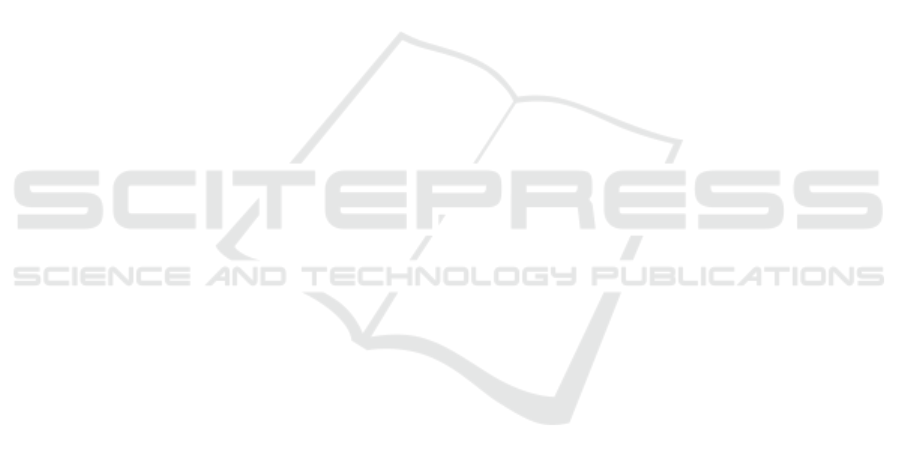
REFERENCES
Baevski, A., Zhou, Y., Mohamed, A., and Auli, M. (2020).
wav2vec 2.0: A framework for self-supervised learn-
ing of speech representations. Advances in neural in-
formation processing systems, 33:12449–12460.
Bigioi, D., Basak, S., Jordan, H., McDonnell, R., and Cor-
coran, P. (2023). Speech driven video editing via
an audio-conditioned diffusion model. arXiv preprint
arXiv:2301.04474.
Blanz, V. and Vetter, T. (1999). A morphable model for
the synthesis of 3d faces. In Proceedings of the 26th
annual conference on Computer graphics and inter-
active techniques, pages 187–194.
Blattmann, A., Rombach, R., Ling, H., Dockhorn, T., Kim,
S. W., Fidler, S., and Kreis, K. (2023). Align your la-
tents: High-resolution video synthesis with latent dif-
fusion models. arXiv preprint arXiv:2304.08818.
Bulat, A. and Tzimiropoulos, G. (2017). How far are we
from solving the 2d & 3d face alignment problem?
(and a dataset of 230,000 3d facial landmarks). In
International Conference on Computer Vision.
Chen, L., Cui, G., Liu, C., Li, Z., Kou, Z., Xu, Y., and
Xu, C. (2020). Talking-head generation with rhythmic
head motion. In Computer Vision–ECCV 2020: 16th
European Conference, Glasgow, UK, August 23–28,
2020, Proceedings, Part IX, pages 35–51. Springer.
Chen, L., Maddox, R. K., Duan, Z., and Xu, C.
(2019). Hierarchical cross-modal talking face gen-
erationwith dynamic pixel-wise loss. arXiv preprint
arXiv:1905.03820.
Cho, J., Lei, J., Tan, H., and Bansal, M. (2021). Unifying
vision-and-language tasks via text generation. In In-
ternational Conference on Machine Learning, pages
1931–1942. PMLR.
Chung, J. S. and Zisserman, A. (2016). Out of time: auto-
mated lip sync in the wild. In Workshop on Multi-view
Lip-reading, ACCV.
Cordonnier, J.-B., Loukas, A., and Jaggi, M. (2019). On the
relationship between self-attention and convolutional
layers. arXiv preprint arXiv:1911.03584.
Dao, T., Fu, D. Y., Ermon, S., Rudra, A., and R
´
e, C. (2022).
FlashAttention: Fast and memory-efficient exact at-
tention with IO-awareness. In Advances in Neural In-
formation Processing Systems.
Dhariwal, P. and Nichol, A. (2021). Diffusion models beat
gans on image synthesis. Advances in Neural Infor-
mation Processing Systems, 34:8780–8794.
