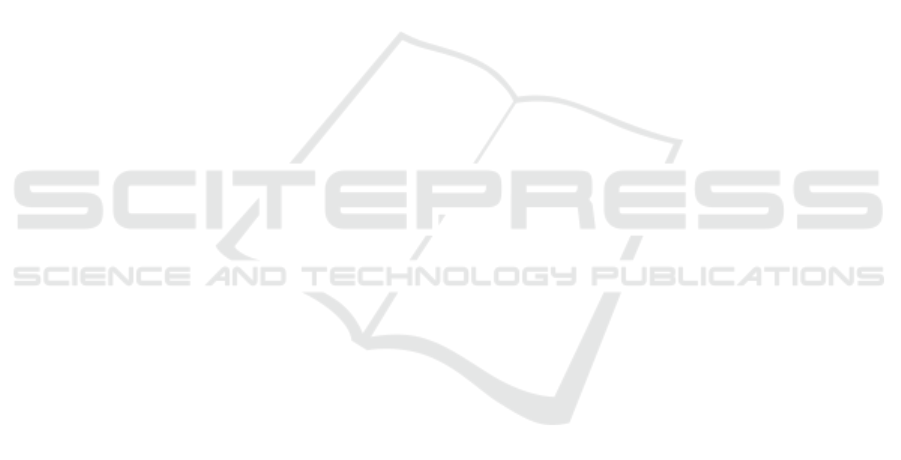
vehicle camera. Therefore, it is expected that the ac-
curacy will be further improved by taking skeletal in-
formation into account in the PEGO Transformer. We
plan to extend the dataset to improve the accuracy of
the proposed method.
ACKNOWLEDGMENT
This work was partially supported by JSPS Grant-in-
Aid for Scientific Research 23H03474. The computa-
tion was carried out using the General Projects on su-
percomputer “Flow” at Information Technology Cen-
ter, Nagoya University.
REFERENCES
Belkada, Y., Bertoni, L., Caristan, R., Mordan, T., and
Alahi, A. (2021). Do pedestrians pay attention? eye
contact detection in the wild.
Caesar, H., Bankiti, V., Lang, A. H., Vora, S., Liong, V. E.,
Xu, Q., Krishnan, A., Pan, Y., Baldan, G., and Bei-
jbom, O. (2020). nuScenes: A multimodal dataset
for autonomous driving. In Proceedings of the 2020
IEEE/CVF Conference on Computer Vision and Pat-
tern Recognition, pages 11618–11628.
Carion, N., Massa, F., Synnaeve, G., Usunier, N., Kirillov,
A., and Zagoruyko, S. (2020). End-to-End object de-
tection with transformers. In In Proceedings of the
European conference on computer vision, pages 213–
229. Springer.
Cordts, M., Omran, M., Ramos, S., Rehfeld, T., Enzweiler,
M., Benenson, R., Franke, U., Roth, S., and Schiele,
B. (2016). The Cityscapes Dataset for Semantic Ur-
ban Scene Understanding. In In Proceedings of the
IEEE/CVF Conference on Computer Vision and Pat-
tern Recognition, pages 3213–3223.
Geiger, A., Lenz, P., Stiller, C., and Urtasun, R. (2013).
Vision meets robotics: The kitti dataset. Interna-
tional Journal of Robotics Research (IJRR), page
1231–1237.
Hata, R., Deguchi, D., Hirayama, T., Kawanishi, Y., and
Murase, H. (2022). Detection of distant eye-contact
using spatio-temporal pedestrian skeletons. In Pro-
ceedings of the IEEE 25th International Conference
on Intelligent Transportation Systems, pages 2730–
2737.
He, K., Zhang, X., Ren, S., and Sun, J. (2016). Deep resid-
ual learning for image recognition. In In Proceedings
of the 2016 IEEE Conference on Computer Vision and
Pattern Recognition, pages 770–778.
Rasouli, A., Kotseruba, I., Kunic, T., and Tsotsos, J. (2019).
PIE: A large-scale dataset and models for pedestrian
intention estimation and trajectory prediction. In Pro-
ceedings of the 2019 IEEE/CVF International Confer-
ence on Computer Vision, pages 6261–6270.
Recasens, A., Khosla, A., Vondrick, C., and Torralba, A.
(2015). Where are they looking? In Proceedings of
the Advances in Neural Information Processing Sys-
tems, volume 28, pages 199–207.
Sun, P., Kretzschmar, H., Dotiwalla, X., Chouard, A., Pat-
naik, V., Tsui, P., Guo, J., Zhou, Y., Chai, Y., Caine,
B., Vasudevan, V., Han, W., Ngiam, J., Zhao, H., Tim-
ofeev, A., Ettinger, S., Krivokon, M., Gao, A., Joshi,
A., Zhang, Y., Shlens, J., Chen, Z., and Anguelov,
D. (2020). Scalability in perception for autonomous
driving: Waymo Open Dataset. In Proceedings of the
2020 IEEE/CVF Conference on Computer Vision and
Pattern Recognition, pages 2443–2451.
Tomas, H., Reyes, M., Dionido, R., Ty, M., Mirando, J.,
Casimiro, J., Atienza, R., and Guinto, R. (2021).
GOO: A dataset for gaze object prediction in retail
environments. In Proceedings of the 2021 IEEE/CVF
Conference on Computer Vision and Pattern Recogni-
tion Workshops, pages 3119–3127.
Tu, D., Min, X., Duan, H., Guo, G., Zhai, G., and Shen, W.
(2022). End-to-End Human-Gaze-Target Detection
with Transformers. In Proceedings of the IEEE/CVF
Conference on Computer Vision and Pattern Recogni-
tion, pages 2192–2200.
Tu, D., Shen, W., Sun, W., Min, X., Zhai, G., and Chen,
C. (2023). Un-gaze: a unified transformer for joint
gaze-location and gaze-object detection. IEEE Trans-
actions on Circuits and Systems for Video Technology.
Vaswani, A., Shazeer, N., Parmar, N., Uszkoreit, J., Jones,
L., Gomez, A. N., Kaiser, L. u., and Polosukhin, I.
(2017). Attention is All you Need. In Proceedings of
the 2017 Advances in Neural Information Processing
Systems, volume 30.
Wang, B., Hu, T., Li, B., Chen, X., and Zhang, Z. (2022).
GaTector: A unified framework for gaze object pre-
diction. In Proceedings of the 2022 IEEE/CVF Con-
ference on Computer Vision and Pattern Recognition,
pages 19588–19597.
Zhu, X., Su, W., Lu, L., Li, B., Wang, X., and Dai, J. (2021).
Deformable DETR: Deformable transformers for end-
to-end object detection. In Proceedings of the 9th In-
ternational Conference on Learning Representations.
VISAPP 2024 - 19th International Conference on Computer Vision Theory and Applications
340