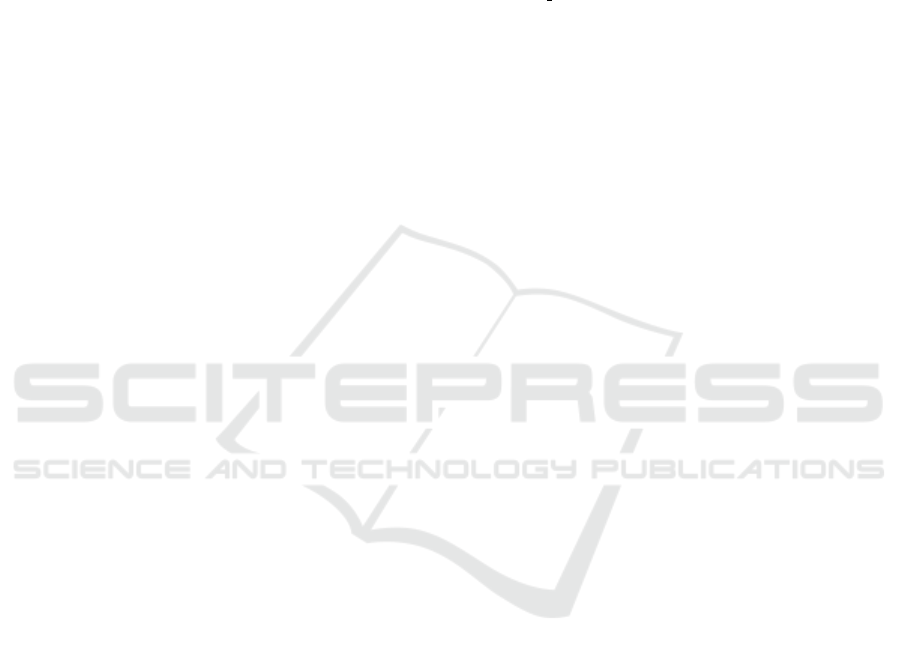
ducting a rather strong promotion (around 40%) at the
very beginning of the simulation, from time steps 0 to
10, increases the profits of Pack A. It is noteworthy
that there are no other promotions on packs B and C
at this specific moment. Finally, the model demon-
strates that it is necessary to conduct at least a second
promotion later in the simulation, often just after the
promotions of B and C, to prevent these promotions
from impacting the sales of A.
In conclusion, our experimental findings suggest
that, within the specified experimental conditions of
our model, it is more advantageous for the profits gen-
erated by pack A to schedule its promotions indepen-
dently of those for packs B and C and to use the pro-
motion sparingly.
6 DISCUSSION
In this paper, we show in section 4 that the agent-
based approach proposed in section 3 is able to repro-
duce emerging phenomena known in marketing such
as the increase in sales volume, the ”cannibalization”
linked to competition or the changes in customers be-
havior caused by the rapid repetition of promotions.
Moreover, the individual-centered approach allows us
to show phenomena that are only observable at the in-
dividual level, such as loyalty during a promotion or
the effects of price wars directly on consumers. We
show the model’s ability to reproduce general stylized
marketing facts and to adapt to different scenarios. In
this way, we propose a form of learning that allows
us to start from a known scenario, and run a complete
simulation of different scenarios that we would like to
explore. Scenario exploration is facilitated by access
to simulated data similar to real data (sales receipts).
In order to deepen the model, it is possible to add
a system of social influence similar to those described
in the section 2, which would allow agents to ex-
change and interact with each other in order to in-
fluence each other. Moreover, it is possible to give
the agents only a partial knowledge of the products,
so the agents would have to discover themselves the
products they do not know or be socially influenced.
Finally, it would be interesting to study the notion of
similarity between products and to see, according to
this similarity, the competition generated and the ef-
fects of promotions.
The proposed model has the possibility to easily
integrate real data (via history) which would improve
the realism, and apply the model to concrete scenar-
ios. We justify its adaptability to the data through the
global parameters built into the model.
REFERENCES
Axtell, R. L. and Farmer, J. D. (2022). Agent-based mod-
eling in economics and finance: Past, present, and fu-
ture. Journal of Economic Literature.
Bawa, K. (1990). Modeling inertia and variety seeking ten-
dencies in brand choice behavior. Marketing science,
9(3):263–278.
Blattberg, R. C., Briesch, R., and Fox, E. J. (1995).
How promotions work. Marketing science,
14(3 supplement):G122–G132.
Borden, N. H. (1964). The concept of the marketing mix.
Journal of advertising research, 4(2):2–7.
Cohen, M. C., Gupta, S., Kalas, J. J., and Perakis, G. (2020).
An efficient algorithm for dynamic pricing using a
graphical representation. Production and Operations
Management, 29(10):2326–2349.
Delre, S. A., Jager, W., Bijmolt, T. H., and Janssen, M. A.
(2007). Targeting and timing promotional activities:
An agent-based model for the takeoff of new products.
Journal of business research, 60(8):826–835.
Hardie, B. G., Johnson, E. J., and Fader, P. S. (1993). Mod-
eling loss aversion and reference dependence effects
on brand choice. Marketing science, 12(4):378–394.
Hung, P. D., Ngoc, N. D., and Hanh, T. D. (2019). K-means
clustering using ra case study of market segmentation.
In Proceedings of the 2019 5th International Confer-
ence on E-Business and Applications, pages 100–104.
Jager, W. (2007). The four p’s in social simulation, a per-
spective on how marketing could benefit from the use
of social simulation. Journal of Business Research,
60(8):868–875.
Negahban, A. and Yilmaz, L. (2014). Agent-based simula-
tion applications in marketing research: an integrated
review. Journal of Simulation, 8(2):129–142.
Said, L. B., Bouron, T., and Drogoul, A. (2002). Agent-
based interaction analysis of consumer behavior. In
Proceedings of the first international joint conference
on Autonomous agents and multiagent systems: part
1, pages 184–190.
Seetharaman, P. and Chintagunta, P. (1998). A model
of inertia and variety-seeking with marketing vari-
ables. International Journal of Research in Market-
ing, 15(1):1–17.
Tellis, G. J. (2006). Modeling marketing mix. Handbook of
marketing research, pages 506–522.
Wigren, R. and Cornell, F. (2019). Marketing mix mod-
elling: A comparative study of statistical models.
Simulation of Consumers Behavior Facing Discounts and Promotions
267