robotic arm in automatic systems. Robotics
and Autonomous Systems, 62(2):267–280.
Landi, C. T., Cheng, Y., Ferraguti, F., Bonf
`
e, M., Sec-
chi, C., and Tomizuka, M. (2019). Prediction of hu-
man arm target for robot reaching movements. In
2019 IEEE/RSJ International Conference on Intelli-
gent Robots and Systems (IROS), pages 5950–5957.
Li, K., Wang, Y., Gao, P., Song, G., Liu, Y., Li, H., and
Qiao, Y. (2022). Uniformer: Unified transformer for
efficient spatiotemporal representation learning. arXiv
preprint arXiv:2201.04676.
Li, S., Han, K., Li, X., Zhang, S., Xiong, Y., and Xie, Z.
(2021). Hybrid trajectory replanning-based dynamic
obstacle avoidance for physical human-robot interac-
tion. Journal of Intelligent & Robotic Systems, 103:1–
14.
Li, S., Zheng, P., Liu, S., Wang, Z., Wang, X. V., Zheng,
L., and Wang, L. (2023). Proactive human–robot col-
laboration: Mutual-cognitive, predictable, and self-
organising perspectives. Robotics and Computer-
Integrated Manufacturing, 81:102510.
Liu, M., Tang, S., Li, Y., and Rehg, J. M. (2020). Fore-
casting human-object interaction: joint prediction of
motor attention and actions in first person video. In
Computer Vision–ECCV 2020, pages 704–721.
Liu, S., Tripathi, S., Majumdar, S., and Wang, X. (2022).
Joint hand motion and interaction hotspots prediction
from egocentric videos. In Proc. of the IEEE/CVF
Conf. on Computer Vision and Pattern Recognition,
pages 3282–3292.
Lyu, J., Ruppel, P., Hendrich, N., Li, S., G
¨
orner, M., and
Zhang, J. (2022). Efficient and collision-free human-
robot collaboration based on intention and trajectory
prediction. IEEE Trans. on Cognitive and Develop-
mental Systems.
Mainprice, J. and Berenson, D. (2013). Human-robot col-
laborative manipulation planning using early predic-
tion of human motion. In 2013 IEEE/RSJ Interna-
tional Conference on Intelligent Robots and Systems,
pages 299–306. IEEE.
Matheson, E., Minto, R., Zampieri, E. G., Faccio, M.,
and Rosati, G. (2019). Human–robot collaboration
in manufacturing applications: A review. Robotics,
8(4):100.
Mukherjee, D., Gupta, K., Chang, L. H., and Najjaran,
H. (2022). A survey of robot learning strategies
for human-robot collaboration in industrial settings.
Robotics and Computer-Integrated Manufacturing,
73:102231.
Vu, D. M., Hewitt, M., Boland, N., and Savelsbergh, M.
(2020). Dynamic discretization discovery for solving
the time-dependent traveling salesman problem with
time windows. Transportation science, 54(3):703–
720.
Zacharia, P. T. and Aspragathos, N. (2005). Optimal robot
task scheduling based on genetic algorithms. Robotics
and Computer-Integrated Manufacturing, 21(1):67–
79.
Augmenting Human-Robot Collaboration Task by Human Hand Position Forecasting
269