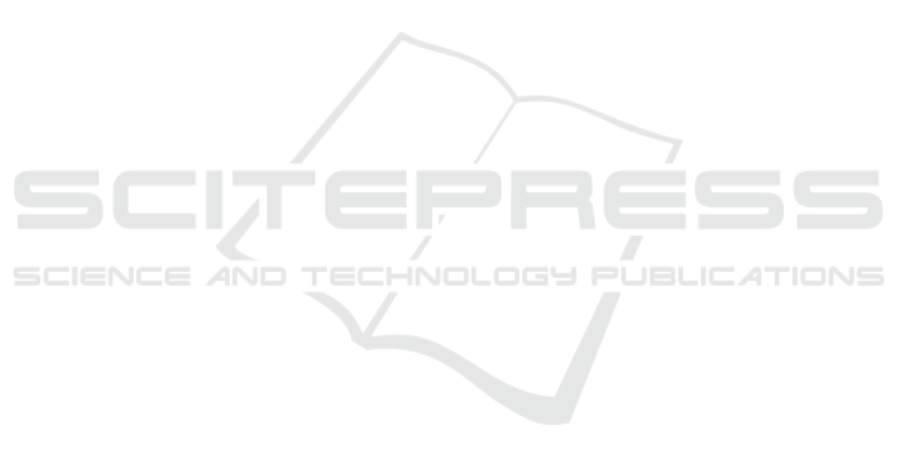
REFERENCES
Bai, X., Hu, Z., Zhu, X., Huang, Q., Chen, Y., Fu, H., and
Tai, C.-L. (2022). Transfusion: Robust lidar-camera
fusion for 3d object detection with transformers. In
IEEE/CVF conference on computer vision and pattern
recognition.
Caesar, H., Bankiti, V., Lang, A. H., Vora, S., Liong, V. E.,
Xu, Q., Krishnan, A., Pan, Y., Baldan, G., and Bei-
jbom, O. (2020). nuscenes: A multimodal dataset
for autonomous driving. In IEEE/CVF conference on
computer vision and pattern recognition.
Carion, N., Massa, F., Synnaeve, G., Usunier, N., Kirillov,
A., and Zagoruyko, S. (2020). End-to-end object de-
tection with transformers. In European conference on
computer vision. Springer.
Chen, Y., Yu, Z., Chen, Y., Lan, S., Anandkumar, A., Jia, J.,
and Alvarez, J. M. (2023). Focalformer3d: Focusing
on hard instance for 3d object detection. In IEEE/CVF
International Conference on Computer Vision.
Chitta, K., Prakash, A., Jaeger, B., Yu, Z., Renz, K.,
and Geiger, A. (2023). Transfuser: Imitation with
transformer-based sensor fusion for autonomous driv-
ing. IEEE Transactions on Pattern Analysis & Ma-
chine Intelligence, 45(11).
Duan, K., Bai, S., Xie, L., Qi, H., Huang, Q., and Tian, Q.
(2019). Centernet: Keypoint triplets for object detec-
tion. In IEEE/CVF international conference on com-
puter vision.
F
¨
urst, M., Wasenm
¨
uller, O., and Stricker, D. (2020). Lrpd:
Long range 3d pedestrian detection leveraging specific
strengths of lidar and rgb. In IEEE international con-
ference on intelligent transportation systems (ITSC).
G
¨
ahlert, N., Mayer, M., Schneider, L., Franke, U., and Den-
zler, J. (2018). Mb-net: Mergeboxes for real-time 3d
vehicles detection. In IEEE Intelligent Vehicles Sym-
posium (IV).
He, K., Zhang, X., Ren, S., and Sun, J. (2016). Deep resid-
ual learning for image recognition. In IEEE confer-
ence on computer vision and pattern recognition.
Koh, J., Lee, J., Lee, Y., Kim, J., and Choi, J. W. (2023).
