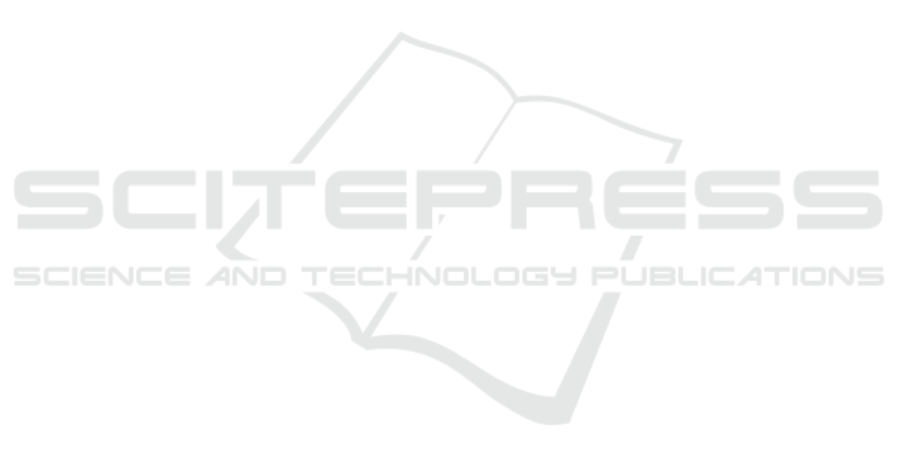
Finke, T., Kr
¨
amer, M., Morandini, A., M
¨
uck, A., and
Oleksiyuk, I. (2021). Autoencoders for unsupervised
anomaly detection in high energy physics. J. High En-
ergy Phys., 2021(6):161.
Fischer, A. and Igel, C. (2012). An introduction to restricted
boltzmann machines. In Iberoamerican congress on
pattern recognition, pages 14–36. Springer.
Gabri
´
e, M., Tramel, E. W., and Krzakala, F. (2015). Train-
ing restricted boltzmann machine via the thouless-
anderson-palmer free energy. Advances in neural in-
formation processing systems, 28.
Hangleiter, D. and Eisert, J. (2023). Computational advan-
tage of quantum random sampling.
Hinton, G. E. (2012). A practical guide to training restricted
boltzmann machines. In Neural networks: Tricks of
the trade, pages 599–619. Springer.
Hoh, M., Sch
¨
ottl, A., Schaub, H., and Wenninger, F. (2022).
A generative model for anomaly detection in time
series data. Procedia Computer Science, 200:629–
637. 3rd International Conference on Industry 4.0 and
Smart Manufacturing.
Kadowaki, T. and Nishimori, H. (1998). Quantum an-
nealing in the transverse ising model. Phys. Rev. E,
58:5355–5363.
Karami, A. (2018). An anomaly-based intrusion detection
system in presence of benign outliers with visualiza-
tion capabilities. Expert Systems with Applications,
108:36–60.
Kumar, V. (2005). Parallel and distributed computing
for cybersecurity. IEEE Distributed Systems Online,
6(10).
Lecun, Y., Chopra, S., Hadsell, R., Ranzato, M., and Huang,
F. (2006). A tutorial on energy-based learning. MIT
Press.
Luo, X., Jiang, Y., Wang, E., and Men, X. (2022). Anomaly
detection by using a combination of generative ad-
versarial networks and convolutional autoencoders.
EURASIP J. Adv. Signal Process., 2022(1):112.
Mascaro, S., Nicholso, A. E., and Korb, K. B. (2014).
Anomaly detection in vessel tracks using bayesian
networks. International Journal of Approximate Rea-
soning, 55(1, Part 1):84–98. Applications of Bayesian
Networks.
Muniyandi, A. P., Rajeswari, R., and Rajaram, R. (2012).
Network anomaly detection by cascading k-means
clustering and c4.5 decision tree algorithm. Proce-
dia Engineering, 30:174–182. International Confer-
ence on Communication Technology and System De-
sign 2011.
Ngairangbam, V. S., Spannowsky, M., and Takeuchi,
M. (2022). Anomaly detection in high-energy
physics using a quantum autoencoder. Phys. Rev. D,
105:095004.
Nishimori, H., Tsuda, J., and Knysh, S. (2015). Compar-
ative study of the performance of quantum annealing
and simulated annealing. Phys. Rev. E, 91:012104.
Pedregosa, F., Varoquaux, G., Gramfort, A., Michel, V.,
Thirion, B., Grisel, O., Blondel, M., Prettenhofer,
P., Weiss, R., Dubourg, V., Vanderplas, J., Passos,
A., Cournapeau, D., Brucher, M., Perrot, M., and
Duchesnay, E. (2011). Scikit-learn: Machine learning
in Python. Journal of Machine Learning Research,
12:2825–2830.
Sato, T., Ohzeki, M., and Tanaka, K. (2021). Assessment
of image generation by quantum annealer. Scientific
reports, 11(1):1–10.
Schuhmacher, J., Boggia, L., Belis, V., Puljak, E., Grossi,
M., Pierini, M., Vallecorsa, S., Tacchino, F., Barkout-
sos, P., and Tavernelli, I. (2023). Unravelling physics
beyond the standard model with classical and quantum
anomaly detection. arXiv preprint arXiv:2301.10787.
Schuman, D., S
¨
unkel, L., Altmann, P., Stein, J., Roch, C.,
Gabor, T., and Linnhoff-Popien, C. (2023). Towards
transfer learning for large-scale image classification
using annealing-based quantum boltzmann machines.
In 2023 IEEE International Conference on Quantum
Computing and Engineering (QCE), volume 02, pages
42–47.
Spence, C., Parra, L., and Sajda, P. (2001). Detection, syn-
thesis and compression in mammographic image anal-
ysis with a hierarchical image probability model. In
Proceedings IEEE Workshop on Mathematical Meth-
ods in Biomedical Image Analysis (MMBIA 2001),
pages 3–10.
Sussmann, H. (1988). Learning algorithms for boltzmann
machines. In Proceedings of the 27th IEEE Confer-
ence on Decision and Control, pages 786–791 vol.1.
Vyas, A., Roberts, L., Gupta, S., and Patel, R. (2022).
Anomaly detection using azure quantum qio (quan-
tum inspired optimization) platform. Technical re-
port, Mphasis Corporation, New York, NY, USA. Ac-
cessed: 2022-08-21.
Wiebe, N. and Wossnig, L. (2019). Generative training
of quantum boltzmann machines with hidden units.
arXiv preprint arXiv:1905.09902.
Yarkoni, S., Raponi, E., Schmitt, S., and B
¨
ack, T. (2021).
Quantum annealing for industry applications: Intro-
duction and review. arXiv preprint arXiv:2112.07491,
page 31.
Younes, L. (1996). Synchronous boltzmann machines can
be universal approximators. Applied Mathematics Let-
ters, 9(3):109–113.
Zhai, S., Cheng, Y., Lu, W., and Zhang, Z. (2016). Deep
structured energy based models for anomaly detec-
tion. In International conference on machine learning,
pages 1100–1109. PMLR.
Zhu, E. Y., Johri, S., Bacon, D., Esencan, M., Kim, J., Muir,
M., Murgai, N., Nguyen, J., Pisenti, N., Schouela,
A., Sosnova, K., and Wright, K. (2022). Genera-
tive quantum learning of joint probability distribution
functions. Phys. Rev. Res., 4:043092.
Exploring Unsupervised Anomaly Detection with Quantum Boltzmann Machines in Fraud Detection
185