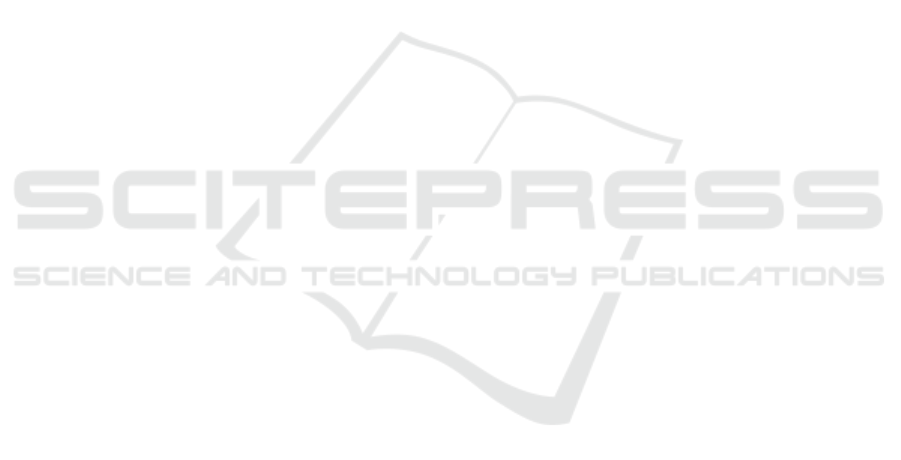
of the predictions of semantic segmentation models.
This information is valuable for guiding future re-
search and clinical applications aimed at improving
the diagnosis and treatment of this important cancer
pathology.
ACKNOWLEDGEMENTS
The work presented in this paper was supported by
the Pesquisa Aplicada em Vis
˜
ao e Intelig
ˆ
encia Com-
putacional (PAVIC) project at Universidade Federal
do Acre, Brazil.
REFERENCES
Al-Dhabyani, W., Gomaa, M., Khaled, H., and Fahmy, A.
(2020). Dataset of breast ultrasound images. Data in
brief, 28:104863.
Athira, K., Dharmarajan, J. P., Vijaykumar, D., and Sub-
banna, N. (2023). Analysis of the various techniques
used for breast segmentation from mammograms. In
2023 International Conference on Distributed Com-
puting and Electrical Circuits and Electronics (ICD-
CECE), pages 1–7. IEEE.
Ayana, G., Dese, K., Raj, H., Krishnamoorthy, J., and Kwa,
T. (2022). De-speckling breast cancer ultrasound
images using a rotationally invariant block matching
based non-local means (ribm-nlm) method. Diagnos-
tics, 12(4):862.
Badrinarayanan, V., Kendall, A., and Cipolla, R. (2017).
Segnet: A deep convolutional encoder-decoder ar-
chitecture for image segmentation. IEEE transac-
tions on pattern analysis and machine intelligence,
39(12):2481–2495.
Bishop, C. M. and Nasrabadi, N. M. (2006). Pattern recog-
nition and machine learning, volume 4. Springer.
Byra, M., Jarosik, P., Szubert, A., Galperin, M., Ojeda-
Fournier, H., Olson, L., O’Boyle, M., Comstock, C.,
and Andre, M. (2020). Breast mass segmentation in
ultrasound with selective kernel u-net convolutional
neural network. Biomedical Signal Processing and
Control, 61:102027.
Chen, L.-C., Papandreou, G., Kokkinos, I., Murphy, K., and
Yuille, A. L. (2017). Deeplab: Semantic image seg-
mentation with deep convolutional nets, atrous convo-
lution, and fully connected crfs. IEEE transactions on
pattern analysis and machine intelligence, 40(4):834–
848.
Cui, W., Meng, D., Lu, K., Wu, Y., Pan, Z., Li, X., and Sun,
S. (2023). Automatic segmentation of ultrasound im-
ages using segnet and local nakagami distribution fit-
ting model. Biomedical Signal Processing and Con-
trol, 81:104431.
Doi, K. (2007). Computer-aided diagnosis in medical imag-
ing: historical review, current status and future po-
tential. Computerized medical imaging and graphics,
31(4-5):198–211.
Gharekhanloo, F., Haseli, M. M., and Torabian, S. (2018).
Value of ultrasound in the detection of benign and ma-
lignant breast diseases: a diagnostic accuracy study.
Oman Medical Journal, 33(5):380.
Gokhale, S. (2009). Ultrasound characterization of breast
masses. Indian Journal of Radiology and Imaging,
19(03):242–247.
Jahwar, A. F. and Abdulazeez, A. M. (2022). Segmen-
tation and classification for breast cancer ultrasound
images using deep learning techniques: a review. In
2022 IEEE 18th International Colloquium on Signal
Processing & Applications (CSPA), pages 225–230.
IEEE.
Liu, M., Fu, B., Xie, S., He, H., Lan, F., Li, Y., Lou,
P., and Fan, D. (2021). Comparison of multi-source
satellite images for classifying marsh vegetation using
deeplabv3 plus deep learning algorithm. Ecological
Indicators, 125:107562.
Ostertagova, E., Ostertag, O., and Kov
´
a
ˇ
c, J. (2014).
Methodology and application of the kruskal-wallis
test. Applied mechanics and materials, 611:115–120.
Ploquin, M., Basarab, A., and Kouame, D. (2015). Res-
olution enhancement in medical ultrasound imaging.
Journal of Medical Imaging, 2(1):171.
Ronneberger, O., Fischer, P., and Brox, T. (2015). U-
net: Convolutional networks for biomedical image
segmentation. In Medical Image Computing and
Computer-Assisted Intervention–MICCAI 2015: 18th
International Conference, Munich, Germany, October
5-9, 2015, Proceedings, Part III 18, pages 234–241.
Springer.
Sun, Y., Yang, H., Zhou, J., and Wang, Y. (2022). Issmf:
Integrated semantic and spatial information of multi-
level features for automatic segmentation in prenatal
ultrasound images. Artificial Intelligence in Medicine,
125:102254.
Yu, K., Chen, S., and Chen, Y. (2021). Tumor segmenta-
tion in breast ultrasound image by means of res path
combined with dense connection neural network. Di-
agnostics, 11(9):1565.
Yurtkulu, S. C., S¸ahin, Y. H., and Unal, G. (2019). Se-
mantic segmentation with extended deeplabv3 archi-
tecture. In 2019 27th Signal Processing and Commu-
nications Applications Conference (SIU), pages 1–4.
IEEE.
Comparision Through Architectures of Semantic Segmentation in Breast Ultrasound Images Across Differents Input Data Dimensions
361