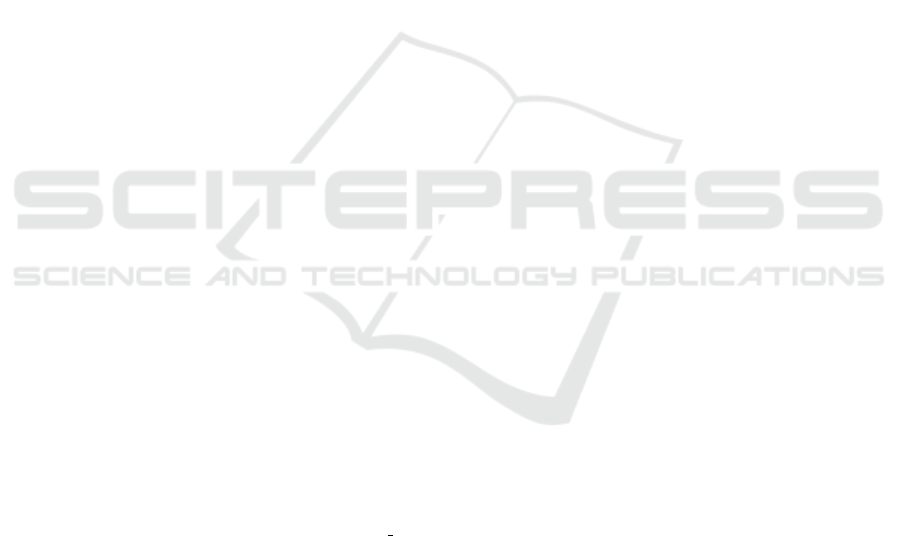
6 CONCLUSION
This paper proposed the novel method to identify
an opinion of a reviewer and its ground in a cus-
tomer review. First, we newly defined the opinion-
ground classification task that aimed to classify a pair
of clauses whether it contained an opinion and its
ground. To train the classifiers of this task, three het-
erogeneous datasets were constructed: (1) the part of
KWDLC that consisted of pairs of clauses under the
“cause/reason” relation as the positive samples, (2)
the dataset constructed by checking the existence of
the discourse markers, and (3) the augmented dataset
including the clauses generated by ChatGPT. In ad-
dition, the rule-based method, BERT, and the hybrid
of these two methods were emprically compared as
the classification models. Results of the experiments
showed that the use of not only the existing manu-
ally annotated out-domain dataset (KWDLC) but also
the automatically constructed in-domain dataset could
improve the F1-score of the opinion-ground classifi-
cation task. The best F1-score, 0.71, was obtained
when the BERT model was trained by the interme-
diate fine-tuning using three datatsets. It was 0.12
points higher than the model using only KWDLC.
In the future, the in-domain datasets should be en-
larged so that the classifier can trained from a corpus
including a wide variety of linguistic expressions that
represent the opinion-ground relation. In addition, in-
stead of just checking two causality discourse mark-
ers, we will investigate a more sophisticated rule-
based method to extract the opinion-ground clause
pairs from unlabeled reviews more precisely.
REFERENCES
Almagrabi, H., Malibari, A., and McNaught, J. (2018). Cor-
pus analysis and annotation for helpful sentences in
product reviews. Computer and Information Science,
11(2):76–87.
Cengiz, C., Sert, U., and Yuret, D. (2019). KU ai at
MEDIQA 2019: Domain-specific pre-training and
transfer learning for medical NLI. In Proceedings of
the 18th BioNLP Workshop and Shared Task, pages
427–436, Florence, Italy. Association for Computa-
tional Linguistics.
Dai, H., Liu, Z., Liao, W., Huang, X., Cao, Y., Wu, Z.,
Zhao, L., Xu, S., Liu, W., Liu, N., et al. (2023). Aug-
GPT: Leveraging ChatGPT for text data augmenta-
tion. arXiv preprint arXiv:2302.13007.
Devlin, J., Chang, M.-W., Lee, K., and Toutanova, K.
(2019). BERT: Pre-training of deep bidirectional
transformers for language understanding. In Pro-
ceedings of the 2019 Conference of the North Amer-
ican Chapter of the Association for Computational
Linguistics: Human Language Technologies, Volume
1 (Long and Short Papers), pages 4171–4186, Min-
neapolis, Minnesota. Association for Computational
Linguistics.
Diaz, G. O. and Ng, V. (2018). Modeling and prediction of
online product review helpfulness: A survey. In Pro-
ceedings of the 56th Annual Meeting of the Associa-
tion for Computational Linguistics (Volume 1: Long
Papers), pages 698–708, Melbourne, Australia. Asso-
ciation for Computational Linguistics.
Gamzu, I., Gonen, H., Kutiel, G., Levy, R., and Agichtein,
E. (2021). Identifying helpful sentences in product re-
views. In Proceedings of the 2021 Conference of the
North American Chapter of the Association for Com-
putational Linguistics: Human Language Technolo-
gies, pages 678–691, Online. Association for Compu-
tational Linguistics.
Gilardi, F., Alizadeh, M., and Kubli, M. (2023). Chatgpt
outperforms crowd-workers for text-annotation tasks.
arXiv preprint arXiv:2303.15056.
Hu, M. and Liu, B. (2004). Mining and summarizing
customer reviews. In Proceedings of the tenth ACM
SIGKDD international conference on Knowledge dis-
covery and data mining, pages 168–177.
Kim, N., Feng, S., Gunasekara, C., and Lastras, L. (2020).
Implicit discourse relation classification: We need to
talk about evaluation. In Proceedings of the 58th An-
nual Meeting of the Association for Computational
Linguistics, pages 5404–5414, Online. Association
for Computational Linguistics.
Kim, S.-M., Pantel, P., Chklovski, T., and Pennacchiotti, M.
(2006). Automatically assessing review helpfulness.
In Proceedings of the 2006 Conference on Empiri-
cal Methods in Natural Language Processing, pages
423–430, Sydney, Australia. Association for Compu-
tational Linguistics.
Kishimoto, Y., Murawaki, Y., Kawahara, D., and Kuro-
hashi, S. (2020). Japanese discourse relation analysis:
Task definition, connective detection, and corpus an-
notation (in Japanese). Journal of Natural Language
Processing, 27(4):899–931.
Liu, J., Cao, Y., Lin, C.-Y., Huang, Y., and Zhou, M. (2007).
Low-quality product review detection in opinion sum-
marization. In Proceedings of the 2007 Joint Confer-
ence on Empirical Methods in Natural Language Pro-
cessing and Computational Natural Language Learn-
ing (EMNLP-CoNLL), pages 334–342, Prague, Czech
Republic. Association for Computational Linguistics.
Loshchilov, I. and Hutter, F. (2019). Decoupled weight
decay regularization. In International Conference on
Learning Representations.
Mudambi, S. M. and Schuff, D. (2010). Research note:
What makes a helpful online review? a study of cus-
tomer reviews on amazon.com. MIS quarterly, pages
185–200.
Pan, Y. and Zhang, J. Q. (2011). Born unequal: a study
of the helpfulness of user-generated product reviews.
Journal of retailing, 87(4):598–612.
Poth, C., Pfeiffer, J., R
¨
uckl
´
e, A., and Gurevych, I. (2021).
What to pre-train on? Efficient intermediate task se-
lection. In Proceedings of the 2021 Conference on
Identification of Opinion and Ground in Customer Review Using Heterogeneous Datasets
77