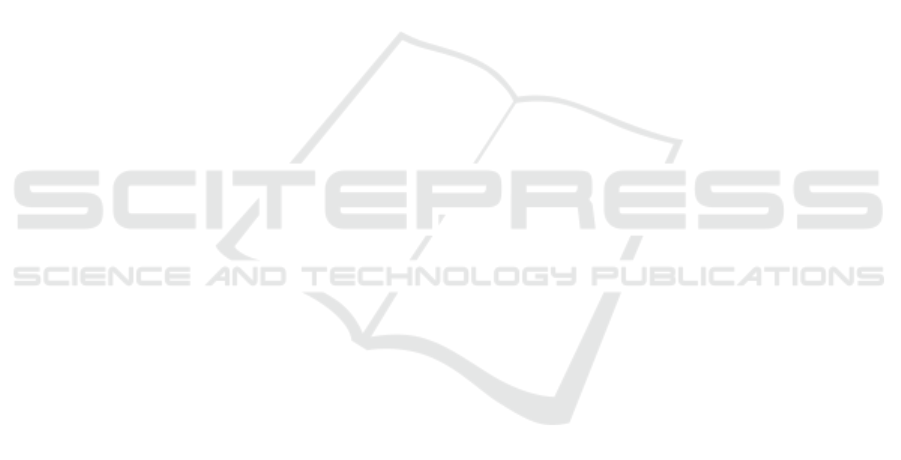
tention (LCA), i.e. mac, at each level of Feature Pyra-
mid Network (FPN), MAC overcomes the great scale
variation and complex background. As a result, nu-
meric and visualization results demonstrate MAC can
output accurate predictions on both very tiny and ex-
tremely large objects, especially on the ambiguous
boundary part. Extensive experiments shows the ef-
fectiveness and state-of-the-art performance of MAC.
6 DISCUSSIONS
With the triumph achieved by current deep learning
and machine learning methods, human can extract
the important geo-spatial information from aerial im-
age. In addition, most of the methods are trained and
tested in a single domain, i.e., clear weather with ad-
equate illumination. Specifically, iSAID (Waqas Za-
mir et al., 2019) and ISPRS datasets are leveraged in
this work, in which the majority of the data (high-
resolution RGB images) is under the aforementioned
comfortable condition.
However, the performance of deep learning model
is prone to deterioration and even collapse due to do-
main shift, i.e., domain transferring from one to an-
other. In particular, the changeable weather and illu-
mination are problematic for the model trained under
the common domain. Therefore, the data in adverse
domain is the desideratum to improve the robustness
of the model while such data like aerial images in low-
illumination, snowy or foggy weather are difficult to
acquire. Therefore, we plan to deploy the current
deep learning-based image synthesis and style trans-
fer methodology to augment the aerial image data
with different weather and illumination conditions to
enhance the model’s ability for domain adaptation.
REFERENCES
Ba, J. L., Kiros, J. R., and Hinton, G. E. (2016). Layer
normalization. arXiv preprint arXiv:1607.06450.
Badrinarayanan, V., Kendall, A., and Cipolla, R. (2017).
Segnet: A deep convolutional encoder-decoder archi-
tecture for image segmentation. IEEE transactions
on Pattern Analysis and Machine Intelligence (PAMI),
39(12):2481–2495.
Chen, L.-C., Papandreou, G., Schroff, F., and Adam,
H. (2017). Rethinking atrous convolution for
semantic image segmentation. arXiv preprint
arXiv:1706.05587.
Chen, L.-C., Zhu, Y., Papandreou, G., Schroff, F., and
Adam, H. (2018). Encoder-decoder with atrous sepa-
rable convolution for semantic image segmentation. In
Proceedings of the European conference on computer
vision (ECCV), pages 801–818.
Chen, Q., Wang, Y., Yang, T., Zhang, X., Cheng, J., and
Sun, J. (2021). You only look one-level feature. In
Proceedings of the IEEE/CVF conference on com-
puter vision and pattern recognition (CVPR), pages
13034–13043.
Dosovitskiy, A., Beyer, L., Kolesnikov, A., Weissenborn,
D., Zhai, X., Unterthiner, T., Dehghani, M., Minderer,
M., Heigold, G., Gelly, S., et al. (2020). An image is
worth 16x16 words: Transformers for image recogni-
tion at scale. arXiv preprint arXiv:2010.11929.
Fu, J., Liu, J., Tian, H., Li, Y., Bao, Y., Fang, Z., and Lu,
H. (2019). Dual attention network for scene segmen-
tation. In Proceedings of the IEEE/CVF conference
on computer vision and pattern recognition (CVPR),
pages 3146–3154.
He, K., Zhang, X., Ren, S., and Sun, J. (2016). Deep resid-
ual learning for image recognition. In Proceedings
of the IEEE/CVF conference on computer vision and
pattern recognition (CVPR), pages 770–778.
Hu, J., Shen, L., and Sun, G. (2018). Squeeze-and-
excitation networks. In Proceedings of the IEEE/CVF
conference on computer vision and pattern recogni-
tion (CVPR), pages 7132–7141.
Jin, Z., Yu, D., Song, L., Yuan, Z., and Yu, L. (2022). You
should look at all objects. In Proceedings of the Euro-
pean conference on computer vision (ECCV).
Kirillov, A., Girshick, R., He, K., and Doll
´
ar, P. (2019).
Panoptic feature pyramid networks. In Proceedings
of the IEEE/CVF conference on computer vision and
pattern recognition (CVPR), pages 6399–6408.
Kirillov, A., Wu, Y., He, K., and Girshick, R. (2020).
Pointrend: Image segmentation as rendering. In Pro-
ceedings of the IEEE/CVF conference on computer
vision and pattern recognition (CVPR), pages 9799–
9808.
Li, X., He, H., Li, X., Li, D., Cheng, G., Shi, J., Weng,
L., Tong, Y., and Lin, Z. (2021). Pointflow: Flowing
semantics through points for aerial image segmenta-
tion. In Proceedings of the IEEE/CVF Conference
on Computer Vision and Pattern Recognition, pages
4217–4226.
Lin, T.-Y., Doll
´
ar, P., Girshick, R., He, K., Hariharan, B.,
and Belongie, S. (2017). Feature pyramid networks
for object detection. In Proceedings of the IEEE/CVF
conference on computer vision and pattern recogni-
tion (CVPR), pages 2117–2125.
Liu, Z., Lin, Y., Cao, Y., Hu, H., Wei, Y., Zhang, Z., Lin,
S., and Guo, B. (2021). Swin transformer: Hierarchi-
cal vision transformer using shifted windows. In Pro-
ceedings of the IEEE/CVF International Conference
on Computer Vision (ICCV), pages 10012–10022.
Long, J., Shelhamer, E., and Darrell, T. (2015). Fully con-
volutional networks for semantic segmentation. In
Proceedings of the IEEE/CVF conference on com-
puter vision and pattern recognition (CVPR), pages
3431–3440.
Ronneberger, O., Fischer, P., and Brox, T. (2015). U-net:
Convolutional networks for biomedical image seg-
ICPRAM 2024 - 13th International Conference on Pattern Recognition Applications and Methods
46