Mgtanet: Encoding sequential lidar points using long
short-term motion-guided temporal attention for 3d
object detection. In AAAI Conference on Artificial In-
telligence.
Ku, J., Mozifian, M., Lee, J., Harakeh, A., and Waslander,
S. L. (2018). Joint 3d proposal generation and ob-
ject detection from view aggregation. In IEEE/RSJ In-
ternational Conference on Intelligent Robots and Sys-
tems (IROS).
Lang, A. H., Vora, S., Caesar, H., Zhou, L., Yang, J., and
Beijbom, O. (2019). Pointpillars: Fast encoders for
object detection from point clouds. In IEEE/CVF
Conference on Computer Vision and Pattern Recog-
nition.
Liu, H., Teng, Y., Lu, T., Wang, H., and Wang, L. (2023a).
Sparsebev: High-performance sparse 3d object detec-
tion from multi-camera videos. In IEEE/CVF Interna-
tional Conference on Computer Vision.
Liu, Z., Tang, H., Amini, A., Yang, X., Mao, H., Rus, D. L.,
and Han, S. (2023b). Bevfusion: Multi-task multi-
sensor fusion with unified bird’s-eye view representa-
tion. In IEEE International Conference on Robotics
and Automation (ICRA).
Mousavian, A., Anguelov, D., Flynn, J., and Kosecka, J.
(2017). 3d bounding box estimation using deep learn-
ing and geometry. In IEEE/CVF Conference on Com-
puter Vision and Pattern Recognition.
Qi, C. R., Liu, W., Wu, C., Su, H., and Guibas, L. J. (2018).
Frustum pointnets for 3d object detection from rgb-d
data. In IEEE/CVF Conference on Computer Vision
and Pattern Recognition.
Vaswani, A., Shazeer, N., Parmar, N., Uszkoreit, J., Jones,
L., Gomez, A. N., Kaiser, Ł., and Polosukhin, I.
(2017). Attention is all you need. Advances in neural
information processing systems.
Vora, S., Lang, A. H., Helou, B., and Beijbom, O. (2020).
Pointpainting: Sequential fusion for 3d object detec-
tion. In IEEE/CVF Conference on Computer Vision
and Pattern Recognition.
Wang, S., Liu, Y., Wang, T., Li, Y., and Zhang, X. (2023).
Exploring object-centric temporal modeling for effi-
cient multi-view 3d object detection. arXiv preprint
arXiv:2303.11926.
Wang, Y., Chao, W.-L., Garg, D., Hariharan, B., Campbell,
M., and Weinberger, K. Q. (2019). Pseudo-lidar from
visual depth estimation: Bridging the gap in 3d object
detection for autonomous driving. In IEEE/CVF Con-
ference on Computer Vision and Pattern Recognition.
Weber, M., F
¨
urst, M., and Z
¨
ollner, J. M. (2019). Direct
3d detection of vehicles in monocular images with a
cnn based 3d decoder. In IEEE Intelligent Vehicles
Symposium (IV).
Yan, J., Liu, Y., Sun, J., Jia, F., Li, S., Wang, T., and Zhang,
X. (2023). Cross modal transformer: Towards fast and
robust 3d object detection. In IEEE/CVF International
Conference on Computer Vision.
Yin, T., Zhou, X., and Krahenbuhl, P. (2021). Center-based
3d object detection and tracking. In IEEE/CVF con-
ference on computer vision and pattern recognition.
Zhan, J., Liu, T., Li, R., Zhang, J., Zhang, Z., and Chen, Y.
(2023). Real-aug: Realistic scene synthesis for lidar
augmentation in 3d object detection. arXiv preprint
arXiv:2305.12853.
Zong, Z., Jiang, D., Song, G., Xue, Z., Su, J., Li, H., and
Liu, Y. (2023). Temporal enhanced training of multi-
view 3d object detector via historical object predic-
tion. arXiv preprint arXiv:2304.00967.
Learned Fusion: 3D Object Detection Using Calibration-Free Transformer Feature Fusion
223