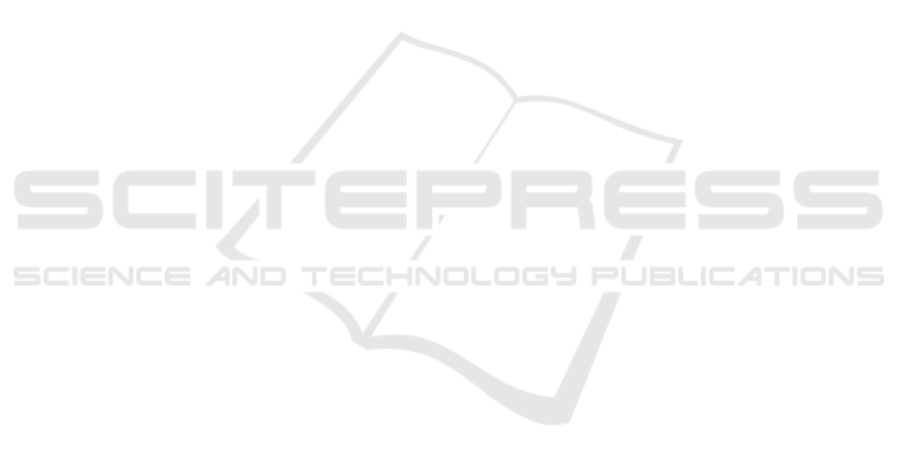
sidered architecture. It is clear that considering other
data related to genetic factors or lifestyle can intro-
duce some context to the network that could improve
considerably our results.
ACKNOWLEDGMENT
This work has been partly supported by the
Spanish Research Agency, grant number PID2019-
106623RB-C41/AEI/10.13039/501100011033 (Big
Theory), PID2022-136887NB-I00 (POLIGRAPH)
and by the European Union NextGeneration-EU
funds (Youth Employment Plan of the Spanish Gov-
ernment) in the INVESTIGO project with reference
URJC-AI-11.
REFERENCES
Anderson, G. and Horvath, J. (2004). The growing burden
of chronic disease in america. Public Health Reports,
119(3):263–70.
Ansah, J. P. and Chiu, C.-T. (2023). Projecting the chronic
disease burden among the adult population in the
united states using a multi-state population model.
Frontiers in Public Health, 10:1082183.
Barwal, R. K. and Raheja, N. (2022). Comparative analy-
sis of recommendation systems based on medical data
sets. In Intl Conf on Advances in Computing, Comm.
Control and Networking, pages 739–46. IEEE.
Bishop, C. M. (2010). Pattern Recognition and Machine
Learning. Springer.
Boyd, C. M. and Fortin, M. (2010). Future of multimorbid-
ity research: how should understanding of multimor-
bidity inform health system design? Public Health
Reviews, 32(2):451–74.
Brennan, P. and et al (2017). Chronic disease research in
europe and the need for integrated population cohorts.
European Journal of Epidemiology, 32:741–49.
Care, D. (2019). Standards of medical care in diabetes 2019.
Diabetes Care, 42(Suppl 1):S124–38.
Cho, K. and et al. (2014). Learning phrase representations
using RNN encoder-decoder for statistical machine
translation. arXiv preprint arXiv:1406.1078.
Chushig, D., Soguero, C., de Miguel, P., and Mora, I.
(2021). Interpreting clinical latent representations us-
ing autoencoders and probabilistic models. Artificial
Intelligence in Medicine, 122:102211.
Chushig, D., Soguero, C., de Miguel, P., and Mora, I.
(2022). Learning and visualizing chronic latent rep-
resentations using electronic health records. BioData
Mining, 15(1):1–27.
Chushig, D., Soguero, C., Engelbrecht, A., de Miguel, P.,
and Mora, I. (2020). Data-driven visual characteri-
zation of patient health-status using electronic health
records and self-organizing maps. IEEE, 8:137019–
31.
Hochreiter, S. and Schmidhuber, J. (1997). Long Short-
Term Memory. Neural Computation, 9(8):1735–80.
Hoffman, J. M. and et al (2012). Projecting future drug
expenditures—2012. American Journal of Health-
System Pharmacy, 69(5):405–21.
Hughes, J. S., Averill, R. F., et al. (2004). Clinical risk
groups (crgs): a classification system for risk-adjusted
capitation-based payment and health care manage-
ment. Medical Care, pages 81–90.
Jordan, I. D., Sok
´
oł, P. A., and Park, I. M. (2021). Gated
recurrent units viewed through the lens of continuous
time dynamical systems. Frontiers in Computational
Neuroscience, 15:678158.
Jurado, T., Chushig, D., Soguero, C., de Miguel, P., and
Mora, I. (2023). On the use of generative adversarial
networks to predict health status among chronic pa-
tients. In Intl Conf Health Informatics, pages 167–78.
Kingma, D. P. and Ba, J. L. (2015). Adam : A method
for stochastic optimization. In Intl Conf on Learning
Representations.
Levin, E. (1990). A recurrent neural network: Limitations
and training. Neural Networks, 3(6):641–50.
Liang, Y. and Guo, C. (2023). Heart failure disease predic-
tion and stratification with temporal electronic health
records data using patient representation. Biocyber-
netics and Biomedical Engineering, 43(1):124–41.
Miotto, R. and et al (2016). Deep patient: an unsupervised
representation to predict the future of patients from the
electronic health records. Scientific Reports, 6(1):1–
10.
Muraki, R. and et al (2022). Automated detection scheme
for acute myocardial infarction using convolutional
neural network and long short-term memory. PLOS
ONE, 17(2):1–22.
Pawlson, L. G. (1994). Chronic illness: implications of a
new paradigm for health care. The Joint Commission
Journal on Quality Improvement, 20(1):33–39.
Petrides, J. and et al (2019). Lifestyle changes for disease
prevention. Primary Care: Clinics in Office Practice,
46(1):1–12.
Qiao, L. and et al (2003). Supporting sliding window
queries for continuous data streams. In Intl Conf
on Scientific and Statistical Database Management,
pages 85–94. IEEE.
Ronning, M. (2002). A historical overview of the atc/ddd
methodology. World Health Organization Drug Infor-
mation, 16(3):233.
Soguero, C., Alonso, N., Mu
˜
noz, S., Rojo, J. L., Rubio, M.,
Caballero, I., and Mora, I. (2020). Finding associa-
tions among chronic conditions by bootstrap and mul-
tiple correspondence analysis. In Intl Conf on Bioin-
formatics and Biomedicine, pages 2066–73. IEEE.
Soguero, C., de Miguel, P., and Mora, I. (2016). A data-
driven model based on support vector machine to
identify chronic hypertensive and diabetic patients. In
Phys. Computing Systems, pages 110–29. Springer.
Wang, H. and Wang, L. (2017). Modeling temporal dynam-
ics and spatial configurations of actions using two-
stream recurrent neural networks. In IEEE Conf on
Computer Vis. and Pattern Recogn., pages 499–508.
HEALTHINF 2024 - 17th International Conference on Health Informatics
44