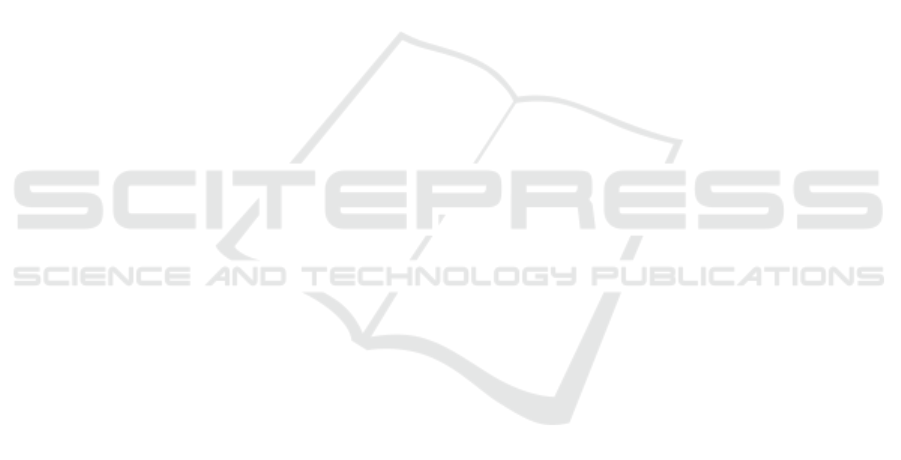
times throughout the day can be accurately inferred
in reality. Even if places like home or workplace
are known, only parts of the day might be inferred.
Therefore, it is a challenge to examine which meth-
ods are suitable for cases where only certain location
information is inferred in future work. Additionally,
we focused on inferring only location information in
this research, but it is conceivable that certain Wi-Fi
information can also be inferred. Exploring scenarios
where partial information of both location and Wi-Fi
is inferred is another future research direction.
REFERENCES
Abuhamad, M., Abusnaina, A., Nyang, D., and Mohaisen,
D. (2020). Sensor-based continuous authentication
of smartphones’ users using behavioral biometrics: A
contemporary survey. IEEE Internet of Things Jour-
nal, 8(1):65–84.
Chen, H., Li, F., Du, W., Yang, S., Conn, M., and Wang,
Y. (2020). Listen to your fingers: User authentication
based on geometry biometrics of touch gesture. Pro-
ceedings of the ACM on Interactive, Mobile, Wearable
and Ubiquitous Technologies, 4(3):1–23.
Corporation, M. Bing maps tile system. https://learn.micr
osoft.com/en-us/bingmaps/articles/bing-maps-tile-s
ystem. Accessed:2023-05-21.
Fridman, L., Weber, S., Greenstadt, R., and Kam, M.
(2016). Active authentication on mobile devices via
stylometry, application usage, web browsing, and gps
location. IEEE Systems Journal, 11(2):513–521.
Khan, H., Hengartner, U., and Vogel, D. (2016). Targeted
mimicry attacks on touch input based implicit authen-
tication schemes. In Proceedings of the 14th Annual
International Conference on Mobile Systems, Appli-
cations, and Services, pages 387–398.
Khan, H., Hengartner, U., and Vogel, D. (2018). Aug-
mented reality-based mimicry attacks on behaviour-
based smartphone authentication. In Proceedings of
the 16th Annual International Conference on Mobile
Systems, Applications, and Services, pages 41–53.
Kobayashi, R. and Yamaguchi, R. S. (2015). A behav-
ior authentication method using wi-fi bssids around
smartphone carried by a user. In 2015 Third Inter-
national Symposium on Computing and Networking
(CANDAR), pages 463–469. IEEE.
Kobayashi, R. and Yamaguchi, R. S. (2017). Behavioral au-
thentication method utilizing wi-fi history information
captured by iot device. In 2017 International Work-
shop on Secure Internet of Things (SIoT), pages 20–
29. IEEE.
Kumar, R., Phoha, V. V., and Jain, A. (2015). Tread-
mill attack on gait-based authentication systems. In
2015 IEEE 7th international conference on biomet-
rics theory, applications and systems (BTAS), pages
1–7. IEEE.
Liao, L., Fox, D., and Kautz, H. (2007). Extracting places
and activities from gps traces using hierarchical con-
ditional random fields. The International Journal of
Robotics Research, 26(1):119–134.
Miyazawa, A., Thao, T. P., and Yamaguchi, R. S. (2022).
Multi-factor behavioral authentication using correla-
tions enhanced by neural network-based score fusion.
In 2022 IEEE 19th Annual Consumer Communica-
tions & Networking Conference (CCNC), pages 569–
577. IEEE.
Rattani, A., Freni, B., Marcialis, G. L., and Roli, F. (2009).
Template update methods in adaptive biometric sys-
tems: A critical review. In Advances in Biometrics:
Third International Conference, ICB 2009, Alghero,
Italy, June 2-5, 2009. Proceedings 3, pages 847–856.
Springer.
Raul, N., Shankarmani, R., and Joshi, P. (2020). A com-
prehensive review of keystroke dynamics-based au-
thentication mechanism. In International Conference
on Innovative Computing and Communications: Pro-
ceedings of ICICC 2019, Volume 2, pages 149–162.
Springer.
Rayani, P. K. and Changder, S. (2023). Continuous user
authentication on smartphone via behavioral biomet-
rics: a survey. Multimedia Tools and Applications,
82(2):1633–1667.
Sufyan, F., Sagar, S., Nayel, S., Chishti, M. S., Ashraf, Z.,
and Banerjee, A. (2023). A novel and lightweight real-
time continuous motion gesture recognition algorithm
for smartphones. IEEE Access.
Thao, T. P., Irvan, M., Kobayashi, R., Yamaguchi, R. S., and
Nakata, T. (2020). Self-enhancing gps-based authen-
tication using corresponding address. In Data and Ap-
plications Security and Privacy XXXIV: 34th Annual
IFIP WG 11.3 Conference, DBSec 2020, Regensburg,
Germany, June 25–26, 2020, Proceedings 34, pages
333–344. Springer.
Traore, I. (2011). Continuous Authentication Using Biomet-
rics: Data, Models, and Metrics: Data, Models, and
Metrics. Igi Global.
Yamaguchi, R. S., Nakata, T., and Kobayashi, R. (2020a).
Redefine and organize, 4th authentication factor, be-
havior. International Journal of Networking and Com-
puting, 10(2):189–199.
Yamaguchi, S., Gomi, H., Kobayashi, R., Thao, T. P., Irvan,
M., and Yamaguchi, R. S. (2020b). Effective classi-
fication for multi-modal behavioral authentication on
large-scale data. In 2020 15th Asia Joint Conference
on Information Security (AsiaJCIS), pages 101–109.
IEEE.
Zeng, Y., Pande, A., Zhu, J., and Mohapatra, P. (2017).
Wearia: Wearable device implicit authentication
based on activity information. In 2017 IEEE 18th In-
ternational Symposium on A World of Wireless, Mo-
bile and Multimedia Networks (WoWMoM), pages 1–
9. IEEE.
ICISSP 2024 - 10th International Conference on Information Systems Security and Privacy
716