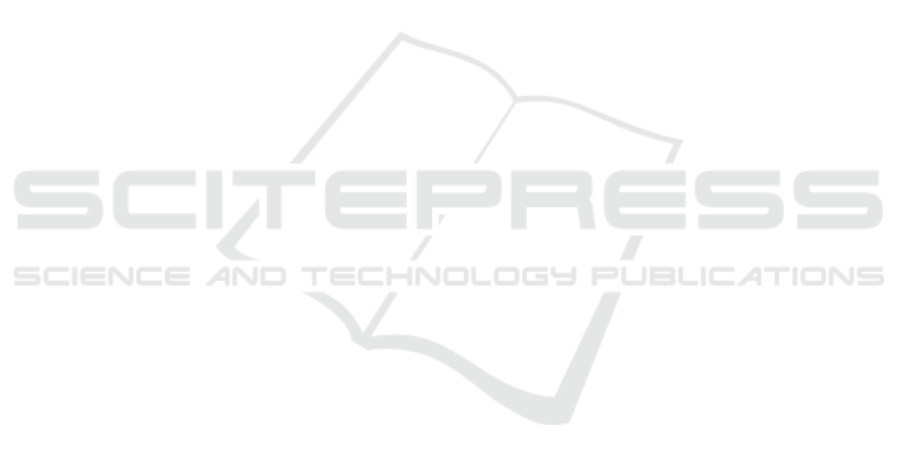
ACKNOWLEDGEMENTS
This work is supported by the International Research
Center “Innovation Transportation and Production
Systems” of the I-SITE CAP 20−25. Financial sup-
port was also received from the Auvergne-Rh
ˆ
one-
Alpes region through the ACCROBOT project (AC-
Costage haute pr
´
ecision ROBOTis
´
e − Chantier Tran-
sitique du laboratoire FACTOLAB) as part of the Pack
Ambition Recherche 2020.
REFERENCES
Aijazi, A. K., Checchin, P., and Trassoudaine, L. (2013).
Automatic removal of imperfections and change de-
tection for accurate 3d urban cartography by classi-
fication and incremental updating. Remote Sensing,
5(8):3701–3728.
Aijazi, A. K., Malaterre, L., Trassoudaine, L., Chateau, T.,
and Checchin, P. (2019). Automatic Detection and
Modeling of Underground Pipes Using a Portable 3D
LiDAR System. Sensors, 19(24).
Besl, P. and McKay, N. (1992). A Method for Registration
of 3-D Shapes. IEEE Trans. on PAMI, 14(2):239–256.
Brazeal, R. G., Wilkinson, B. E., and Hochmair, H. H.
(2021). A Rigorous Observation Model for the Ris-
ley Prism-Based Livox Mid-40 Lidar Sensor. Sensors,
21(14).
Choy, C., Dong, W., and Koltun, V. (2020). Deep Global
Registration. In Proceedings of the IEEE Computer
Society Conference on Computer Vision and Pattern
Recognition, pages 2511–2520.
He, Y., Ma, L., Jiang, Z., Tang, Y., and Xing, G. (2021).
VI-Eye: Semantic-Based 3D Point Cloud Registra-
tion for Infrastructure-Assisted Autonomous Driving.
In Proceedings of the 27th Annual International Con-
ference on Mobile Computing and Networking, Mobi-
Com ’21, page 573–586, New York, NY, USA.
Klasing, K., Althoff, D., Wollherr, D., and Buss, M. (2009).
Comparison of surface normal estimation methods for
range sensing applications. In 2009 IEEE Interna-
tional Conference on Robotics and Automation, pages
3206–3211.
Li, H., Zhao, H., Ye, B., and Zhang, Y. (2023). 3D semantic
map construction based on point cloud and image fu-
sion. IET Cyber-Systems and Robotics, 5(1):e12078.
Pais, G. D., Ramalingam, S., Govindu, V. M., Nascimento,
J. C., Chellappa, R., and Miraldo, P. (2019). 3DReg-
Net: A Deep Neural Network for 3D Point Registra-
tion. In Proceedings of the IEEE Computer Society
Conference on Computer Vision and Pattern Recogni-
tion, pages 7191–7201.
Rusu, R. B., Blodow, N., and Beetz, M. (2009). Fast Point
Feature Histograms (FPFH) for 3D registration. In
IEEE International Conference on Robotics and Au-
tomation, pages 3212–3217.
Rusu, R. B. and Cousins, S. (2011). 3D is here: Point Cloud
Library (PCL). In IEEE International Conference on
Robotics and Automation (ICRA), pages 1 – 4.
Segal, A., Haehnel, D., and Thrun, S. (2009). Generalized-
ICP. In Robotics: Science and Systems, volume 5,
pages 168–176.
Serafin, J. and Grisetti, G. (2015). NICP: Dense normal
based point cloud registration. In IEEE International
Conference on Intelligent Robots and Systems, vol-
ume 2015-Decem, pages 742–749.
Tazir, M. L., Gokhool, T., Checchin, P., Malaterre, L., and
Trassoudaine, L. (2018). CICP: Cluster Iterative Clos-
est Point for sparse-dense point cloud registration.
Robotics Auton. Syst., 108:66–86.
Wang, Y., Bu, S., Chen, L., Dong, Y., Li, K., Cao, X.,
and Li, K. (2023). HybridFusion: LiDAR and Vision
Cross-Source Point Cloud Fusion.
Wang, Z., Wang, X., Fang, B., Yu, K., and Ma, J. (2021).
Vehicle detection based on point cloud intensity and
distance clustering. Journal of Physics: Conference
Series, 1748(4):042053.
Xie, Z., Liang, P., Tao, J., Zeng, L., Zhao, Z., Cheng, X.,
Zhang, J., and Zhang, C. (2022). An Improved Super-
voxel Clustering Algorithm of 3D Point Clouds for the
Localization of Industrial Robots. Electronics, 11(10).
Xue, G., Wei, J., Li, R., and Cheng, J. (2022). LeGO-
LOAM-SC: An Improved Simultaneous Localization
and Mapping Method Fusing LeGO-LOAM and Scan
Context for Underground Coalmine. Sensors, 22(2).
Zou, Z., Lang, H., Lou, Y., and Lu, J. (2023). Plane-based
global registration for pavement 3D reconstruction us-
ing hybrid solid-state LiDAR point cloud. Automation
in Construction, 152:104907.
ICPRAM 2024 - 13th International Conference on Pattern Recognition Applications and Methods
686