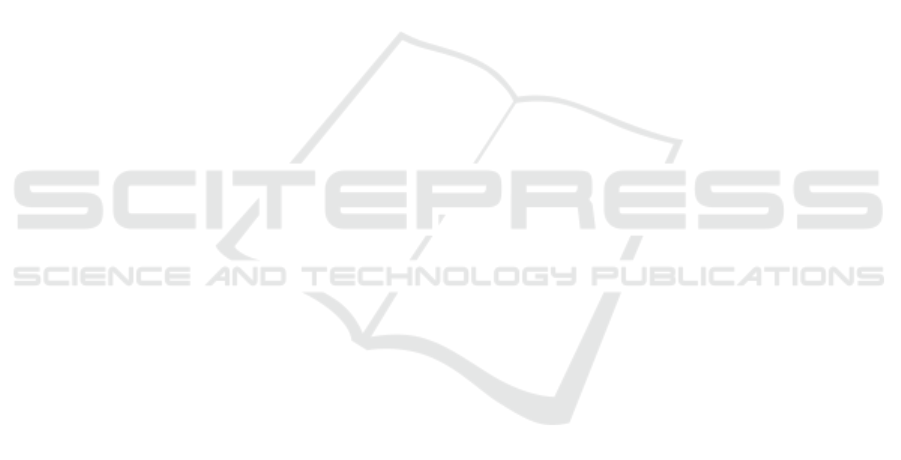
gists and administrators in hospitals in their decision-
making process (e.g. better admission control in the
ER, more isolation of infected patients, or greater care
in the movement of at-risk patients). It can also be ap-
plied, for example, in the Objective Structured Clin-
ical Examination (OSCE), where the skills acquired
by students upon completing their medical degree are
evaluated. In these tests, they create simulated sce-
narios and students must make a series of decisions
as doctors.
Our following step is to validate the visual tool
by means of qualitative evaluations with specialized
users to ensure its usability and usefulness.
6 CONCLUSIONS
In this paper, we propose a decision-support visual
tool to help intertwine the spatial-temporal locations
of hospitalized patients with information about their
health conditions during the spread of an infection
by multidrug-resistant bacteria. To do this, the tool
combines several views which include a 3D visualiza-
tion of a hospital, a 2D visualization of the temporal
progression of the disease, and a tabular visualization
to detail the information depicted in the other views.
The interactivity of the created 3D model allows for
a faithful representation of the movements and the in-
fection processes, while the use of widespread charts
can help understand the temporal progress through
epidemiological indicators. The tasks were defined
together with epidemiologists from hospitals from
Murcia, and we consider that this tool is going to be of
utility in different areas (infection control, decisions
in a hospital, teaching). Our next step is to validate
the usefulness and usability of the tool with said users.
ACKNOWLEDGEMENTS
This work was partially funded by the CON-
FAINCE project (Ref: PID2021-122194OB-I00) by
MCIN/AEI/10.13039/501100011033 and by ”ERDF
A way of making Europe”, by the ”European
Union” or by the ”European Union NextGenera-
tionEU/PRTR”. This research is also partially funded
by the FPI program grant (Ref: PRE2019-089806).
REFERENCES
Aigner, W., Miksch, S., Schumann, H., and Tominski, C.
(2011). Visualization of Time-Oriented Data. Human-
Computer Interaction Series. Springer, London.
Baumgartl, T., Petzold, M., Wunderlich, M., H
¨
ohn, M.,
Archambault, D., Lieser, M., Dalpke, A., Schei-
thauer, S., Marschollek, M., Eichel, V. M., Mut-
ters, N. T., Consortium, H., and von Landesberger, T.
(2021). In Search of Patient Zero: Visual Analytics of
Pathogen Transmission Pathways in Hospitals. IEEE
Transactions on Visualization and Computer Graph-
ics, 27(2):711–721.
Brauer, F. (2008). Compartmental Models in Epidemiology.
Mathematical Epidemiology, 1945:19–79.
Caban, J. J. and Gotz, D. (2015). Visual analytics in health-
care – opportunities and research challenges. Jour-
nal of the American Medical Informatics Association,
22(2):260–262.
Cassini, A., H
¨
ogberg, L. D., Plachouras, D., Quattrocchi,
A., Hoxha, A., Simonsen, G. S., Colomb-Cotinat,
M., Kretzschmar, M. E., Devleesschauwer, B., Cec-
chini, M., Ouakrim, D. A., Oliveira, T. C., Strue-
lens, M. J., Suetens, C., and Monnet, D. L. (2019).
Attributable deaths and disability-adjusted life-years
caused by infections with antibiotic-resistant bacteria
in the EU and the European Economic Area in 2015:
a population-level modelling analysis. The Lancet. In-
fectious Diseases, 19(1):56–66.
Chorianopoulos, K. and Talvis, K. (2016). Flutrack.org:
Open-source and linked data for epidemiology. Health
informatics journal, 22(4):962–974.
Hota, S. S., Achonu, C., Crowcroft, N. S., Harvey, B. J.,
Lauwers, A., and Gardam, M. A. (2012). Determining
Mortality Rates Attributable to Clostridium difficile
Infection. Emerging Infectious Diseases, 18(2):305–
307.
Kim, D., Canovas-Segura, B., Jimeno-Almaz
´
an, A., Cam-
pos, M., and Juarez, J. M. (2023). Spatial-temporal
simulation for hospital infection spread and outbreaks
of Clostridioides difficile. Scientific Reports, 13(1).
Number: 1 Publisher: Nature Publishing Group.
K
¨
onig, B., Limberger, D., Klimke, J., Hagedorn, B., and
D
¨
ollner, J. (2021). RoomCanvas: A Visualization Sys-
tem for Spatiotemporal Temperature Data in Smart
Homes. The Eurographics Association.
Lital Meyer, S., Ricardo Espinoza, A., and Rodrigo Quera,
P. (2014). Infecci
´
on por clostridium difficile: epidemi-
olog
´
ıa, diagn
´
ostico y estrategias terap
´
euticas. Revista
M
´
edica Cl
´
ınica Las Condes, 25(3):473–484. Pub-
lisher: Elsevier.
Monegro, A. F., Muppidi, V., and Regunath, H. (2023).
Hospital-Acquired Infections. In StatPearls. Stat-
Pearls Publishing, Treasure Island (FL).
Munzner, T. (2009). A Nested Model for Visualization De-
sign and Validation. IEEE Transactions on Visualiza-
tion and Computer Graphics, 15(6):921–928.
Munzner, T. (2015). Visualization Analysis and Design. A
K Peters/CRC Press.
M
¨
uller, M., Petzold, M., Wunderlich, M., Baumgartl, T.,
H
¨
ohn, M., Eichel, V., Mutters, N. T., Scheithauer, S.,
Marschollek, M., and Landesberger, T. v. (2020). Vi-
sual Analysis for Hospital Infection Control using a
RNN Model. The Eurographics Association.
Spatial-Temporal Visualization Tool for Hospital Support for Infection Spread and Outbreaks
313