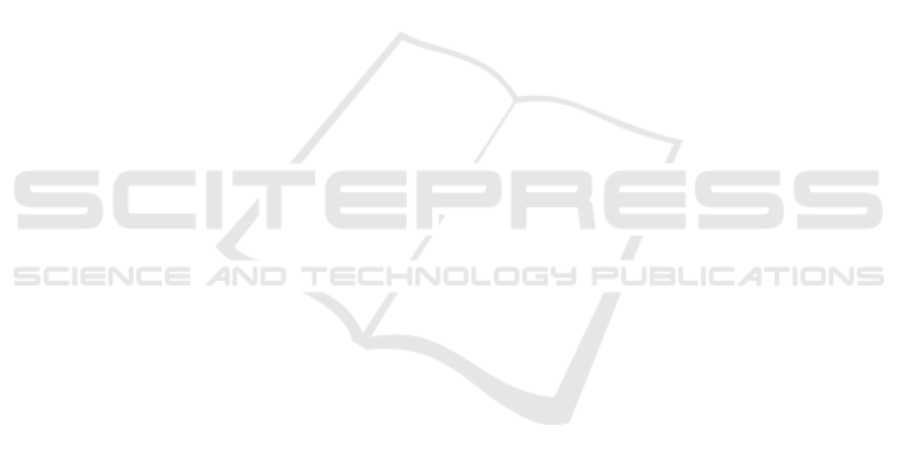
REFERENCES
Brostow, G. J. and et al. (2009). Semantic object classes in
video: A high-definition ground truth database. Pat-
tern Recognition Letters, 30.
Chandra, S. and et al. (2018). Deep spatio-temporal random
fields for efficient video segmentation. In Proceedings
of the IEEE Conference on CVPR, pages 8915–8924.
Chen, L.-C., Zhu, Y., Papandreou, G., Schroff, F., and
Adam, H. (2018). Encoder-decoder with atrous sepa-
rable convolution for semantic image segmentation. In
Proceedings of the European conference on computer
vision (ECCV), pages 801–818.
Cheng, B. and et al. (2020). Panoptic-deeplab: A simple,
strong, and fast baseline for bottom-up panoptic seg-
mentation. In Proceedings of the IEEE/CVF confer-
ence on CVPR, pages 12475–12485.
Cheng, B. and et al. (2022). Masked-attention mask trans-
former for universal image segmentation. In Proceed-
ings of the IEEE/CVF Conference on CVPR, pages
1290–1299.
Chitta, K. and et al. (2022). Transfuser: Imitation with
transformer-based sensor fusion for autonomous driv-
ing. IEEE Transactions on PAMI.
Cordts, M. and et al. (2016). The cityscapes dataset for se-
mantic urban scene understanding. Proceedings of the
IEEE Computer Society Conference (CSC) on Com-
puter Vision and Pattern Recognition (CVPR), 2016-
December.
Dosovitskiy, A. and et al. (2020). An image is worth 16x16
words: Transformers for image recognition at scale.
arXiv preprint arXiv:2010.11929.
Feng, D. and et al. (2020). Deep multi-modal object detec-
tion and semantic segmentation for autonomous driv-
ing: Datasets, methods, and challenges. IEEE T-ITS,
22(3):1341–1360.
Geiger, A. et al. (2012). Are we ready for autonomous driv-
ing? the kitti vision benchmark suite. Proceedings of
the IEEE CSC on CVPR.
Hassan, T. and et al. (2021). Trainable structure tensors
for autonomous baggage threat detection under ex-
treme occlusion. Lecture Notes in Computer Science
(including subseries Lecture Notes in Artificial Intel-
ligence and Lecture Notes in Bioinformatics), 12627
LNCS.
He, K. and et al. (2020). Mask r-cnn. IEEE Transactions on
PAMI, 42.
Hua, Z. and et al. (2022). Dual attention based multi-scale
feature fusion network for indoor rgbd semantic seg-
mentation. In 2022 26th International Conference on
Pattern Recognition (ICPR), pages 3639–3644. IEEE.
Jain, J. and et al. (2022). OneFormer: One Transformer to
Rule Universal Image Segmentation. arXiv.
Li, P. and et al. (2019). Stereo r-cnn based 3d object de-
tection for autonomous driving. In Proceedings of the
IEEE/CVF Conference on CVPR, pages 7644–7652.
Li, Z. and et al. (2022). Maskformer with improved
encoder-decoder module for semantic segmentation of
fine-resolution remote sensing images. In 2022 IEEE
ICIP, pages 1971–1975. IEEE.
Liu, H. and et al. (2022). Cmx: Cross-modal fusion for
rgb-x semantic segmentation with transformers. IEEE
Transactions on Intelligent Transportation Systems
(T-ITS).
Liu, Y. and et al. (2020). Efficient semantic video segmen-
tation with per-frame inference. In Computer Vision–
ECCV 2020: 16th European Conference, Glasgow,
UK, August 23–28, 2020, Proceedings, Part X 16,
pages 352–368. Springer.
Nag, S. and et al. (2019). What’s there in the dark. In 2019
IEEE International Conference on Image Processing
(ICIP), pages 2996–3000. IEEE.
Neuhold, G. and et al. (2017). The mapillary vistas dataset
for semantic understanding of street scenes. Proceed-
ings of the IEEE ICCV, 2017-October.
Papadeas, I. and et al. (2021). Real-time semantic image
segmentation with deep learning for autonomous driv-
ing: A survey. Applied Sciences, 11(19):8802.
Siam, M. and et al. (2018). A comparative study of real-time
semantic segmentation for autonomous driving. In
Proceedings of the IEEE conference on CVPR work-
shops, pages 587–597.
Verelst, T. and et al. (2023). Segblocks: Block-based dy-
namic resolution networks for real-time segmentation.
IEEE Transactions on PAMI, 45(2):2400–2411.
Wang, C. Y. et al. (2021). Scaled-yolov4: Scaling cross
stage partial network. Proceedings of the IEEE CSC
on CVPR.
Wang, J. and et al. (2021). Deep high-resolution represen-
tation learning for visual recognition. IEEE Transac-
tions on PAMI, 43(10):3349–3364.
Wang, J. and et al. (2022a). Rtformer: Efficient design
for real-time semantic segmentation with transformer.
arXiv preprint arXiv:2210.07124.
Wang, W. and et al. (2022b). Pvt v2: Improved baselines
with pyramid vision transformer. Computational Vi-
sual Media, 8.
Xiao, T. and et al. (2018). Unified perceptual parsing
for scene understanding. Lecture Notes in Computer
Science (including subseries Lecture Notes in Artifi-
cial Intelligence and Lecture Notes in Bioinformatics),
11209 LNCS.
Xie, E. and et al. (2021). Segformer: Simple and efficient
design for semantic segmentation with transformers.
Advances in Neural Information Processing Systems,
34:12077–12090.
Yin, W. and et al. (2022). The devil is in the labels: Se-
mantic segmentation from sentences. Conference on
CVPR.
Yu, F. et al. (2020). Bdd100k: A diverse driving dataset
for heterogeneous multitask learning. In Proceedings
of the IEEE/CVF conference on CVPR, pages 2636–
2645.
Zheng, S. et al. (2021). Rethinking semantic segmentation
from a sequence-to-sequence perspective with trans-
formers. In Proceedings of the IEEE/CVF conference
on CVPR, pages 6881–6890.
Zhou, B. et al. (2017). Scene parsing through ade20k
dataset. In Proceedings of the IEEE Conference on
CVPR.
VISAPP 2024 - 19th International Conference on Computer Vision Theory and Applications
332