Dosovitskiy, A., Beyer, L., Kolesnikov, A., Weissenborn,
D., Zhai, X., Unterthiner, T., Dehghani, M., Minderer,
M., Heigold, G., Gelly, S., et al. (2020). An image is
worth 16x16 words: Transformers for image recogni-
tion at scale. arXiv preprint arXiv:2010.11929.
Doukas, M. C., Zafeiriou, S., and Sharmanska, V. (2021).
Headgan: One-shot neural head synthesis and editing.
In Proceedings of the IEEE/CVF International Con-
ference on Computer Vision, pages 14398–14407.
Esser, P., Rombach, R., and Ommer, B. (2021). Tam-
ing transformers for high-resolution image synthesis.
In Proceedings of the IEEE/CVF conference on com-
puter vision and pattern recognition, pages 12873–
12883.
Geyer, C. J. (1992). Practical markov chain monte carlo.
Statistical science, pages 473–483.
Guo, Y., Liu, Z., Chen, D., and Chen, Q. (2021). Ad-nerf:
Audio driven neural radiance fields for talking head
synthesis. In Proceedings of the IEEE/CVF Interna-
tional Conference on Computer Vision, pages 12388–
12397.
Heusel, M., Ramsauer, H., Unterthiner, T., Nessler, B., and
Hochreiter, S. (2017). Gans trained by a two time-
scale update rule converge to a local nash equilibrium.
Advances in neural information processing systems,
30.
Ho, J., Jain, A., and Abbeel, P. (2020). Denoising diffusion
probabilistic models. Advances in Neural Information
Processing Systems, 33:6840–6851.
Huang, Z., Zhang, T., Heng, W., Shi, B., and Zhou, S.
(2020). Rife: Real-time intermediate flow estima-
tion for video frame interpolation. arXiv preprint
arXiv:2011.06294.
Isola, P., Zhu, J.-Y., Zhou, T., and Efros, A. A. (2017).
Image-to-image translation with conditional adversar-
ial networks. In Proceedings of the IEEE conference
on computer vision and pattern recognition, pages
1125–1134.
Korshunov, P. and Marcel, S. (2022). The threat of deep-
fakes to computer and human visions. In Hand-
book of Digital Face Manipulation and Detection:
From DeepFakes to Morphing Attacks, pages 97–115.
Springer International Publishing Cham.
Kotevski, Z. and Mitrevski, P. (2010). Experimental com-
parison of psnr and ssim metrics for video quality es-
timation. In ICT Innovations 2009, pages 357–366.
Springer.
Liu, H., Chen, Z., Yuan, Y., Mei, X., Liu, X., Mandic, D.,
Wang, W., and Plumbley, M. D. (2023). Audioldm:
Text-to-audio generation with latent diffusion models.
arXiv preprint arXiv:2301.12503.
Lovelace, J., Kishore, V., Wan, C., Shekhtman, E., and
Weinberger, K. (2022). Latent diffusion for language
generation. arXiv preprint arXiv:2212.09462.
Mildenhall, B., Srinivasan, P. P., Tancik, M., Barron, J. T.,
Ramamoorthi, R., and Ng, R. (2021). Nerf: Repre-
senting scenes as neural radiance fields for view syn-
thesis. Communications of the ACM, 65(1):99–106.
Mirza, M. and Osindero, S. (2014). Conditional generative
adversarial nets. arXiv preprint arXiv:1411.1784.
Ngo, L. M., de Wiel, C. a., Karaoglu, S., and Gevers, T.
(2020). Unified application of style transfer for face
swapping and reenactment. In Proceedings of the
Asian Conference on Computer Vision (ACCV).
Peebles, W. and Xie, S. (2022). Scalable diffusion models
with transformers. arXiv preprint arXiv:2212.09748.
Prajwal, K., Mukhopadhyay, R., Namboodiri, V. P., and
Jawahar, C. (2020). A lip sync expert is all you need
for speech to lip generation in the wild. In Proceed-
ings of the 28th ACM International Conference on
Multimedia, pages 484–492.
ICAART 2024 - 16th International Conference on Agents and Artificial Intelligence
168