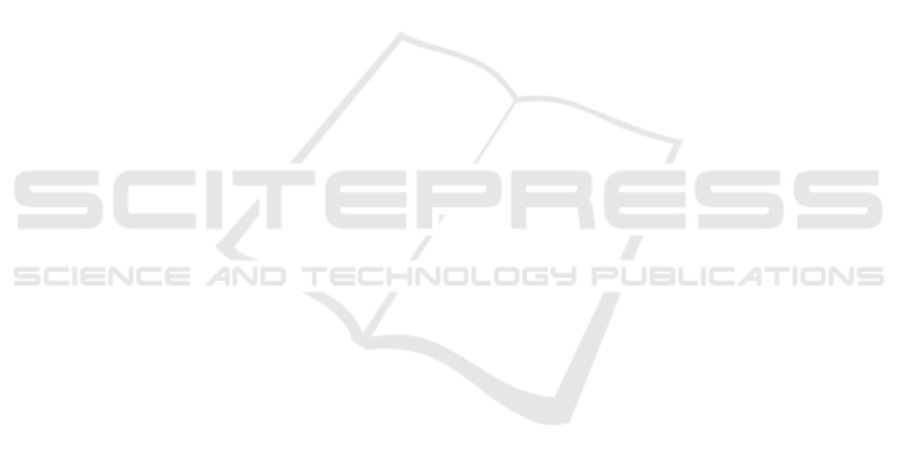
Research Journal, 9(2):147 – 164. Place: Budapest,
Hungary Publisher: Akad
´
emiai Kiad
´
o.
Kitchenham, B. A. (2012). Systematic review in software
engineering: Where we are and where we should be
going. In Proceedings of the 2nd International Work-
shop on Evidential Assessment of Software Technolo-
gies, EAST ’12, page 1–2, New York, NY, USA. As-
sociation for Computing Machinery.
Kotsiantis, S. B., Pierrakeas, C. J., and Pintelas, P. E.
(2003). Preventing student dropout in distance learn-
ing using machine learning techniques. In Palade,
V., Howlett, R. J., and Jain, L., editors, Knowledge-
Based Intelligent Information and Engineering Sys-
tems, pages 267–274, Berlin, Heidelberg. Springer
Berlin Heidelberg.
L
´
opez-Angulo, Y., S
´
aez-Delgado, F., Mella-Norambuena,
J., Bernardo, A. B., and D
´
ıaz-Mujica, A. (2023). Pre-
dictive model of the dropout intention of chilean uni-
versity students. Frontiers in Psychology, 13:893894.
Moseley, L. and Mead, D. (2008). Predicting who will drop
out of nursing courses: A machine learning exercise.
Nurse education today, 28:469–75.
Nagy, M. and Molontay, R. (2023). Interpretable dropout
prediction: Towards xai-based personalized interven-
tion. International Journal of Artificial Intelligence in
Education.
Opazo, D., Moreno, S.,
´
Alvarez Miranda, E., and Pereira,
J. (2021). Analysis of first-year university student
dropout through machine learning models: A compar-
ison between universities. Mathematics, 9:2599.
Osorio, J. and Santacoloma, G. (2023). Predictive model
to identify college students with high dropout rates.
Revista Electr
´
onica de Investigaci
´
on Educativa, 25:1–
10.
Pachas, D. A. G., Garcia-Zanabria, G., Cuadros-Vargas,
A. J., Camara-Chavez, G., Poco, J., and Gomez-Nieto,
E. (2021). A comparative study of who and when
prediction approaches for early identification of uni-
versity students at dropout risk. In 2021 XLVII Latin
American Computing Conference (CLEI), pages 1–10.
Petersen, K., Vakkalanka, S., and Kuzniarz, L. (2015).
Guidelines for conducting systematic mapping stud-
ies in software engineering: An update. Information
and software technology, 64:1–18.
Ramirez, J. A. R., Garcia-Bedoya, O., and Galpin, I. (2022).
Maximizing student retention using supervised mod-
els informed by student counseling data. 3282:225–
239.
Realinho, V., Machado, J., Baptista, L., and Martins, M. V.
(2022). Predicting student dropout and academic suc-
cess. Data, 7(11).
Revathy, M., Kamalakkannan, S., and Kavitha, P. (2022).
Machine learning based prediction of dropout students
from the education university using smote. In 2022
4th International Conference on Smart Systems and
Inventive Technology (ICSSIT), pages 1750–1758.
S Sani, N., Fikri, A., Ali, Z., Zakree, M., and Nadiyah,
K. (2020). Drop-out prediction in higher education
among b40 students. International Journal of Ad-
vanced Computer Science and Applications, 11:550–
559.
Santos, G., Belloze, K., Tarrataca, L., Haddad, D., Bor-
dignon, A., and Brandao, D. (2020). Evolvedtree: An-
alyzing student dropout in universities. In 2020 Inter-
national Conference on Systems, Signals and Image
Processing (IWSSIP), pages 173–178.
Silva, J. and Roman, N. (2021). Predicting dropout in
higher education: a systematic review. In Anais
do XXXII Simp
´
osio Brasileiro de Inform
´
atica na
Educac¸
˜
ao, pages 1107–1117, Porto Alegre, RS,
Brasil. SBC.
Solis, M., Moreira-Mora, T., Gonzalez, R., Fernandez, T.,
and Hernandez, M. (2018). Perspectives to predict
dropout in university students with machine learn-
ing. In 2018 IEEE International Work Conference on
Bioinspired Intelligence (IWOBI), pages 1–6.
Tete, M. F., Sousa, M. d. M., de Santana, T. S., and Silva,
S. F. (2022). Predictive models for higher education
dropout: A systematic literature review. Education
Policy Analysis Archives, 30:(149).
Uliyan, D., Aljaloud, A. S., Alkhalil, A., Al Amer, H. S.,
Mohamed, M. A. E. A., and Alogali, A. F. M. (2021).
Deep learning model to predict students retention us-
ing blstm and crf. IEEE Access, 9:135550–135558.
Zhang, L. and Rangwala, H. (2018). Early identification
of at-risk students using iterative logistic regression.
In 2018 International Conference on Artificial Intelli-
gence in Education.
Zihan, S., Sung, S.-H., Park, D.-M., and Park, B.-K. (2023).
All-year dropout prediction modeling and analysis for
university students. Applied Sciences, 13:1143.
Artificial Intelligence Algorithms to Predict College Students’ Dropout: A Systematic Mapping Study
351