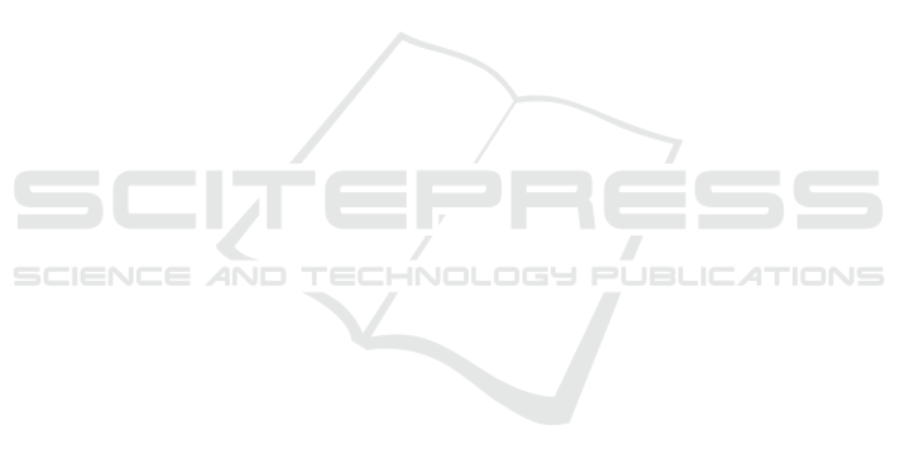
4 CONCLUSION
In this study, we have discovered that SSL frame-
works can provide suitable representations for the
analysis of micro-CT images of rock. These repre-
sentations significantly enhance the performance of
downstream tasks compared to traditional supervised
learning methods across all label fractions. The chal-
lenges posed by the scarcity and cost associated with
manual annotation in the domain of DRP make it diffi-
cult to acquire datasets of a scale comparable to those
in well-established computer vision domains. Our
success in showcasing performance improvements
over traditional supervised learning methods, particu-
larly in scenarios with limited labeled data, holds the
potential for broader applications in micro-CT image
analysis, encompassing both 2D and 3D representa-
tions characterized by intricate textures. A pretrained
network tailored for DRP can be used for different
purposes, including classification, segmentation, and
the estimation of rock properties.
Based on our analysis, we anticipate that in-
creased computational resources allocated to model
training could result in improved performance. Addi-
tionally, we identify the potential for further research,
particularly in the exploration of various downstream
tasks such as regression which are of interest for 3D
tomography of rock images.
REFERENCES
Ahmad, I. and Shin, S. (2021). An approach to run pre-
trained deep learning models on grayscale images.
In 2021 International Conference on Artificial Intel-
ligence in Information and Communication (ICAIIC),
pages 177–180.
Andr
¨
a, H., Combaret, N., Dvorkin, J., Glatt, E., Han, J., Ka-
bel, M., Keehm, Y., Krzikalla, F., Lee, M., Madonna,
C., et al. (2013). Digital rock physics bench-
marks—part i: Imaging and segmentation. Computers
& Geosciences, 50:25–32.
Blunt, M. J., Bijeljic, B., Dong, H., Gharbi, O., Iglauer, S.,
Mostaghimi, P., Paluszny, A., and Pentland, C. (2013).
Pore-scale imaging and modelling. Advances in Water
resources, 51:197–216.
Chen, T., Kornblith, S., Norouzi, M., and Hinton, G.
(2020a). A simple framework for contrastive learning
of visual representations. In International conference
on machine learning, pages 1597–1607. PMLR.
Chen, X., Fan, H., Girshick, R., and He, K. (2020b). Im-
proved baselines with momentum contrastive learn-
ing. arXiv preprint arXiv:2003.04297.
Cheng, G. and Guo, W. (2017). Rock images classification
by using deep convolution neural network. In Jour-
nal of Physics: Conference Series, volume 887, page
012089. IOP Publishing.
Da Costa, V. G. T., Fini, E., Nabi, M., Sebe, N., and Ricci,
E. (2022). solo-learn: A library of self-supervised
methods for visual representation learning. Journal
of Machine Learning Research, 23(56):1–6.
Deng, J., Dong, W., Socher, R., Li, L.-J., Li, K., and Fei-
Fei, L. (2009). Imagenet: A large-scale hierarchical
image database. In 2009 IEEE conference on com-
puter vision and pattern recognition, pages 248–255.
Ieee.
Dosovitskiy, A., Springenberg, J. T., Riedmiller, M., and
Brox, T. (2014). Discriminative unsupervised fea-
ture learning with convolutional neural networks. Ad-
vances in neural information processing systems, 27.
Dwibedi, D., Aytar, Y., Tompson, J., Sermanet, P., and Zis-
serman, A. (2021). With a little help from my friends:
Nearest-neighbor contrastive learning of visual repre-
sentations. In Proceedings of the IEEE/CVF Interna-
tional Conference on Computer Vision, pages 9588–
9597.
Grill, J.-B., Strub, F., Altch
´
e, F., Tallec, C., Richemond, P.,
Buchatskaya, E., Doersch, C., Avila Pires, B., Guo,
Z., Gheshlaghi Azar, M., et al. (2020). Bootstrap your
own latent-a new approach to self-supervised learn-
ing. Advances in neural information processing sys-
tems, 33:21271–21284.
Guojian, C., Wei, M., Xinshan, W., Chunlong, R., and Junx-
iang, N. (2013). Research of rock texture identifica-
tion based on image processing and neural network. J
Xi’an Shiyou Univ (Nat Sci Edition).
He, K., Fan, H., Wu, Y., Xie, S., and Girshick, R. (2020).
Momentum contrast for unsupervised visual represen-
tation learning. In Proceedings of the IEEE/CVF con-
ference on computer vision and pattern recognition,
pages 9729–9738.
Huang, J., Kong, X., and Zhang, X. (2022). Revisiting the
critical factors of augmentation-invariant representa-
tion learning. In European Conference on Computer
Vision, pages 42–58. Springer.
Jaiswal, A., Babu, A. R., Zadeh, M. Z., Banerjee, D., and
Makedon, F. (2020). A survey on contrastive self-
supervised learning. Technologies, 9(1):2.
Karimpouli, S. and Tahmasebi, P. (2019). Segmentation of
digital rock images using deep convolutional autoen-
coder networks. Computers & geosciences, 126:142–
150.
Liu, Y., Zhang, Z., Liu, X., Wang, L., and Xia, X. (2021).
Ore image classification based on small deep learning
model: Evaluation and optimization of model depth,
model structure and data size. Minerals Engineering,
172:107020.
Misra, I. and Maaten, L. v. d. (2020). Self-supervised learn-
ing of pretext-invariant representations. In Proceed-
ings of the IEEE/CVF conference on computer vision
and pattern recognition, pages 6707–6717.
Oord, A. v. d., Li, Y., and Vinyals, O. (2018). Representa-
tion learning with contrastive predictive coding. arXiv
preprint arXiv:1807.03748.
Shim, M. S., Thiele, C., Vila, J., Saxena, N., and Hohl, D.
(2023). Content-based image retrieval for industrial
material images with deep learning and encoded phys-
ical properties. Data-Centric Engineering, 4:e21.
VISAPP 2024 - 19th International Conference on Computer Vision Theory and Applications
400