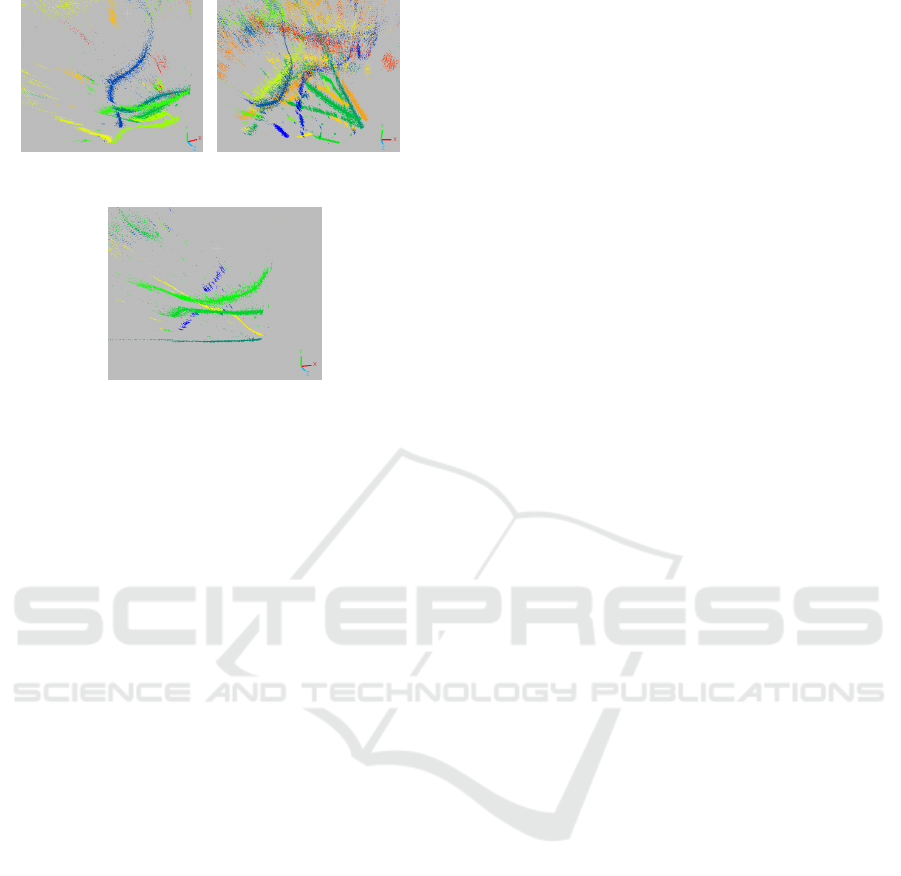
(a) 25 seconds of the first
recording.
(b) 90 seconds of the first
recording.
(c) 19 seconds of the second recording.
Figure 14: Trajectories of the first recording of a meadow
over 25 and 90 seconds and the second recording over 19
seconds.
6 CONCLUSIONS AND FUTURE
WORK
This paper presents the steps of the developed pro-
cessing pipeline from image acquisition to 4D display
for long-term insect monitoring with a stereo event
camera setup. Segmentation results have shown that
insect trajectories can be reliably separated from plant
movements. The use of histogram encoding gave the
best results. To improve the segmentation, besides
the improvement of the dataset a Siamese neural net-
work will be tested, which uses the same weights but
works in parallel on the two different input images to
obtain comparable segmentation results. This could
compensate for differences in segmentation quality
between the left and right camera images.
There are still some inaccuracies in the calcula-
tion of the 4D coordinates. These are caused by the
construction of the measurement system. The align-
ment of the two event cameras changed slightly due to
transport and heat, so that using the calibration data
resulted in an offset of up to 10 lines, depending on
the position of the events in the pixel matrix. A more
mechanically stable setup will be developed in the fu-
ture. For further interpretation of the data, the next
step will be to cluster the individual trajectories and
convert them to spline curves in order to obtain better
3D flight curves. Methods for trajectory tracking will
also be investigated in order to obtain longer sections
and to avoid double counting of insects. Finally, the
individual trajectories will be classified into different
insect groups based on the flight patterns as proposed
in (Pohle-Fr
¨
ohlich and Bolten, 2023).
REFERENCES
Bjerge, K., Mann, H. M., and Høye, T. T. (2022). Real-time
insect tracking and monitoring with computer vision
and deep learning. Remote Sensing in Ecology and
Conservation, 8(3):315–327.
Bolten, T., Lentzen, F., Pohle-Fr
¨
ohlich, R., and T
¨
onnies,
K. D. (2022a). Evaluation of deep learning based
3d-point-cloud processing techniques for semantic
segmentation of neuromorphic vision sensor event-
streams. In VISIGRAPP (4: VISAPP), pages 168–179.
Bolten, T., Pohle-Fr
¨
ohlich, R., and T
¨
onnies, K. (2023a). Se-
mantic Scene Filtering for Event Cameras in Long-
Term Outdoor Monitoring Scenarios. In Bebis, G.
et al., editors, 18th International Symposium on Vi-
sual Computing (ISVC), Advances in Visual Comput-
ing, volume 14362 of Lecture Notes in Computer Sci-
ence, pages 79–92, Cham. Springer Nature Switzer-
land.
Bolten, T., Pohle-Fr
¨
ohlich, R., and T
¨
onnies, K. D. (2023b).
Semantic segmentation on neuromorphic vision sen-
sor event-streams using pointnet++ and unet based
processing approaches. In VISIGRAPP (4: VISAPP),
pages 168–178.
Bolten, T., Pohle-Fr
¨
ohlich, R., Volker, D., Br
¨
uck, C.,
Beucker, N., and Hirsch, H.-G. (2022b). Visualiza-
tion of activity data from a sensor-based long-term
monitoring study at a playground. In VISIGRAPP (3:
IVAPP), pages 146–155.
Bradski, G. (2000). The OpenCV Library. Dr. Dobb’s Jour-
nal of Software Tools.
Dennis, R., Shreeve, T., Isaac, N., Roy, D., Hardy, P., Fox,
R., and Asher, J. (2006). The effects of visual ap-
parency on bias in butterfly recording and monitoring.
Biological conservation, 128(4):486–492.
Dong, S., Du, J., Jiao, L., Wang, F., Liu, K., Teng, Y.,
and Wang, R. (2022). Automatic crop pest detection
oriented multiscale feature fusion approach. Insects,
13(6):554.
Droissart, V., Azandi, L., Onguene, E. R., Savignac, M.,
Smith, T. B., and Deblauwe, V. (2021). Pict: A
low-cost, modular, open-source camera trap system to
study plant–insect interactions. Methods in Ecology
and Evolution, 12(8):1389–1396.
Gallego, G., Delbr
¨
uck, T., Orchard, G., Bartolozzi, C.,
Taba, B., Censi, A., Leutenegger, S., Davison, A. J.,
Conradt, J., Daniilidis, K., et al. (2020). Event-based
vision: A survey. IEEE transactions on pattern anal-
ysis and machine intelligence, 44(1):154–180.
Hallmann, C. A., Sorg, M., Jongejans, E., Siepel, H.,
Hofland, N., Schwan, H., Stenmans, W., M
¨
uller,
A., Sumser, H., H
¨
orren, T., et al. (2017). More
than 75 percent decline over 27 years in total fly-
ing insect biomass in protected areas. PloS one,
12(10):e0185809.
Stereo-Event-Camera-Technique for Insect Monitoring
383