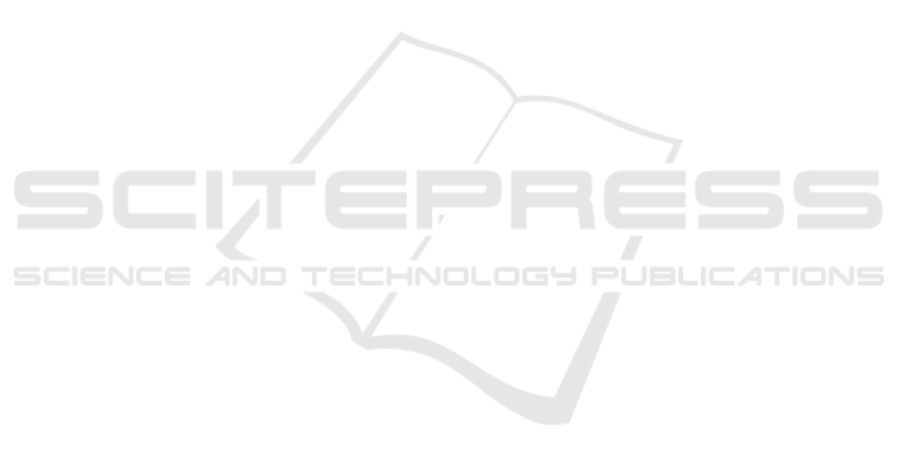
ACKNOWLEDGEMENTS
This work has received funding from Basque Gov-
ernment under project AutoTrust of the program
ELKARTEK-2023.
REFERENCES
Chen, Y., Zhang, L., Shen, Y., Zhao, B. N., and Zhou, Y.
(2022). Extrinsic self-calibration of the surround-view
system: A weakly supervised approach. IEEE Trans-
actions on Multimedia.
Deng, J., Dong, W., Socher, R., Li, L.-J., Li, K., and Fei-
Fei, L. (2009). Imagenet: A large-scale hierarchical
image database. In 2009 IEEE conference on com-
puter vision and pattern recognition, pages 248–255.
Ieee.
Georgiev, M., Boev, A., Gotchev, A., and Hannuksela, M.
(2010). Influence of camera parameters on the qual-
ity of mobile 3d capture. In Multimedia on Mobile
Devices 2010, volume 7542, pages 246–257. SPIE.
He, D., Zou, Z., Chen, Y., Liu, B., Yao, X., and Shan, S.
(2021). Obstacle detection of rail transit based on deep
learning. Measurement, 176:109241.
He, K., Zhang, X., Ren, S., and Sun, J. (2016). Deep resid-
ual learning for image recognition. In Proceedings of
the IEEE conference on computer vision and pattern
recognition, pages 770–778.
Heng, L., Choi, B., Cui, Z., Geppert, M., Hu, S., Kuan,
B., Liu, P., Nguyen, R., Yeo, Y. C., Geiger, A., et al.
(2019). Project autovision: Localization and 3d scene
perception for an autonomous vehicle with a multi-
camera system. In 2019 International Conference on
Robotics and Automation (ICRA), pages 4695–4702.
IEEE.
I
˜
niguez de Gordoa, J. A., Garc
´
ıa, S., P
´
erez, L., Urbieta,
I., Aranjuelo, N., Nieto, M., and Ochoa, D. (2023).
Scenario-based validation of automated train systems
using a 3d virtual railway environment. in press.
Liu, X., Zhang, L., Shen, Y., Zhang, S., and Zhao, S. (2019).
Online camera pose optimization for the surround-
view system. In Proceedings of the 27th ACM Inter-
national Conference on Multimedia, pages 383–391.
S
´
anchez, M., I
˜
niguez de Gordoa, J. A., Nieto, M., and Car-
balleira, P. (2023). Method for the automatic measure-
ment of camera-calibration quality in a surround-view
system. In Fifteenth International Conference on Ma-
chine Vision (ICMV 2022), volume 12701, pages 428–
436. SPIE.
Wu, D., Liao, M.-W., Zhang, W.-T., Wang, X.-G., Bai, X.,
Cheng, W.-Q., and Liu, W.-Y. (2022). Yolop: You
only look once for panoptic driving perception. Ma-
chine Intelligence Research, 19(6):550–562.
Yanan, S., Hui, Z., Li, L., and Hang, Z. (2018). Rail surface
defect detection method based on yolov3 deep learn-
ing networks. In 2018 chinese automation congress
(CAC), pages 1563–1568. IEEE.
Yin, J., Tang, T., Yang, L., Xun, J., Huang, Y., and Gao,
Z. (2017). Research and development of automatic
train operation for railway transportation systems: A
survey. Transportation Research Part C: Emerging
Technologies, 85:548–572.
Zhang, T., Zhang, L., Shen, Y., Ma, Y., Zhao, S., and Zhou,
Y. (2020). Oecs: Towards online extrinsics correction
for the surround-view system. In 2020 IEEE Interna-
tional Conference on Multimedia and Expo (ICME),
pages 1–6. IEEE.
Zhang, T., Zhao, N., Shen, Y., Shao, X., Zhang, L., and
Zhou, Y. (2021). Roecs: A robust semi-direct pipeline
towards online extrinsics correction of the surround-
view system. In Proceedings of the 29th ACM Interna-
tional Conference on Multimedia, pages 3153–3161.
VISAPP 2024 - 19th International Conference on Computer Vision Theory and Applications
324