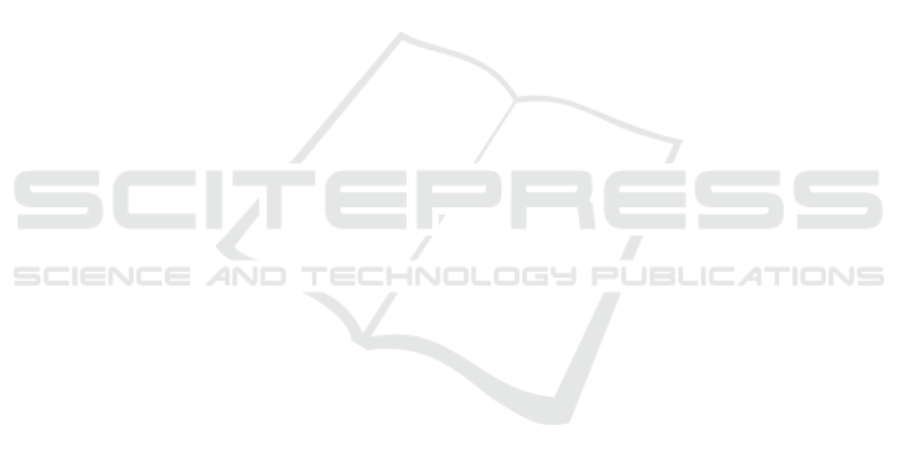
surement conditions. The dataset includes data cap-
tured in different body postures and hand positions.
The analysis of the dataset is carried out from two
perspectives: Aggregate and Subject-wise, consider-
ing three cases: body postures, hand positions, and all
positions in both experiments along with class-wise
analysis on impact of body postures. Among the clas-
sifiers examined, the hybrid CNN Bi-LSTM demon-
strates the best performance, successfully recognizing
Fine-ADL even in diverse measurement conditions.
The most challenging body posture for the classifier is
Folded Knees, while the least challenging is the Stand
posture. Interestingly, both the hand positions consid-
ered yield similar accuracies. Nevertheless, the cur-
rent outcomes highlight the potential of the proposed
framework for real-time Fine-ADL and also demon-
strate the impact of various measurement conditions
on Fine-ADL. In terms of future work, there is po-
tential for further enhancing the model through fine-
tuning to achieve improved results. Additionally, the
impact of the amount of training data, pertaining to a
specific measurement condition, on testing accuracy
needs to be investigated. Furthermore, feature selec-
tion analysis also requires further improvements and
the generalization ability of the model to new subjects
needs to be explored.
REFERENCES
Castellini, C., Fiorilla, A. E., and Sandini, G. (2009). Multi-
subject/daily-life activity emg-based control of me-
chanical hands. Journal of neuroengineering and re-
habilitation, 6(1):1–11.
Chen, M., Dutt, A. S., and Nair, R. (2022). Systematic re-
view of reviews on activities of daily living measures
for children with developmental disabilities. Heliyon,
8(6):e09698.
Criswell, E. (2010). Cram’s introduction to surface elec-
tromyography. Jones & Bartlett Publishers, Burling-
ton.
Dai, S., Wang, Q., and Meng, F. (2021). A telehealth frame-
work for dementia care: an adls patterns recognition
model for patients based on nilm. In 2021 Interna-
tional Joint Conference on Neural Networks (IJCNN),
pages 1–8.
Faria, A. L., Pinho, M. S., and Berm
´
udez i Badia, S. (2020).
A comparison of two personalization and adaptive
cognitive rehabilitation approaches: a randomized
controlled trial with chronic stroke patients. Journal
of NeuroEngineering and Rehabilitation, 17(1):78.
Fauth, E. B., Schaefer, S. Y., Zarit, S. H., Ernsth-Bravell,
M., and Johansson, B. (2017). Associations between
fine motor performance in activities of daily living and
cognitive ability in a nondemented sample of older
adults: Implications for geriatric physical rehabilita-
tion. Journal of Aging and Health, 29(7):1144–1159.
Fougner, A., Scheme, E., Chan, A. D. C., Englehart, K.,
and Stavdahl, Ø (2011). Resolving the limb position
effect in myoelectric pattern recognition. IEEE Trans-
actions on Neural Systems and Rehabilitation Engi-
neering, 19(6):644–651.
He, C., Xiong, C.-H., Chen, Z.-J., Fan, W., Huang, X.-L.,
and Fu, C. (2021). Preliminary assessment of a pos-
tural synergy-based exoskeleton for post-stroke upper
limb rehabilitation. IEEE Transactions on Neural Sys-
tems and Rehabilitation Engineering, 29:1795–1805.
Karnam, N. K., Dubey, S. R., Turlapaty, A. C., and
Gokaraju, B. (2022). Emghandnet: A hybrid cnn and
bi-lstm architecture for hand activity classification us-
ing surface emg signals. Biocybernetics and Biomed-
ical Engineering, 42(1):325–340.
Karnam, N. K., Turlapaty, A. C., Dubey, S. R., and
Gokaraju, B. (2021). Classification of semg signals
of hand gestures based on energy features. Biomedi-
cal Signal Processing and Control, 70:102948.
Katz, S. (1983). Assessing self-maintenance: activities of
daily living, mobility, and instrumental activities of
daily living. J Am Geriatr Soc, 31(12):721–727.
Park, J., Zahabi, M., Kaber, D., Ruiz, J., and Huang, H.
(2020). Evaluation of activities of daily living tes-
beds for assessing prosthetic device usability. In 2020
IEEE International Conference on Human-Machine
Systems (ICHMS), pages 1–4.
Rosenburg, R. and Seidel, H. (1989). Electromyography of
lumbar erector spinae muscles–influence of posture,
interelectrode distance, strength, and fatigue. Eur J
Appl Physiol Occup Physiol, 59(1-2):104–114.
Sandberg, M., Kristensson, J., Midl
¨
ov, P., Fagerstr
¨
om, C.,
and Jakobsson, U. (2012). Prevalence and predictors
of healthcare utilization among older people (60+):
Focusing on adl dependency and risk of depression.
Archives of Gerontology and Geriatrics, 54(3):e349–
e363.
Sapsanis, C., Georgoulas, G., and Tzes, A. (2013). Emg
based classification of basic hand movements based
on time-frequency features. In 21st Mediterranean
Conference on Control and Automation, pages 716–
722.
Song, M. and Kim, J. (2018). An ambulatory gait moni-
toring system with activity classification and gait pa-
rameter calculation based on a single foot inertial sen-
sor. IEEE Transactions on Biomedical Engineering,
65(4):885–893.
Toledo-P
´
erez, D. C., Rodr
´
ıguez-Res
´
endiz, J., G
´
omez-
Loenzo, R. A., and Jauregui-Correa, J. (2019). Sup-
port vector machine-based EMG signal classification
techniques: A review. Applied Sciences, 9(20):4402.
doi: 10.3390/app9204402.
Williams, H., Shehata, A. W., Dawson, M., Scheme, E.,
Hebert, J., and Pilarski, P. (2022). Recurrent convo-
lutional neural networks as an approach to Position-
Aware myoelectric prosthesis control. IEEE Trans
Biomed Eng, 69(7):2243–2255.
Yang, D., Yang, W., Huang, Q., and Liu, H. (2015). Clas-
sification of multiple finger motions during dynamic
upper limb movements. IEEE journal of biomedical
and health informatics, 21(1):134–141.
BIOSIGNALS 2024 - 17th International Conference on Bio-inspired Systems and Signal Processing
598