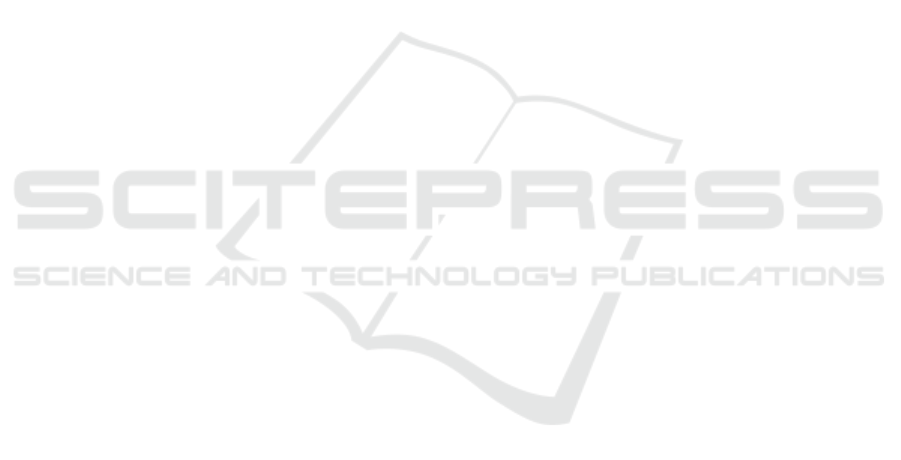
ETHICAL CONSIDERATIONS
The Ethics Review Committee of Ehime University
Hospital approved this study (“Quality evaluation of
synthetic data generation methods preserving statis-
tical characteristics,” Permission number 2012001),
and we conducted it in accordance with the commit-
tee’s guidelines.
REFERENCES
Abadi, M., Chu, A., Goodfellow, I., McMahan, H. B.,
Mironov, I., Talwar, K., and Zhang, L. (2016). Deep
learning with differential privacy. In Proceedings of
the 2016 ACM SIGSAC conference on computer and
communications security, pages 308–318.
Aggarwal, C. C. (2005). On k-anonymity and the curse of
dimensionality. In VLDB, volume 5, pages 901–909.
Asghar, H. J., Ding, M., Rakotoarivelo, T., Mrabet, S., and
Kaafar, D. (2020). Differentially private release of
datasets using gaussian copula. Journal of Privacy
and Confidentiality, 10(2).
Azizi, Z., Zheng, C., Mosquera, L., Pilote, L., and
El Emam, K. (2021). Can synthetic data be a proxy
for real clinical trial data? a validation study. BMJ
open, 11(4):e043497.
Barth-Jones, D. (2012). The’re-identification’of governor
william weld’s medical information: a critical re-
examination of health data identification risks and pri-
vacy protections, then and now. Then and Now (July
2012).
Chen, Q., Xiang, C., Xue, M., Li, B., Borisov, N., Kaarfar,
D., and Zhu, H. (2018). Differentially private data
generative models. arXiv preprint arXiv:1812.02274.
Cramer, R., Damg
˚
ard, I. B., and Nielsen, J. B. (2015).
Secure Multiparty Computation and Secret Sharing.
Cambridge University Press.
Culnane, C., Rubinstein, B. I., and Teague, V. (2017).
Health data in an open world. arXiv preprint
arXiv:1712.05627.
Dankar, F. K., Ibrahim, M. K., and Ismail, L. (2022). A
multi-dimensional evaluation of synthetic data gener-
ators. IEEE Access, 10:11147–11158.
Drechsler, J. and Reiter, J. (2009). Disclosure risk and data
utility for partially synthetic data: An empirical study
using the german iab establishment survey. Journal of
Official Statistics, 25(4):589–603.
Dwork, C. (2006). Differential privacy. In International col-
loquium on automata, languages, and programming,
pages 1–12. Springer.
Dwork, C., Roth, A., et al. (2014). The algorithmic foun-
dations of differential privacy. Found. Trends Theor.
Comput. Sci., 9(3-4):211–407.
El Emam, K. (2020). Seven ways to evaluate the utility of
synthetic data. IEEE Security & Privacy, 18(4):56–
59.
El Emam, K., Mosquera, L., Fang, X., and El-Hussuna, A.
(2022). Utility metrics for evaluating synthetic health
data generation methods: validation study. JMIR med-
ical informatics, 10(4):e35734.
Fang, M. L., Dhami, D. S., and Kersting, K. (2022).
Dp-ctgan: Differentially private medical data gen-
eration using ctgans. In Artificial Intelligence in
Medicine: 20th International Conference on Artificial
Intelligence in Medicine, AIME 2022, Halifax, NS,
Canada, June 14–17, 2022, Proceedings, pages 178–
188. Springer.
Guibas, J. T., Virdi, T. S., and Li, P. S. (2017). Synthetic
medical images from dual generative adversarial net-
works. arXiv preprint arXiv:1709.01872.
Guo, A., Foraker, R. E., MacGregor, R. M., Masood, F. M.,
Cupps, B. P., and Pasque, M. K. (2020). The use of
synthetic electronic health record data and deep learn-
ing to improve timing of high-risk heart failure sur-
gical intervention by predicting proximity to catas-
trophic decompensation. Frontiers in digital health,
2:576945.
Hernandez, M., Epelde, G., Alberdi, A., Cilla, R., and
Rankin, D. (2022). Synthetic data generation for tab-
ular health records: A systematic review. Neurocom-
puting, 493:28–45.
Kotelnikov, A., Baranchuk, D., Rubachev, I., and Babenko,
A. (2022). Tabddpm: Modelling tabular data with dif-
fusion models. arXiv preprint arXiv:2209.15421.
Lee, J., Kim, M., Jeong, Y., and Ro, Y. (2022). Differen-
tially private normalizing flows for synthetic tabular
data generation. In Proceedings of the AAAI Con-
ference on Artificial Intelligence, volume 36, pages
7345–7353.
Li, H., Xiong, L., Zhang, L., and Jiang, X. (2014). Dp-
synthesizer: Differentially private data synthesizer for
privacy preserving data sharing. In Proceedings of the
VLDB Endowment International Conference on Very
Large Data Bases, volume 7, page 1677. NIH Public
Access.
Liew, S. P., Takahashi, T., and Ueno, M. (2022). PEARL:
Data synthesis via private embeddings and adversarial
reconstruction learning. In International Conference
on Learning Representations.
McKenna, R., Mullins, B., Sheldon, D., and Miklau, G.
(2022). Aim: An adaptive and iterative mechanism
for differentially private synthetic data. arXiv preprint
arXiv:2201.12677.
McKenna, R., Sheldon, D., and Miklau, G. (2019).
Graphical-model based estimation and inference for
differential privacy. In International Conference on
Machine Learning, pages 4435–4444. PMLR.
Shan, Z., Ren, K., Blanton, M., and Wang, C. (2018). Prac-
tical secure computation outsourcing: A survey. ACM
Computing Surveys (CSUR), 51(2):1–40.
Sklar, M. (1959). Fonctions de repartition an dimensions
et leurs marges. Publ. inst. statist. univ. Paris, 8:229–
231.
Stadler, T., Oprisanu, B., and Troncoso, C. (2022). Syn-
thetic data–anonymisation groundhog day. In 31st
Evaluating Synthetic Data Generation Techniques for Medical Dataset
321