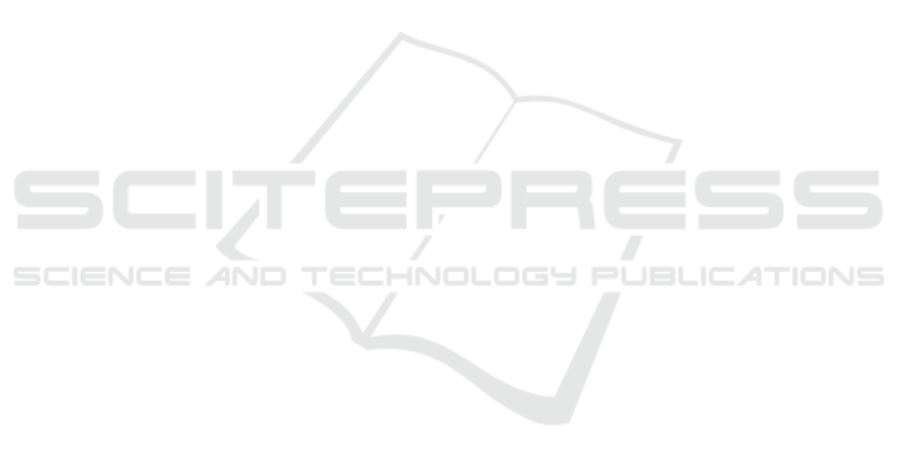
REFERENCES
Ackerman, S., Farchi, E., Raz, O., et al. (2020). Detec-
tion of data drift and outliers affecting machine learn-
ing model performance over time. arXiv preprint
arXiv:2012.09258.
Ackerman, S., Raz, O., Zalmanovici, M., and Zlotnick, A.
(2021). Automatically detecting data drift in machine
learning classifiers. arXiv preprint arXiv:2111.05672.
Bayram, F., Ahmed, B. S., and Kassler, A. (2022). From
concept drift to model degradation: An overview on
performance-aware drift detectors. Knowledge-Based
Systems.
Cordts, M., Omran, M., Ramos, S., Rehfeld, T., et al.
(2016). The cityscapes dataset for semantic urban
scene understanding. In CVPR.
Davis, S. E., Greevy Jr, R. A., Fonnesbeck, C., Lasko, T. A.,
et al. (2019). A nonparametric updating method to
correct clinical prediction model drift. Journal of the
American Medical Informatics Association.
Devarakota, P., Gala, A., Li, Z., Alkan, E., et al. (2022).
Deep learning in salt interpretation from r&d to de-
ployment: Challenges and lessons learned. In Second
International Meeting for Applied Geoscience & En-
ergy.
Dries, A. and R
¨
uckert, U. (2009). Adaptive concept drift
detection. Statistical Analysis and Data Mining: The
ASA Data Science Journal.
Everingham, M., Van Gool, L., Williams, C. K., et al.
(2010). The pascal visual object classes (voc) chal-
lenge. International journal of computer vision.
Guerra-Manzanares, A., Luckner, M., and Bahsi, H. (2022).
Android malware concept drift using system calls: de-
tection, characterization and challenges. Expert Sys-
tems with Applications.
He, K., Zhang, X., Ren, S., and Sun, J. (2015). Spatial pyra-
mid pooling in deep convolutional networks for visual
recognition. IEEE transactions on pattern analysis
and machine intelligence.
Hofer, V. and Krempl, G. (2013). Drift mining in data: A
framework for addressing drift in classification. Com-
putational Statistics & Data Analysis.
Johannes, J., Michael, V., Niklas, K., et al. (2023). Data-
centric artificial intelligence.
Khaldi, K., Mantini, P., and Shah, S. K. (2022). Unsuper-
vised person re-identification based on skeleton joints
using graph convolutional networks. In International
Conference on Image Analysis and Processing.
Klinkenberg, R. and Joachims, T. (2000). Detecting concept
drift with support vector machines. In ICML.
Lacson, R., Eskian, M., Licaros, A., et al. (2022). Machine
learning model drift: predicting diagnostic imaging
follow-up as a case example. Journal of the Ameri-
can College of Radiology.
Lu, J., Liu, A., Dong, F., et al. (2018). Learning under con-
cept drift: A review. IEEE transactions on knowledge
and data engineering.
Mittal, A., Moorthy, A. K., and Bovik, A. C. (2012). No-
reference image quality assessment in the spatial do-
main. IEEE Transactions on image processing.
Rahmani, K., Thapa, R., Tsou, P., Chetty, S. C., Barnes,
G., et al. (2023). Assessing the effects of data drift
on the performance of machine learning models used
in clinical sepsis prediction. International Journal of
Medical Informatics.
Ronneberger, O., Fischer, P., and Brox, T. (2015). U-net:
Convolutional networks for biomedical image seg-
mentation. In MICCAI.
Tsymbal, A. (2004). The problem of concept drift: defi-
nitions and related work. Computer Science Depart-
ment, Trinity College Dublin.
Wang, H. and Abraham, Z. (2015). Concept drift detection
for streaming data. In IJCNN.
Wang, P., Jin, N., Woo, W. L., et al. (2022). Noise tolerant
drift detection method for data stream mining. Infor-
mation Sciences.
Webb, G. I., Hyde, R., Cao, H., et al. (2016). Characterizing
concept drift. Data Mining and Knowledge Discovery.
Whang, S. E., Roh, Y., Song, H., and Lee, J.-G. (2023).
Data collection and quality challenges in deep learn-
ing: A data-centric ai perspective. The VLDB Journal.
Zha, D., Bhat, Z. P., Lai, K.-H., Yang, F., et al. (2023). Data-
centric artificial intelligence: A survey.
Zhou, B., Zhao, H., Puig, X., Fidler, S., et al. (2017). Scene
parsing through ade20k dataset. In CVPR.
Zhou, B., Zhao, H., Puig, X., Xiao, T., et al. (2019). Se-
mantic understanding of scenes through the ade20k
dataset. International Journal of Computer Vision.
Data Quality Aware Approaches for Addressing Model Drift of Semantic Segmentation Models
341