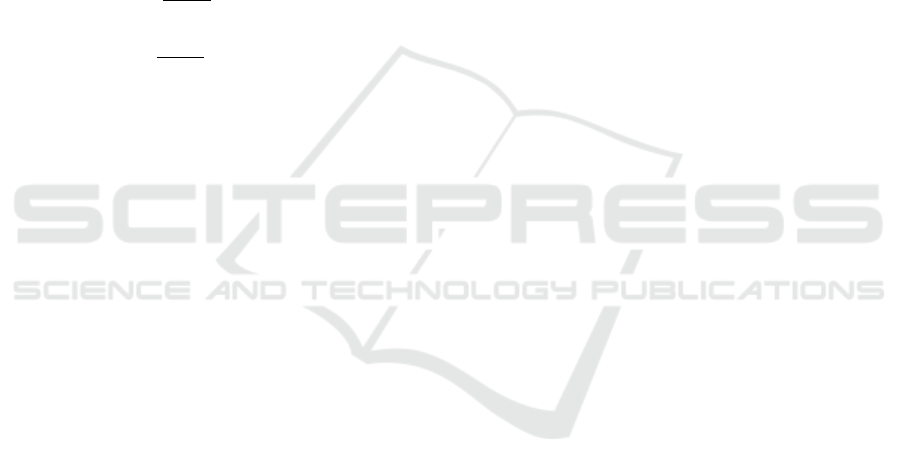
attribute m.”. For a set of objects A ⊆ G, the set of
common attributes for the objects. of A is denoted by.
A :=
{
m ∈ M|∀g ∈ A : (g, m) ∈ I
}
, similarly, the set of
ommon attributes for the objects of B is denoted by
B :=
{
g ∈ G|∀m ∈ B : (g, m) ∈ I
}
.
A formal concept of a formal context K =
(G, M, I) is defined by pair (A, B) where A is called
extension and B is called intention. For a pair (A, B)
to be considered a concept, one needs to follow the
condition where. (A = B
′
) and (B = A
′
). The set of
formal concepts of a context K is said to be β (K).
Association rules are dependencies between elements
of a formal context.
The rule A → B is valid only if for every object
containing attributes B, it also contains attributes from
C. Given a rule r and parameters s and c, one can
denote:
s = suppr (r) =
|
A
′
∩B
′
|
|
G
′
|
- called the support of rule
r, and
c = con f (r) =
|
A
′
∩B
′
|
|
A
′
|
- called confidence.
When con f (r) = 100% the rule is referred to as
an implication. (Felde and Stumme, 2023)
Studies that explore domains that can be repre-
sented as a binary tabular base of objects and at-
tributes can often apply FCA. Longitudinal study ap-
proaches aim to investigate a sample of individuals
with certain characteristics over consecutive time pe-
riods, referred to as waves. On the other hand, FCA
is an approach in formal set theory that focuses on the
representation and analysis of the semantic structure
of data at a single point in time, without considering
evolution or changes over time.
FCA is based on the idea that concepts can be de-
fined based on the relationships between objects and
attributes, enabling the creation of conceptual hierar-
chies and the understanding of associations between
meaningful terms. It is a useful technique for orga-
nizing and extracting information from data sets.
The dimensionality of a database is a crucial point
when attempting to generalize and find relationships
within data. A low-dimensional database is one that
contains few samples from a specific domain. For
example, databases related to human behavior often
have low dimensionality when we want to analyze be-
havior within a certain population. One application
of FCA is to understand how the objects present in
these low-dimensional databases, along with their at-
tributes, can have implications.
2.2 Aggressive Behaviors in Traffic
Behaviors in traffic that can lead to accidents are char-
acterized into three main categories: 1) aggressive be-
haviors in traffic, 2) influence of friends and close ac-
quaintances, 3) family influence. These categories en-
compass a fourth one that is used as a threshold for
analysis, which is socio-cultural information about
the drivers. The information pertains to 1039 Chi-
nese drivers whose sociocultural factors were asso-
ciated with these behaviors. The database was col-
lected through an online survey, publicly available in
the Data in Brief journal. This study was published
in August 2023 and has the Bayesian Mindsponge
Framework (BMF) as a validation index, specifically
showing how safe driving behaviors are affected by
information that promotes safe driving, actively ab-
sorbed with the support of friends/colleagues and/or
the driver’s family.
A fundamental concept of the Mindsponge Theory
is that the human mind tends to be influenced by infor-
mation absorbed from external sources. As analyzed
in (Jin et al., 2023), the factors that contribute to safe
driving may be related to external factors from family,
friends, and/or colleagues. These factors, along with
socio-cultural factors, provide interesting information
to be analyzed in this issue.
The application of FCA to the dataset in question
can provide important insights into the behavior of
drivers in traffic leading to accidents. Rules of the
form A → B take into account that when drivers ex-
hibit a certain aggressive behavior A, it implies B.
2.3 Lattice Miner
The Lattice Miner 2.0 tool is a data mining prototype
developed under the supervision of Professor Rokia
Missaoui by the laboratory of the University of Que-
bec. This is a publicly available Java platform in
which the main functions include all low-level opera-
tions that allow the manipulation of input data, struc-
tures, and rule association. The platform enables the
generation of groups, called formal concepts, includ-
ing logical implications, thereby showing binary rela-
tionships between collections of objects and their sets
of attributes or properties.
3 RELATED WORKS
This work utilizes Formal Concept Analysis, and the
approach is justified through a relationship between
theoretical and practical knowledge of this subject.
Related works on this topic are presented below.
(Wei et al., 2018) analyzes the triadic approach of
formal concept analysis in four aspects: (i) the basic
approach of triadic concept analysis, (ii) triadic im-
plications and rules, (iii) the triadic factor of analysis,
Application of Formal Concept Analysis to Characterize Driving Behaviors and Socio-Cultural Factors Related to Driving
57