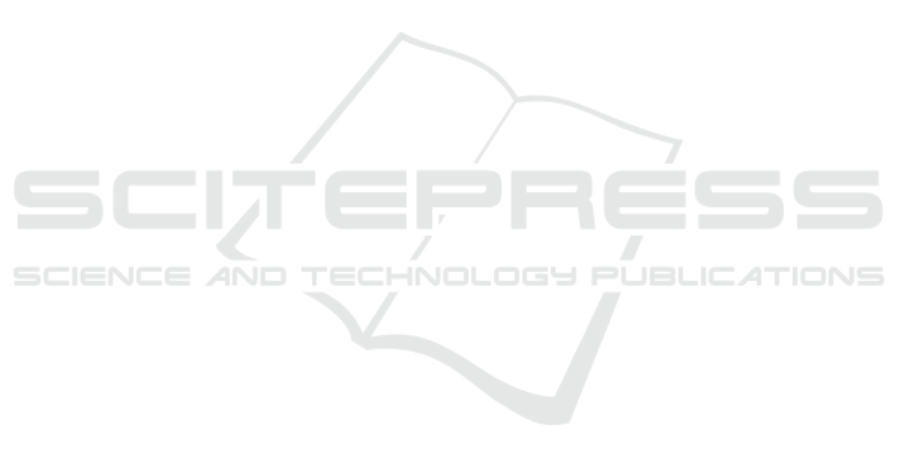
in generalizing to unseen data. These shortcomings
manifested in consistent overestimations and skewed
predictions, highlighting potential pitfalls for its ap-
plication in complex real-world settings.
In contrast, the GENUINE model emerged as
more versatile and resilient. Its commendable ro-
bustness against label noise and consistent perfor-
mance across diverse data distributions were partic-
ularly noteworthy. The model demonstrated its abil-
ity to tackle the challenges posed by induced label
noise and showcased its ability to underpin even in
the presence of varied and complex data inputs. The
GENUINE model’s adaptability was further affirmed
by its organized representation of artificially created
nuclei in the feature space, elucidating its capabilities
in robust representation learning and interpretability.
Moreover, the visual analyses and the exploration
of the embedding space organization provided invalu-
able insights into the inner workings of the GEN-
UINE network. The observed organization in the fea-
ture space, indicative of the model’s ability to discern
subtle differences in input and map similar features
closely, reinforced GENUINE’s potential as a power-
ful tool in FISH classification tasks. The clear and
consistent mapping of features, even under variations
in singal size and number, confirmed the model’s ca-
pacity to build meaningful and robust representations,
highlighting its utility in complex scenarios.
In the future, deepening the development of
leveraging unlabeled data and uncertainty assess-
ment methods will help improve the reliability and
adaptability of the model in diagnostic environments,
thereby promoting more harmonious and effective in-
tegration with human interventions in medical diag-
noses.
REFERENCES
Bahry, E., Breimann, L., Zouinkhi, M., Epstein, L., Koly-
vanov, K., Long, X., Harrington, K. I. S., Lionnet, T.,
and Preibisch, S. (2021). Rs-fish: Precise, interactive,
fast, and scalable fish spot detection. bioRxiv, page
2021.03.09.434205.
Bouilhol, E., Lefevre, E., Dartigues, B., Brackin, R.,
Savulescu, A. F., and Nikolski, M. (2021). Deepspot:
a deep neural network for rna spot enhancement in sm-
fish microscopy images.
Chrzanowska, N. M., Kowalewski, J., and Lewandowska,
M. A. (2020). Use of fluorescence in situ hybridiza-
tion (fish) in diagnosis and tailored therapies in solid
tumors.
Cohn, S. L., Pearson, A. D. J., London, W. B., Monclair, T.,
Ambros, P. F., Brodeur, G. M., Faldum, A., Hero, B.,
Iehara, T., Machin, D., Mosseri, V., Simon, T., Gar-
aventa, A., Castel, V., and Matthay, K. K. (2009). The
international neuroblastoma risk group (inrg) classifi-
cation system: An inrg task force report. Journal of
Clinical Oncology.
Graf, F., Hofer, C. D., Niethammer, M., and Kwitt, R.
(2021). Dissecting supervised contrastive learning.
Gudla, P. R., Nakayama, K., Pegoraro, G., and Misteli,
T. (2017). Spotlearn: Convolutional neural network
for detection of fluorescence in situ hybridization
(fish) signals in high-throughput imaging approaches.
Cold Spring Harbor symposia on quantitative biol-
ogy, 82:57–70.
He, K., Zhang, X., Ren, S., and Sun, J. (2015). Deep resid-
ual learning for image recognition. Proceedings of the
IEEE Computer Society Conference on Computer Vi-
sion and Pattern Recognition, 2016-December:770–
778.
Huang, M. and Weiss, W. A. (2013). Neuroblastoma and
mycn. Cold Spring Harbor Perspectives in Medicine,
3.
Lin, T. Y., Goyal, P., Girshick, R., He, K., and Dollar, P.
(2017). Focal loss for dense object detection. IEEE
Transactions on Pattern Analysis and Machine Intel-
ligence, 42:318–327.
Litjens, G., Kooi, T., Bejnordi, B. E., Setio, A. A. A.,
Ciompi, F., Ghafoorian, M., van der Laak, J. A., van
Ginneken, B., and S
´
anchez, C. I. (2017). A survey
on deep learning in medical image analysis. Medical
Image Analysis, 42:60–88.
Mathew, P., Valentine, M. B., Bowman, L. C., Rowe, S. T.,
Nash, M. B., Valentine, V. A., Cohn, S. L., Castle-
berry, R. P., Brodeur, G. M., and Look, A. T. (2001).
Detection of mycn gene amplification in neuroblas-
toma by fluorescence in situ hybridization: a pediatric
oncology group study. Neoplasia (New York, N.Y.),
3:105–109.
McInnes, L., Healy, J., and Melville, J. (2020). Umap:
Uniform manifold approximation and projection for
dimension reduction. arXiv:1802.03426 [cs, stat].
Comment: Reference implementation available at
http://github.com/lmcinnes/umap.
Otte, J., Dyberg, C., Pepich, A., and Johnsen, J. I. (2021).
Mycn function in neuroblastoma development.
Pinkel, D., Straume, T., and Gray, J. W. (1986). Cytogenetic
analysis using quantitative, high-sensitivity, fluores-
cence hybridization (in situ hybridization/biotin label-
ing/hybrid cells/chromosome-specific staining).
Sadr, A. V., Vos, E. E., Bassett, B. A., Hosenie, Z., Oozeer,
N., and Lochner, M. (2018). Deepsource: Point source
detection using deep learning.
Stringer, C., Michaelos, M., and Pachitariu, M. (2021).
Cellpose: a generalist algorithm for cellular segmen-
tation. page 17.
Zakrzewski, F., de Back, W., Weigert, M., Wenke, T.,
Zeugner, S., Roeder, I., Aust, D., Baretton, G., and
H
¨
onscheid, P. (2019). Automated detection of the
her2 gene amplification status in fluorescence in situ
hybridization images for the diagnostics of cancer tis-
sues. Scientific Reports, 9.
ICPRAM 2024 - 13th International Conference on Pattern Recognition Applications and Methods
36