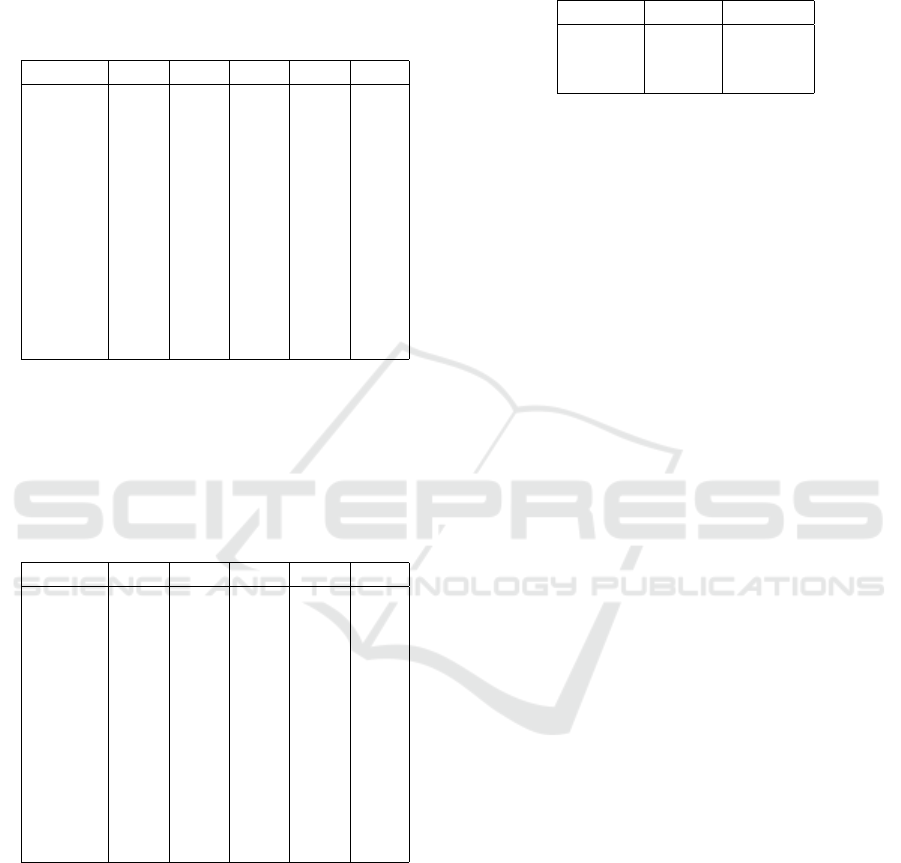
Table 2: Sensitivities for experiments with different combi-
nations of real and augmented data for the left side. Col-
umn ”Real” contains the results from the baseline with only
real data. The columns ”10%”, ”30%” and ”50%” contain
the results from experiments with the corresponding amount
of augmented data. ”Gap” refers to the approach of only
adding as much augmented data as needed to balance the
data set for those users with smaller amounts of data.
User ID Real 10% 30% 50% Gap
U1 0.96 0.96 0.96 0.94 0.96
U2 0.88 0.89 0.89 0.86 0.91
U3 0.91 0.93 0.94 0.90 0.91
U4 0.93 0.93 0.94 0.91 0.93
U5 0.96 0.97 0.97 0.96 0.97
U6 0.94 0.96 0.96 0.93 0.94
U7 0.90 0.91 0.93 0.91 0.93
U8 0.92 0.93 0.93 0.91 0.95
U9 0.69 0.69 0.70 0.70 0.74
U10 0.81 0.82 0.85 0.82 0.86
U11 0.66 0.67 0.69 0.67 0.76
U12 0.93 0.93 0.94 0.90 0.95
Table 3: Sensitivities for experiments with different combi-
nations of real and augmented data for the right side. Col-
umn ”Real” contains the results from the baseline with only
real data. The columns ”10%”, ”30%” and ”50%” contain
the results from experiments with the corresponding amount
of augmented data. ”Gap” refers to the approach of only
adding as much augmented data as needed to balance the
data set for those users with smaller amounts of data.
User ID Real 10% 30% 50% Gap
U1 0.95 0.95 0.95 0.93 0.96
U2 0.93 0.94 0.94 0.89 0.95
U3 0.92 0.92 0.93 0.90 0.94
U4 0.94 0.94 0.94 0.91 0.94
U5 0.92 0.93 0.94 0.91 0.94
U6 0.93 0.94 0.95 0.90 0.96
U7 0.90 0.91 0.91 0.90 0.93
U8 0.92 0.93 0.93 0.91 0.95
U9 0.66 0.69 0.70 0.64 0.74
U10 0.83 0.83 0.85 0.82 0.86
U11 0.69 0.70 0.72 0.67 0.76
U12 0.92 0.93 0.94 0.90 0.95
ilar to the original images. Interestingly, when we an-
alyze the two resulting confusion matrices from the
second evaluation method (one using GANs and the
other using CGANs with augmented real data in a
3:7 ratio), we notice minor differences. Despite us-
ing GANs to augment the dataset, the performance
improvement of the CNN is just slightly better, about
0-1%, compared to using CGANs. This is intriguing
given that GANs require about 8 hours of training per
label, whereas CGANs can train across all labels si-
multaneously in the same time frame. This small per-
Table 4: Results from the pre-experiments investigating the
difference in synthetic images generated by the GANs vs.
the CGAN based on a subset of three users (U1-U3). Shows
the Fr
´
echet inception distance for each user and both kinds
of generated data.
User ID GANS CGANS
U1 11 23
U2 10 18
U3 12 29
formance gap prompts us to consider the efficiency of
these two training approaches.
For the experimentation conducted on the com-
plete dataset enriched with CGAN-generated sam-
ples, our approach began with the integration of 10%
augmented data alongside the authentic images. The
outcomes demonstrated an initial marginal enhance-
ment of 1-2% across at least 8 out of the 12 users.
To provide further insight, we present the sensitivities
pertaining to various blends of real and augmented
data in Table 2 and 3. However, as the augmenta-
tion escalated to encompass 50% augmented data and
50% real data, a decline in results became evident,
indicative of overfitting. This phenomenon was illus-
trated by user U1, where the sensitivity dropped from
96% with only real data to 94% upon introducing 50%
augmented data. This pattern was echoed across nu-
merous labels, reflecting a decrease in accuracy by
3-4%.
Interestingly, a turning point was observed when
we employed 30% augmented data and 70% real
data. This configuration yielded promising outcomes,
showcasing a consistent rise in sensitivity across all
12 users. Optimal results materialized when we
strategically utilized CGAN-generated synthetic data
to bridge gaps in labels that required additional in-
stances to achieve a balanced dataset of 1,000 images
per user. Notably, some users, like U4, U5, U6, and
U12, already possessed over 1,000 images, render-
ing augmentation unnecessary. However, users such
as U1, U2, and U3, who required a modest influx
of CGAN-generated images to attain the 1,000-image
threshold, experienced modest performance improve-
ments ranging from 0-3%.
Employing this methodology yielded a general
augmentation in sensitivity for all users. Particu-
larly remarkable were the advancements in sensitiv-
ity achieved for labels U9, U10, and U11, which ex-
hibited increases of 6-10% using this approach, as
meticulously illustrated in Table 2 and 3. This under-
scores the efficacy of judiciously introducing CGAN-
generated data to enrich datasets, resulting in substan-
tial improvements across diverse users and labels.
HEALTHINF 2024 - 17th International Conference on Health Informatics
344