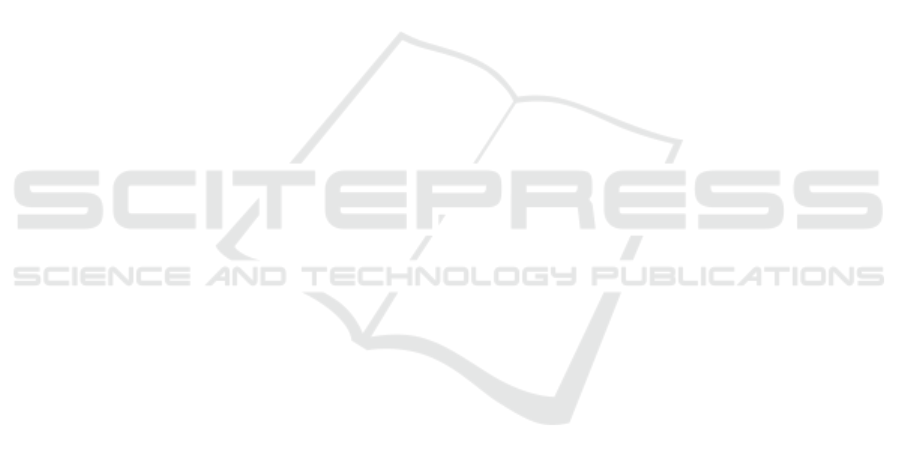
ing an ABM, by RCGA. Conventionally, researchers
have set the parameters of ABMs by referring to styl-
ized facts. However, in recent years, an increas-
ing number of studies have attempted to estimate
the parameters of ABMs using quantitative methods
such as heuristic methods or Bayesian estimation-
based methods. In this study, we attempted to esti-
mate time-varying model parameters using evolution-
ary computation methods based on the concept of the
inverse simulation method proposed by (Kurahashi,
1999). From the experimental results, we confirmed
that parameter estimation by evolutionary computa-
tion works well in cases where the economic transi-
tion to be assimilated is stable. On this basis, we con-
firmed that parameter search using evolutionary com-
putation works well as an inverse simulation method.
Conversely, we confirmed that it is difficult to esti-
mate appropriate parameters in cases where the eco-
nomic situation to be assimilated changes in the short
term. One reason for this may be that it may be diffi-
cult to fit the economic fluctuations in the short term
with fixed model parameters.
One of our future tasks will be to develop a more
appropriate method of measuring the evaluation val-
ues of individuals in evolutionary computation. Cur-
rently, the absolute error is calculated for multiple so-
cial indicators to be assimilated, and the sum of these
values is used as the individual’s evaluation value.
However, it may be more appropriate to use a single
social indicator. As described in the previous section,
it is difficult to evaluate parameters when multiple in-
dicators are used, some of which are good while oth-
ers are not. The second issue is to develop a method
for capturing changes in parameter values in the short
term. In the analysis of this study, the economic sim-
ulator was run for 24 steps to evaluate each individ-
ual. The parameters used in the simulation reflected
the parameters estimated by evolutionary computa-
tion in the economic simulator only at the beginning
of the simulation, and the reflected parameters were
then continued. This is because the genes of each in-
dividual in the RCGA corresponded to each MABM
parameter. We would like to confirm as a future issue
whether it is possible to capture changes in parameter
values in cases wherein economic conditions change
in the short term by setting genes corresponding to
each parameter at each point in time.
REFERENCES
Fagiolo, G. and Richiardi, M. (2018). Empirical
validation of agent-based models. Agent-Based
Models in Economics: A Toolkit (pp. 163–182).
Cambridge: Cambridge University Press.
Delli Gatti, D. and Grazzini, J. (2020). Rising to the
challenge: bayesian estimation and forecasting
techniques for macroeconomic agent based mod-
els. Journal of Economic Behavior and Organi-
zation, Vol.178, pp.875–902.
Fagiolo, G. and Roventini, A. (2012). Macroeco-
nomic policy in dsge and agent-based models..
Revue de l’OFCE, Vol.124, No.5, pp.67–116.
Dawid, H. and Delli Gatti, D. Agent-based macroe-
conomics. (2018). Handbook of Computational
Economics, Vol.4, Elsevier, pp.63–156.
Caiani, A., Godin, A., Caverzasi, E., Gallegati, M.,
Kinsella, S. and Stiglitz, J. (2016). Agent based-
stock flow consistent macroeconomics: Toward
a benchmark model. Journal of Economic Dy-
namics and Control, Vol.69, pp.375–408.
Obata, T., Sakazaki J., and Kurahashi, S. (2023)
Building a macroeconomic simulator with multi-
layered supplier–customer relationships. Risks,
Vol.11 ,No.7, pp. 128.
Grazzini, J., Richiardi, M. G., and Tsionas, M.
(2017). Bayesian estimation of agent-based
models. Journal of Economic Dynamics and
Control, Vol.77, pp.26–47.
Lux, T. (2022). Bayesian estimation of agent-based
models via adaptive particle markov chain monte
carlo. Computational Economics 60, pp.451–
477.
Kurahashi, S. (2013). State-of-the-art of social system
research : model estimation and inverse simu-
lation. (Japanese) Journal of the Society of In-
strument and Control Engineers Vol. 52, No. 7,
pp.588–594.
Kurahashi, S., Minami, U., and Terano, T. (1999). In-
verse Simulation for analyzing models of artifi-
cial societies. (Japanese) Transactions of the So-
ciety of Instrument and Control Engineers Vol.
35, No. 11, pp.1454–1461.
Alden H. Wright. (1991). Genetic algorithms for real
parmter optimization. Foundations of Genetic
Algorithms, pp.205–218.
Fukushima, N., Nagata, Y., Kobayashi, S. and Ono,
I. (2013). Distance-weighted exponential natu-
ral evolution strategy and its performance evalu-
ation. (Japanese) Transaction of the Japanese So-
ciety for Evolutionary Computation Vol. 4, No.
2, pp.57–73.
Poledna, S., Miess, M. G., Hommes, C., and Ra-
bitsch, K. (2023). Economic forecasting with an
agent-based model. European Economic Review
Vol. 151, 104306.
ICAART 2024 - 16th International Conference on Agents and Artificial Intelligence
212